BERTRAM: Improved Word Embeddings Have Big Impact on Contextualized Model Performance
Timo Schick, Hinrich Schütze
Semantics: Lexical Long Paper
Session 7A: Jul 7
(08:00-09:00 GMT)
Session 8A: Jul 7
(12:00-13:00 GMT)
Abstract:
Pretraining deep language models has led to large performance gains in NLP. Despite this success, Schick and Schütze (2020) recently showed that these models struggle to understand rare words. For static word embeddings, this problem has been addressed by separately learning representations for rare words. In this work, we transfer this idea to pretrained language models: We introduce BERTRAM, a powerful architecture based on BERT that is capable of inferring high-quality embeddings for rare words that are suitable as input representations for deep language models. This is achieved by enabling the surface form and contexts of a word to interact with each other in a deep architecture. Integrating BERTRAM into BERT leads to large performance increases due to improved representations of rare and medium frequency words on both a rare word probing task and three downstream tasks.
You can open the
pre-recorded video
in a separate window.
NOTE: The SlidesLive video may display a random order of the authors.
The correct author list is shown at the top of this webpage.
Similar Papers
Interpreting Pretrained Contextualized Representations via Reductions to Static Embeddings
Rishi Bommasani, Kelly Davis, Claire Cardie,
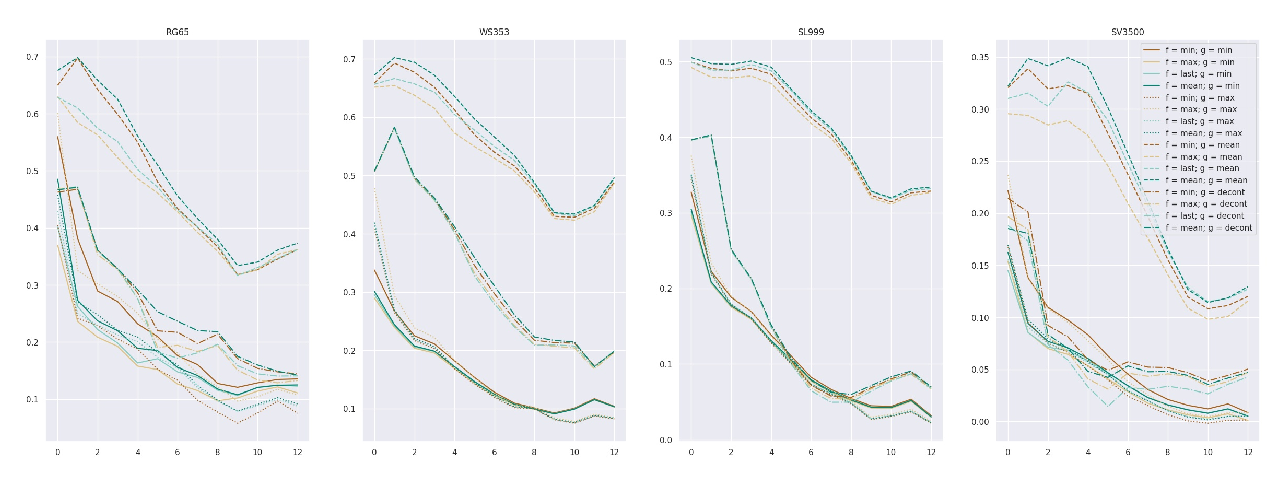
Distilling Knowledge Learned in BERT for Text Generation
Yen-Chun Chen, Zhe Gan, Yu Cheng, Jingzhou Liu, Jingjing Liu,
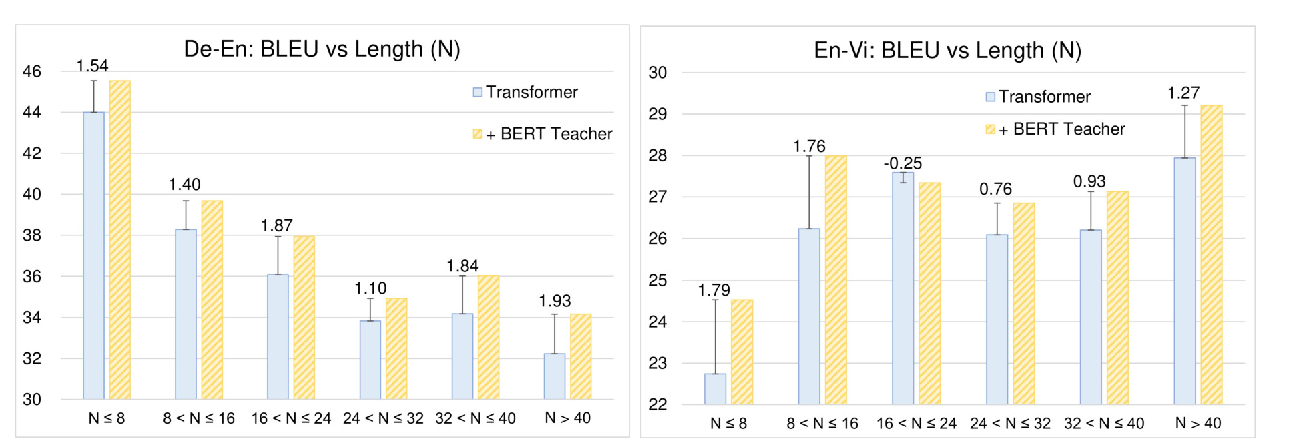
Fast and Accurate Deep Bidirectional Language Representations for Unsupervised Learning
Joongbo Shin, Yoonhyung Lee, Seunghyun Yoon, Kyomin Jung,
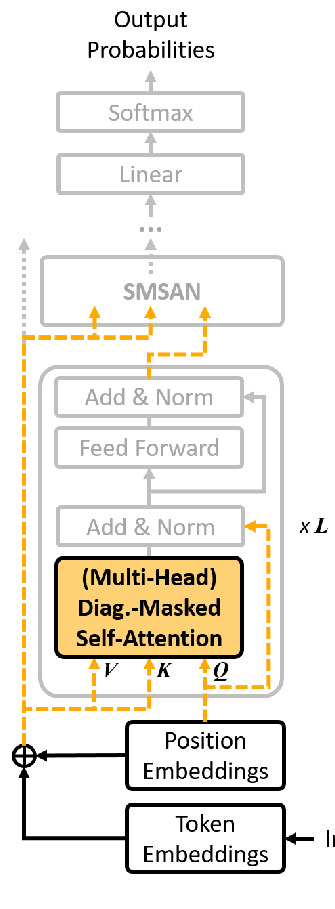
MobileBERT: a Compact Task-Agnostic BERT for Resource-Limited Devices
Zhiqing Sun, Hongkun Yu, Xiaodan Song, Renjie Liu, Yiming Yang, Denny Zhou,
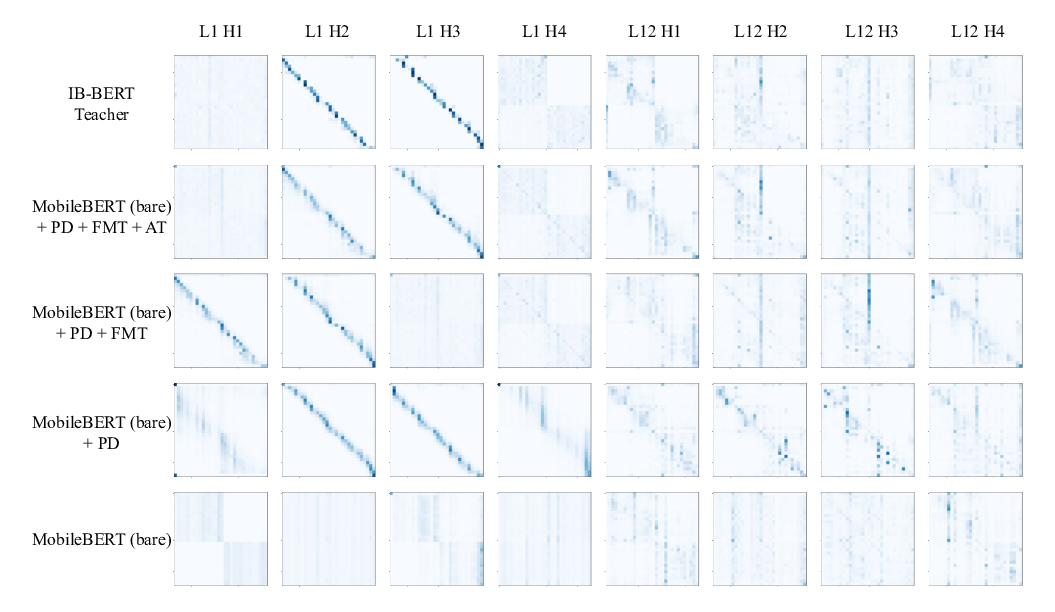