An Effective Transition-based Model for Discontinuous NER
Xiang Dai, Sarvnaz Karimi, Ben Hachey, Cecile Paris
Information Extraction Long Paper
Session 11A: Jul 8
(05:00-06:00 GMT)
Session 12B: Jul 8
(09:00-10:00 GMT)
Abstract:
Unlike widely used Named Entity Recognition (NER) data sets in generic domains, biomedical NER data sets often contain mentions consisting of discontinuous spans. Conventional sequence tagging techniques encode Markov assumptions that are efficient but preclude recovery of these mentions. We propose a simple, effective transition-based model with generic neural encoding for discontinuous NER. Through extensive experiments on three biomedical data sets, we show that our model can effectively recognize discontinuous mentions without sacrificing the accuracy on continuous mentions.
You can open the
pre-recorded video
in a separate window.
NOTE: The SlidesLive video may display a random order of the authors.
The correct author list is shown at the top of this webpage.
Similar Papers
A Unified MRC Framework for Named Entity Recognition
Xiaoya Li, Jingrong Feng, Yuxian Meng, Qinghong Han, Fei Wu, Jiwei Li,
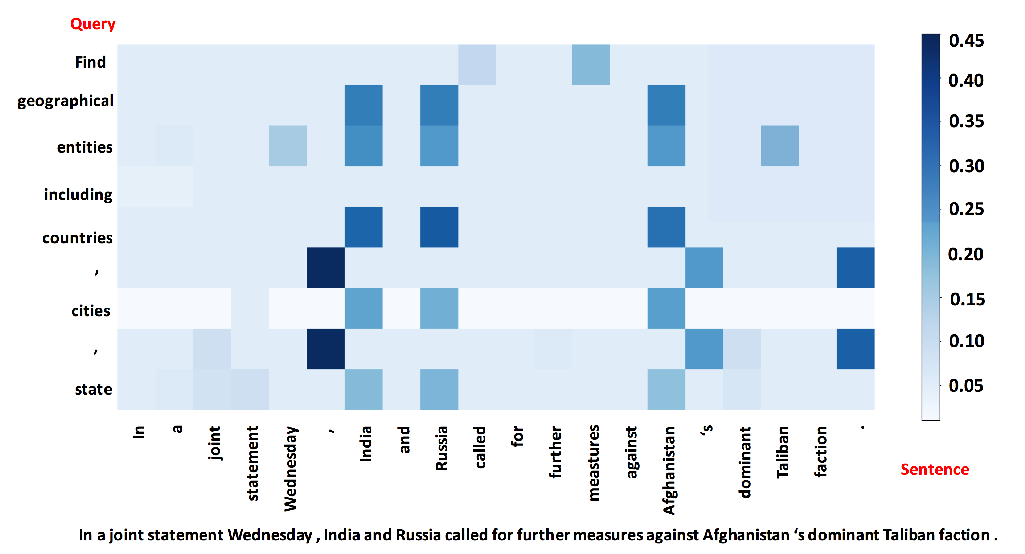
Named Entity Recognition without Labelled Data: A Weak Supervision Approach
Pierre Lison, Jeremy Barnes, Aliaksandr Hubin, Samia Touileb,
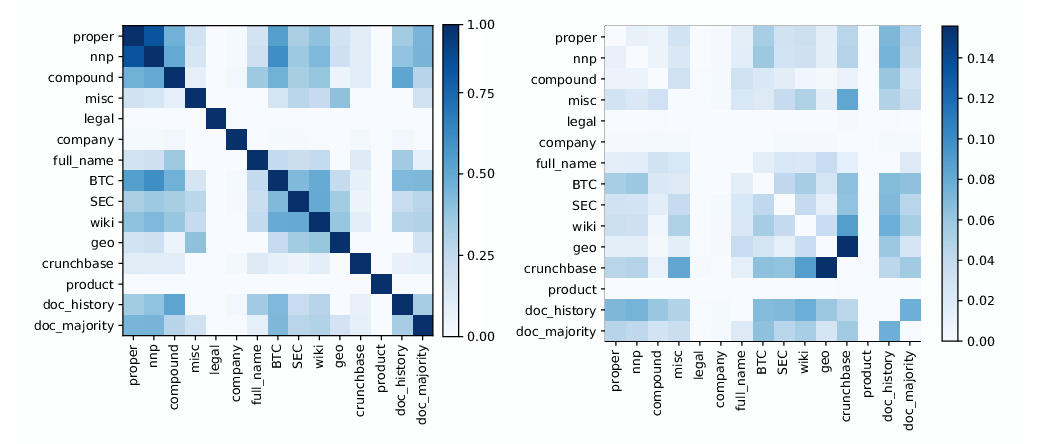
Simplify the Usage of Lexicon in Chinese NER
Ruotian Ma, Minlong Peng, Qi Zhang, Zhongyu Wei, Xuanjing Huang,
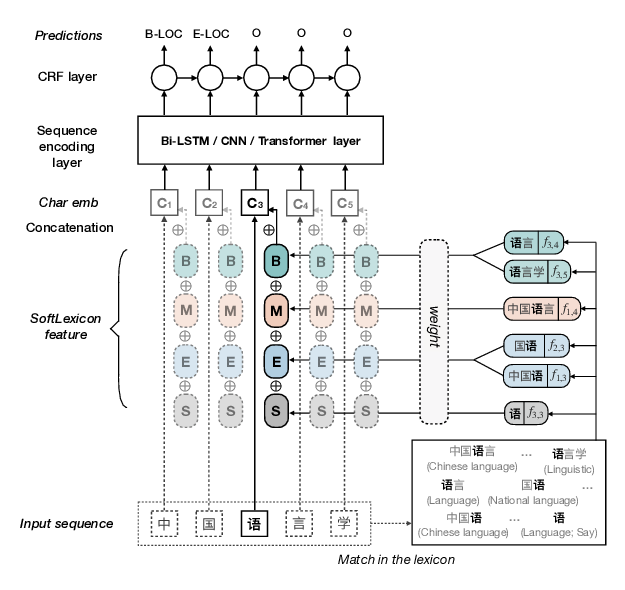
TriggerNER: Learning with Entity Triggers as Explanations for Named Entity Recognition
Bill Yuchen Lin, Dong-Ho Lee, Ming Shen, Ryan Moreno, Xiao Huang, Prashant Shiralkar, Xiang Ren,
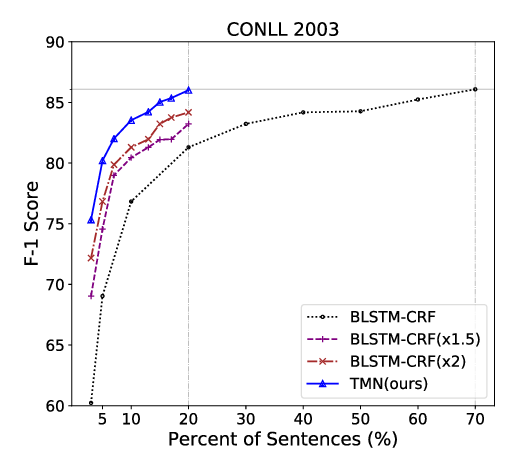