Leveraging Pre-trained Checkpoints for Sequence Generation Tasks
Sascha Rothe, Shashi Narayan and Aliaksei Severyn
Generation TACL Paper
Session 3B: Jul 6
(13:00-14:00 GMT)
Session 4B: Jul 6
(18:00-19:00 GMT)
Abstract:
Unsupervised pre-training of large neural models has recently revolutionized Natural Language Processing. By warm-starting from the publicly released checkpoints, NLP practitioners have pushed the state-of-the-art on multiple benchmarks while saving significant amounts of compute time. So far the focus has been mainly on the Natural Language Understanding tasks. In this paper, we demonstrate the efficacy of pre-trained checkpoints for Sequence Generation. We developed a Transformer-based sequence-to-sequence model that is compatible with publicly available pre-trained BERT, GPT-2 and RoBERTa checkpoints and conducted an extensive empirical study on the utility of initializing our model, both encoder and decoder, with these checkpoints. Our models result in new state-of-the-art results on Machine Translation, Text Summarization, Sentence Splitting, and Sentence Fusion.
You can open the
pre-recorded video
in a separate window.
NOTE: The SlidesLive video may display a random order of the authors.
The correct author list is shown at the top of this webpage.
Similar Papers
Span Selection Pre-training for Question Answering
Michael Glass, Alfio Gliozzo, Rishav Chakravarti, Anthony Ferritto, Lin Pan, G P Shrivatsa Bhargav, Dinesh Garg, Avi Sil,
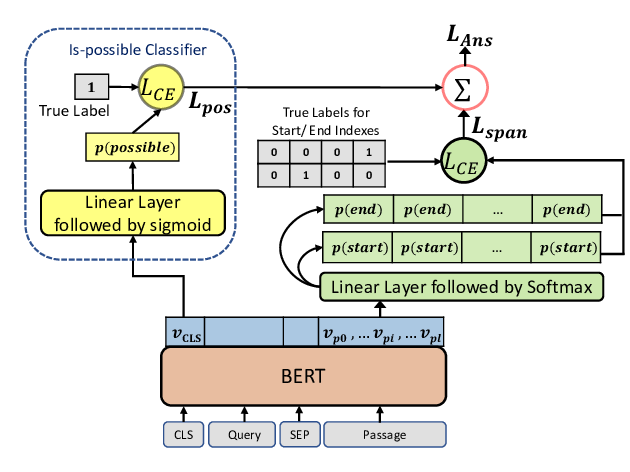
SMART: Robust and Efficient Fine-Tuning for Pre-trained Natural Language Models through Principled Regularized Optimization
Haoming Jiang, Pengcheng He, Weizhu Chen, Xiaodong Liu, Jianfeng Gao, Tuo Zhao,
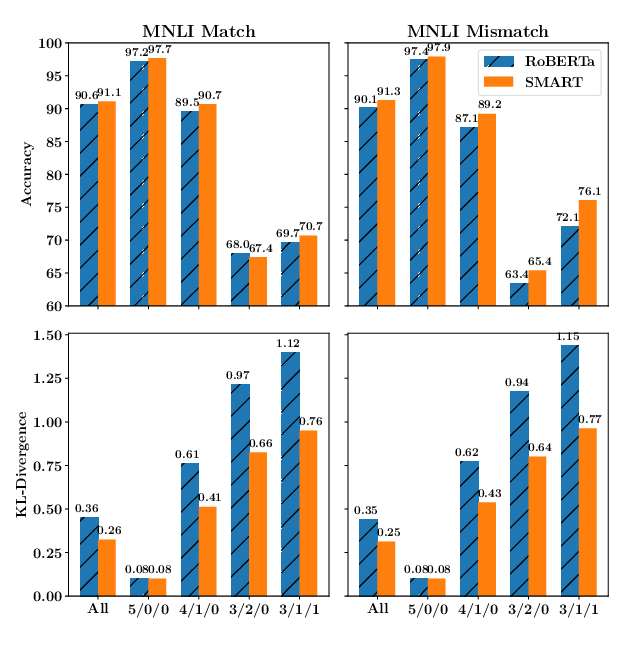