SKEP: Sentiment Knowledge Enhanced Pre-training for Sentiment Analysis
Hao Tian, Can Gao, Xinyan Xiao, Hao Liu, Bolei He, Hua Wu, Haifeng Wang, Feng Wu
Sentiment Analysis, Stylistic Analysis, and Argument Mining Long Paper
Session 7A: Jul 7
(08:00-09:00 GMT)
Session 8B: Jul 7
(13:00-14:00 GMT)
Abstract:
Recently, sentiment analysis has seen remarkable advance with the help of pre-training approaches. However, sentiment knowledge, such as sentiment words and aspect-sentiment pairs, is ignored in the process of pre-training, despite the fact that they are widely used in traditional sentiment analysis approaches. In this paper, we introduce Sentiment Knowledge Enhanced Pre-training (SKEP) in order to learn a unified sentiment representation for multiple sentiment analysis tasks. With the help of automatically-mined knowledge, SKEP conducts sentiment masking and constructs three sentiment knowledge prediction objectives, so as to embed sentiment information at the word, polarity and aspect level into pre-trained sentiment representation. In particular, the prediction of aspect-sentiment pairs is converted into multi-label classification, aiming to capture the dependency between words in a pair. Experiments on three kinds of sentiment tasks show that SKEP significantly outperforms strong pre-training baseline, and achieves new state-of-the-art results on most of the test datasets. We release our code at https://github.com/baidu/Senta.
You can open the
pre-recorded video
in a separate window.
NOTE: The SlidesLive video may display a random order of the authors.
The correct author list is shown at the top of this webpage.
Similar Papers
CH-SIMS: A Chinese Multimodal Sentiment Analysis Dataset with Fine-grained Annotation of Modality
Wenmeng Yu, Hua Xu, Fanyang Meng, Yilin Zhu, Yixiao Ma, Jiele Wu, Jiyun Zou, Kaicheng Yang,
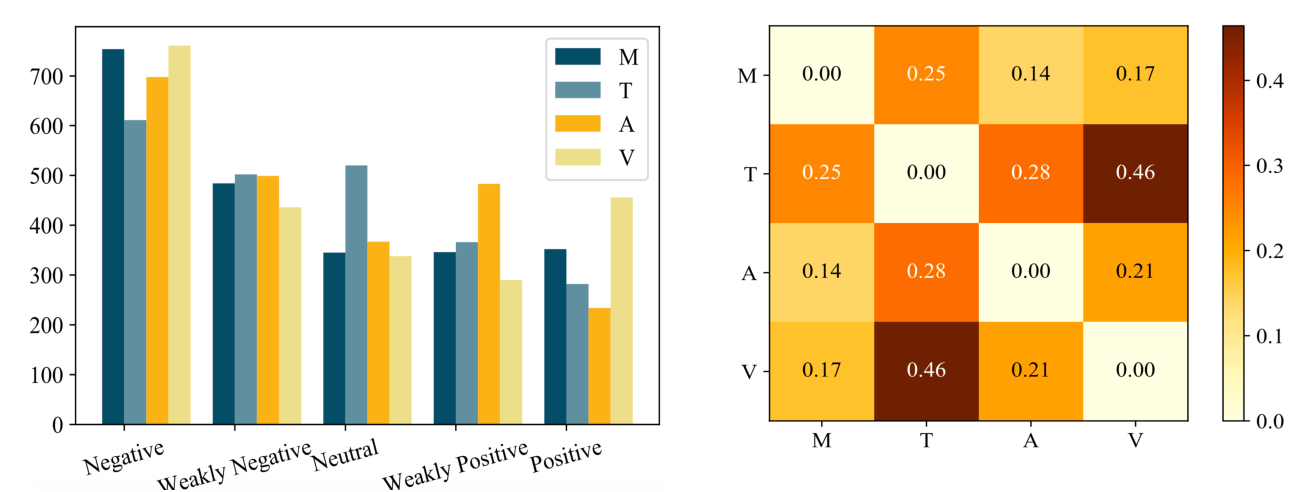
Learning Spoken Language Representations with Neural Lattice Language Modeling
Chao-Wei Huang, Yun-Nung Chen,
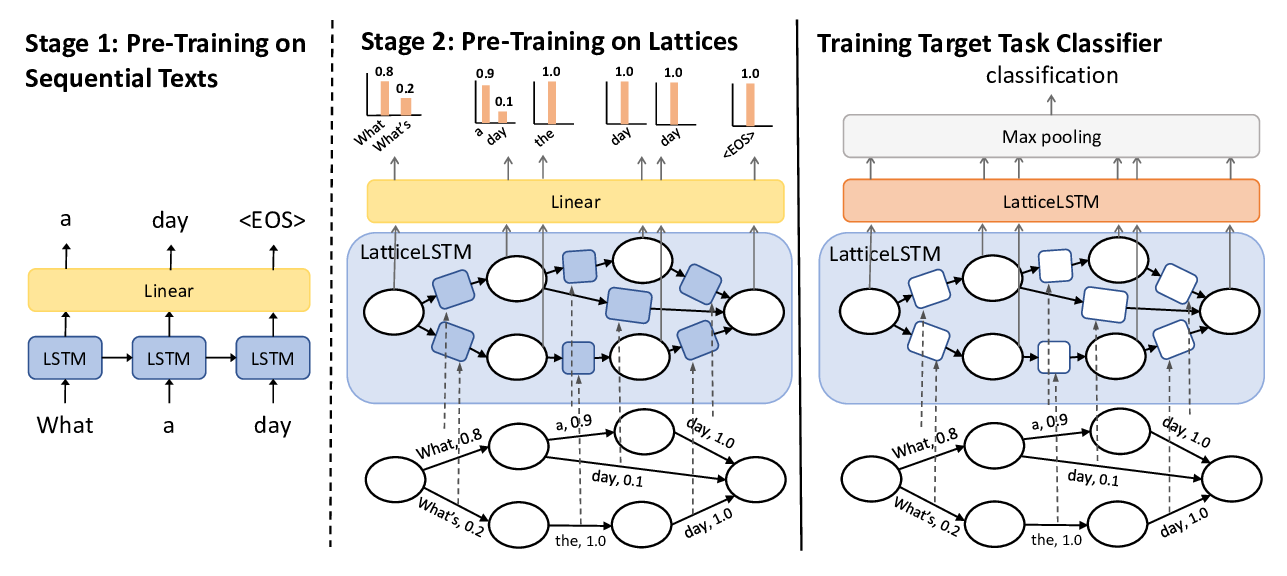
SentiBERT: A Transferable Transformer-Based Architecture for Compositional Sentiment Semantics
Da Yin, Tao Meng, Kai-Wei Chang,
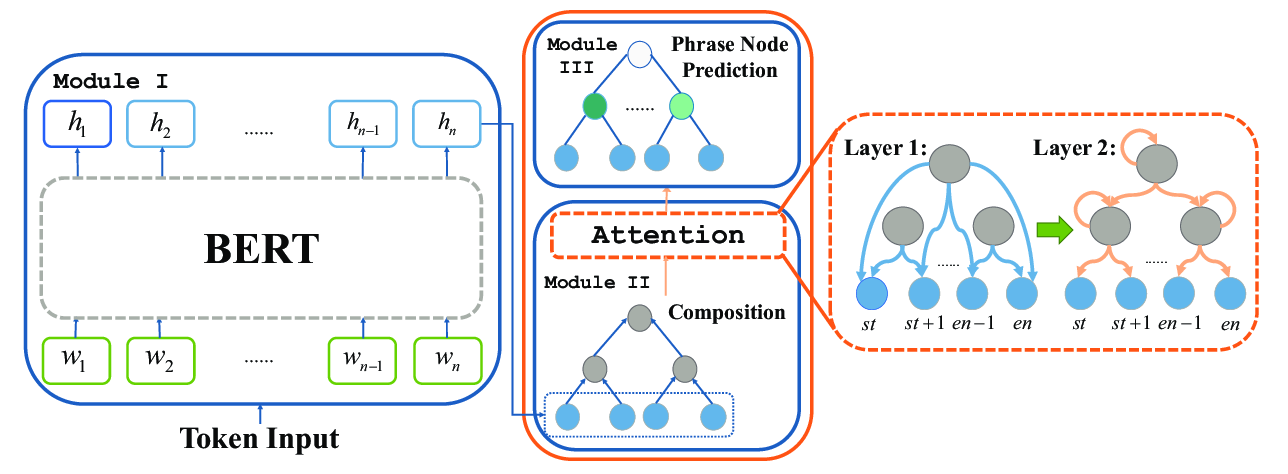
Aspect Sentiment Classification with Document-level Sentiment Preference Modeling
Xiao Chen, Changlong Sun, Jingjing Wang, Shoushan Li, Luo Si, Min Zhang, Guodong Zhou,
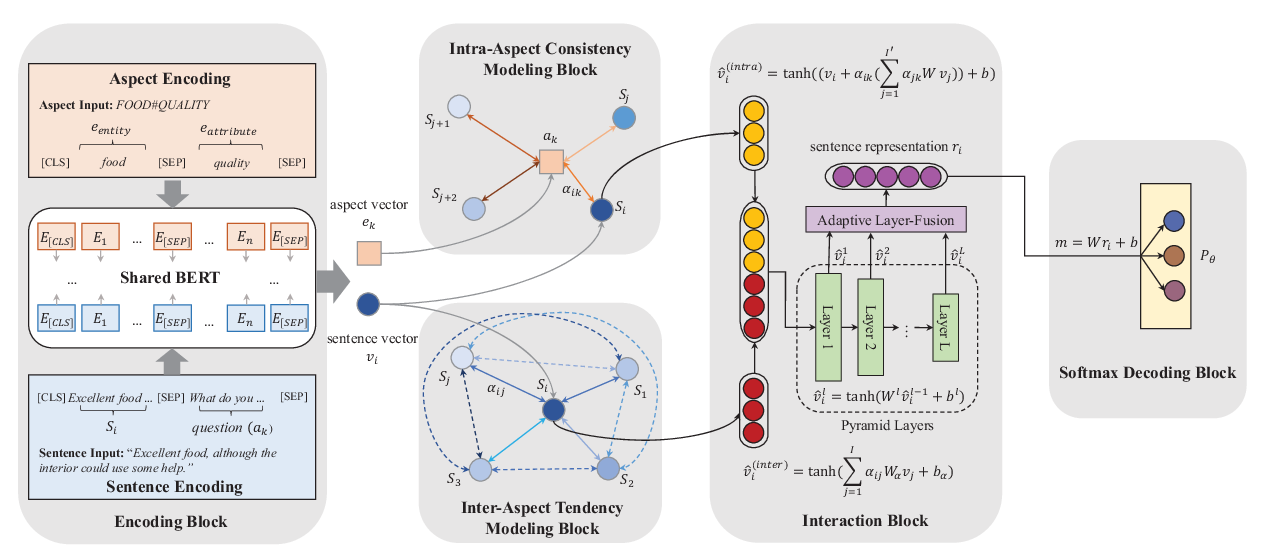