Hard-Coded Gaussian Attention for Neural Machine Translation
Weiqiu You, Simeng Sun, Mohit Iyyer
Machine Translation Long Paper
Session 13B: Jul 8
(13:00-14:00 GMT)
Session 15A: Jul 8
(20:00-21:00 GMT)
Abstract:
Recent work has questioned the importance of the Transformer's multi-headed attention for achieving high translation quality. We push further in this direction by developing a ``hard-coded'' attention variant without any learned parameters. Surprisingly, replacing all learned self-attention heads in the encoder and decoder with fixed, input-agnostic Gaussian distributions minimally impacts BLEU scores across four different language pairs. However, additionally, hard-coding cross attention (which connects the decoder to the encoder) significantly lowers BLEU, suggesting that it is more important than self-attention. Much of this BLEU drop can be recovered by adding just a single learned cross attention head to an otherwise hard-coded Transformer. Taken as a whole, our results offer insight into which components of the Transformer are actually important, which we hope will guide future work into the development of simpler and more efficient attention-based models.
You can open the
pre-recorded video
in a separate window.
NOTE: The SlidesLive video may display a random order of the authors.
The correct author list is shown at the top of this webpage.
Similar Papers
Towards Transparent and Explainable Attention Models
Akash Kumar Mohankumar, Preksha Nema, Sharan Narasimhan, Mitesh M. Khapra, Balaji Vasan Srinivasan, Balaraman Ravindran,
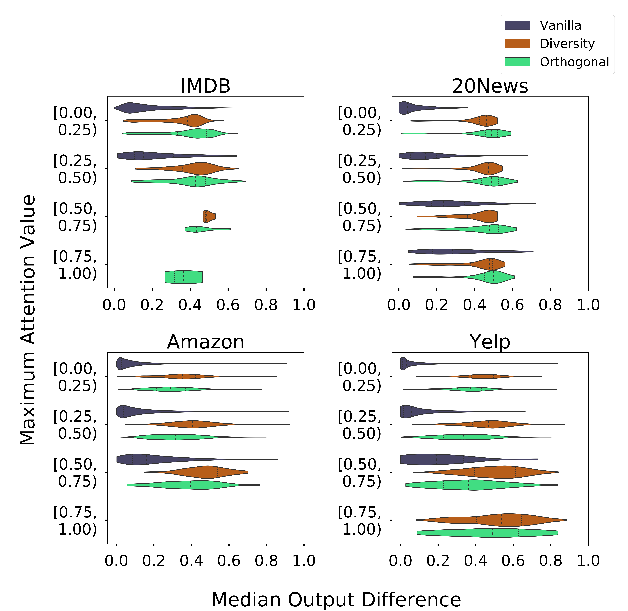
Self-Attention is Not Only a Weight: Analyzing BERT with Vector Norms
Goro Kobayashi, Tatsuki Kuribayashi, Sho Yokoi, Kentaro Inui,
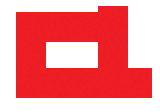