SentiBERT: A Transferable Transformer-Based Architecture for Compositional Sentiment Semantics
Da Yin, Tao Meng, Kai-Wei Chang
Sentiment Analysis, Stylistic Analysis, and Argument Mining Long Paper
Session 6B: Jul 7
(06:00-07:00 GMT)
Session 8A: Jul 7
(12:00-13:00 GMT)
Abstract:
We propose SentiBERT, a variant of BERT that effectively captures compositional sentiment semantics. The model incorporates contextualized representation with binary constituency parse tree to capture semantic composition. Comprehensive experiments demonstrate that SentiBERT achieves competitive performance on phrase-level sentiment classification. We further demonstrate that the sentiment composition learned from the phrase-level annotations on SST can be transferred to other sentiment analysis tasks as well as related tasks, such as emotion classification tasks. Moreover, we conduct ablation studies and design visualization methods to understand SentiBERT. We show that SentiBERT is better than baseline approaches in capturing negation and the contrastive relation and model the compositional sentiment semantics.
You can open the
pre-recorded video
in a separate window.
NOTE: The SlidesLive video may display a random order of the authors.
The correct author list is shown at the top of this webpage.
Similar Papers
SKEP: Sentiment Knowledge Enhanced Pre-training for Sentiment Analysis
Hao Tian, Can Gao, Xinyan Xiao, Hao Liu, Bolei He, Hua Wu, Haifeng Wang, Feng Wu,
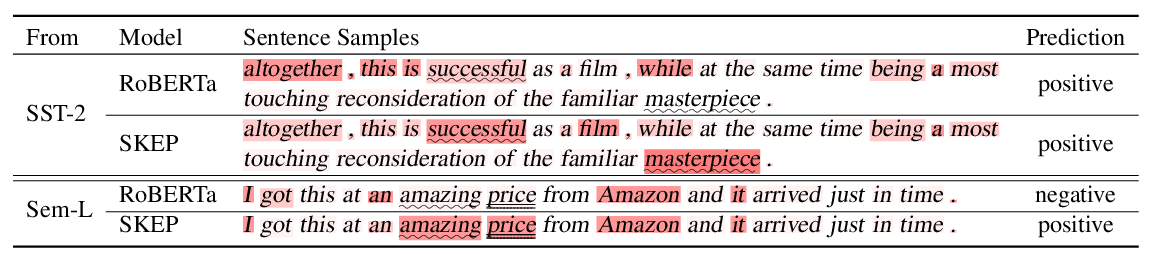
MobileBERT: a Compact Task-Agnostic BERT for Resource-Limited Devices
Zhiqing Sun, Hongkun Yu, Xiaodan Song, Renjie Liu, Yiming Yang, Denny Zhou,
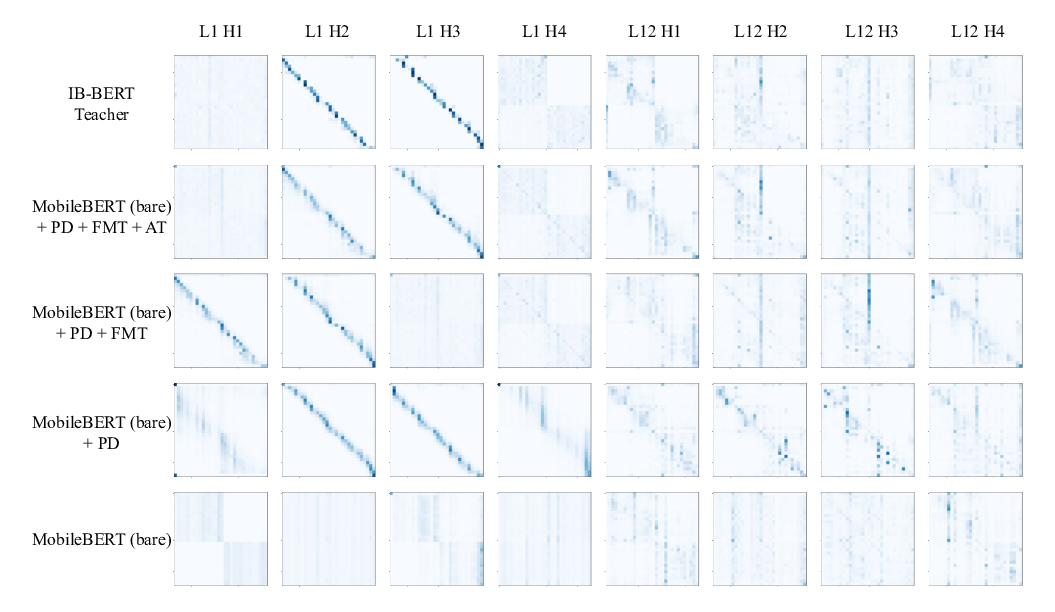
DeeBERT: Dynamic Early Exiting for Accelerating BERT Inference
Ji Xin, Raphael Tang, Jaejun Lee, Yaoliang Yu, Jimmy Lin,
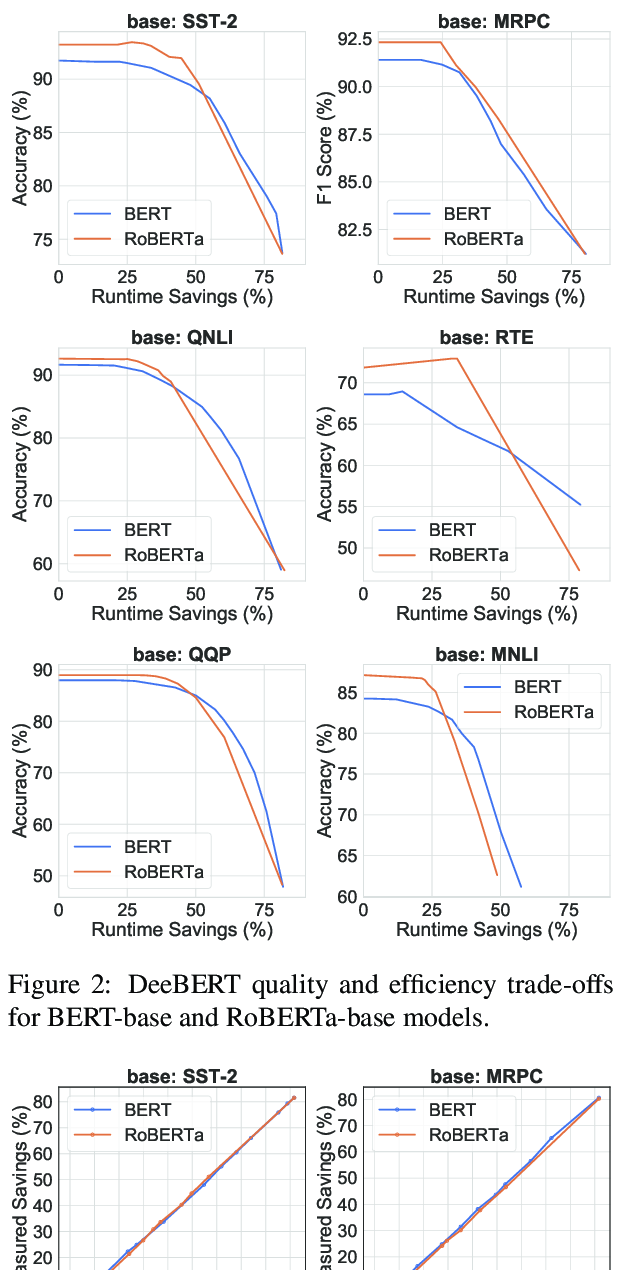
Aspect Sentiment Classification with Document-level Sentiment Preference Modeling
Xiao Chen, Changlong Sun, Jingjing Wang, Shoushan Li, Luo Si, Min Zhang, Guodong Zhou,
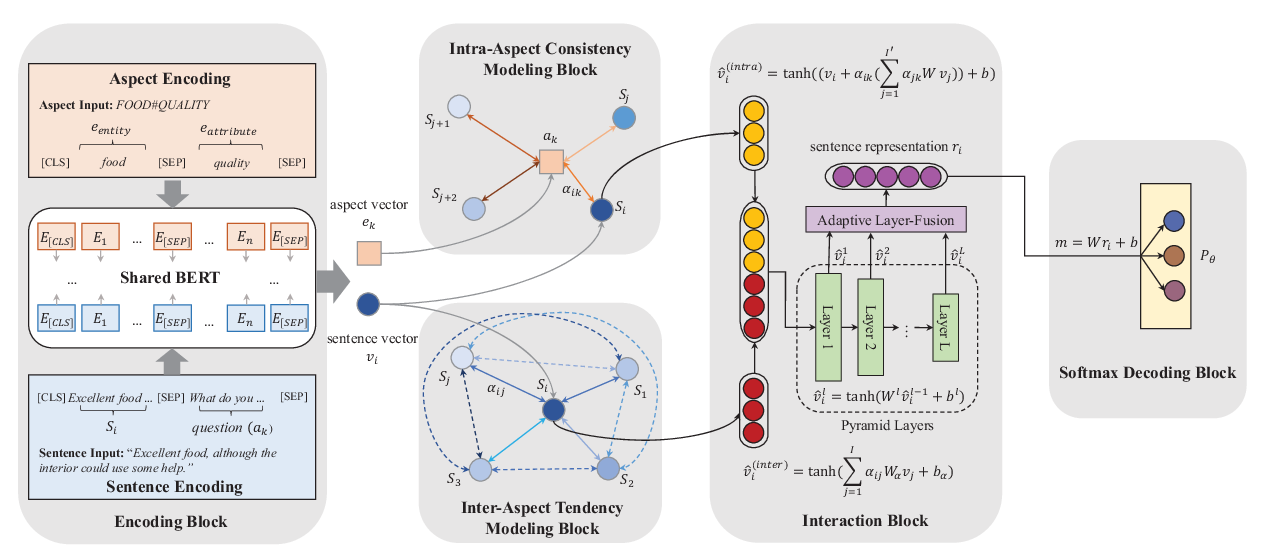