Jointly Masked Sequence-to-Sequence Model for Non-Autoregressive Neural Machine Translation
Junliang Guo, Linli Xu, Enhong Chen
Machine Translation Long Paper
Session 1A: Jul 6
(05:00-06:00 GMT)
Session 3A: Jul 6
(12:00-13:00 GMT)
Abstract:
The masked language model has received remarkable attention due to its effectiveness on various natural language processing tasks. However, few works have adopted this technique in the sequence-to-sequence models. In this work, we introduce a jointly masked sequence-to-sequence model and explore its application on non-autoregressive neural machine translation~(NAT). Specifically, we first empirically study the functionalities of the encoder and the decoder in NAT models, and find that the encoder takes a more important role than the decoder regarding the translation quality. Therefore, we propose to train the encoder more rigorously by masking the encoder input while training. As for the decoder, we propose to train it based on the consecutive masking of the decoder input with an n-gram loss function to alleviate the problem of translating duplicate words. The two types of masks are applied to the model jointly at the training stage. We conduct experiments on five benchmark machine translation tasks, and our model can achieve 27.69/32.24 BLEU scores on WMT14 English-German/German-English tasks with 5+ times speed up compared with an autoregressive model.
You can open the
pre-recorded video
in a separate window.
NOTE: The SlidesLive video may display a random order of the authors.
The correct author list is shown at the top of this webpage.
Similar Papers
Lipschitz Constrained Parameter Initialization for Deep Transformers
Hongfei Xu, Qiuhui Liu, Josef van Genabith, Deyi Xiong, Jingyi Zhang,
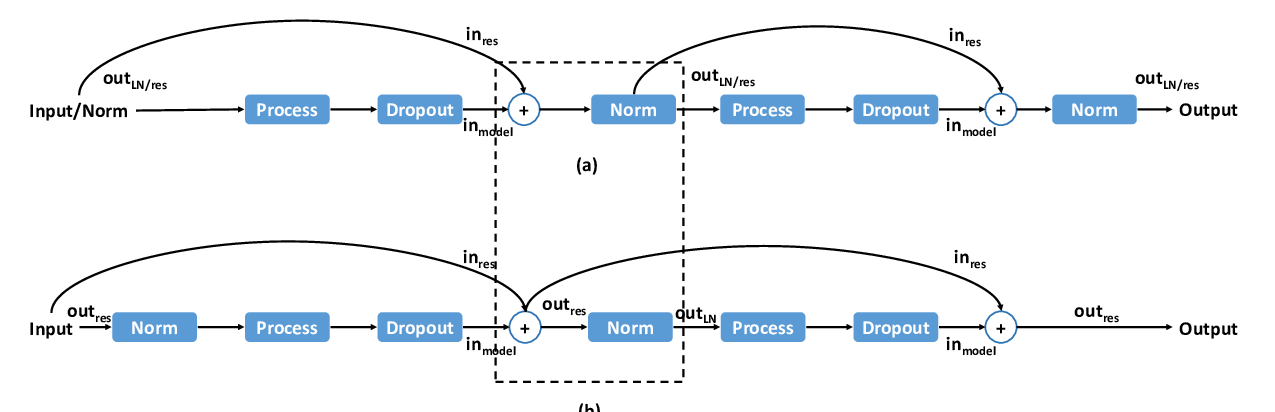
Leveraging Pre-trained Checkpoints for Sequence Generation Tasks
Sascha Rothe, Shashi Narayan and Aliaksei Severyn,
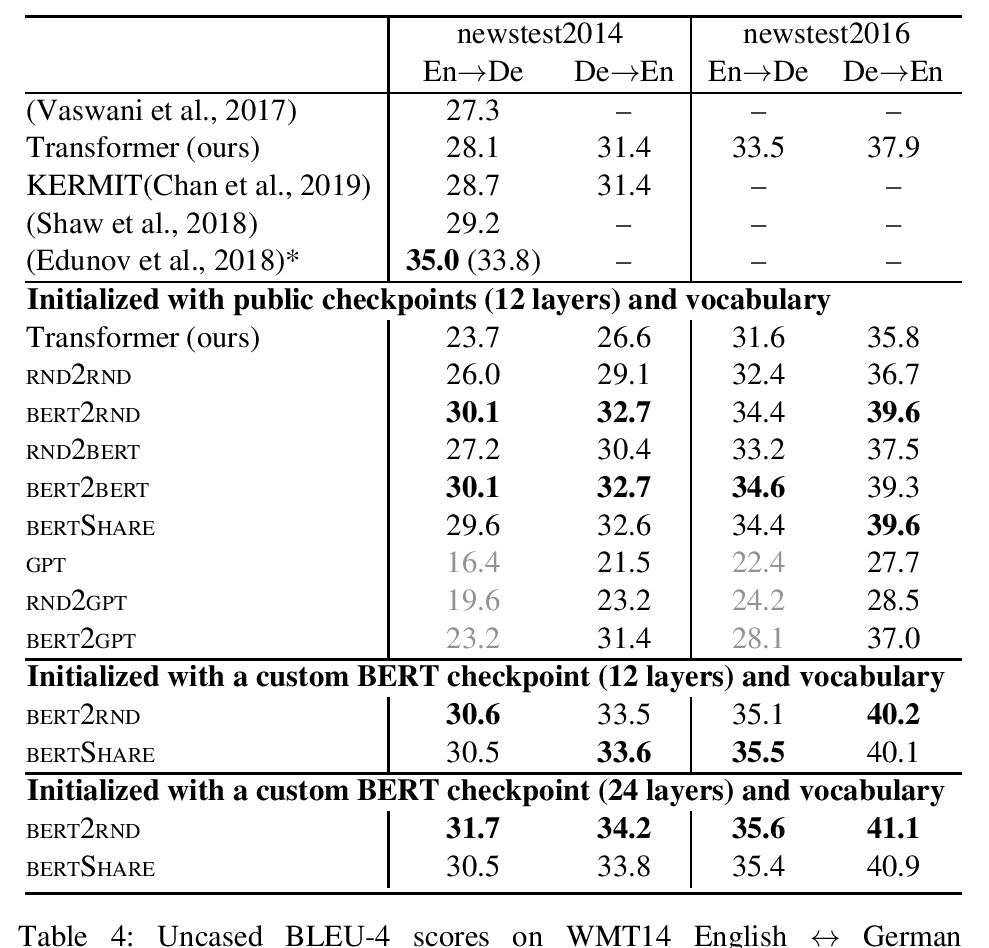
DRTS Parsing with Structure-Aware Encoding and Decoding
Qiankun Fu, Yue Zhang, Jiangming Liu, Meishan Zhang,
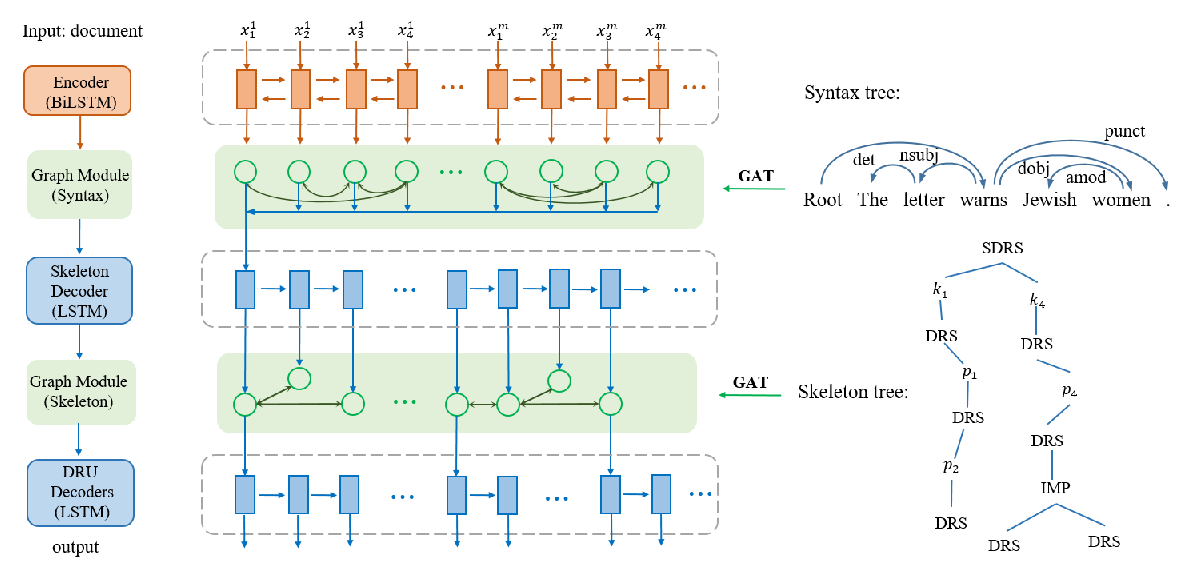