Learning Spoken Language Representations with Neural Lattice Language Modeling
Chao-Wei Huang, Yun-Nung Chen
Speech and Multimodality Short Paper
Session 6B: Jul 7
(06:00-07:00 GMT)
Session 8B: Jul 7
(13:00-14:00 GMT)
Abstract:
Pre-trained language models have achieved huge improvement on many NLP tasks. However, these methods are usually designed for written text, so they do not consider the properties of spoken language. Therefore, this paper aims at generalizing the idea of language model pre-training to lattices generated by recognition systems. We propose a framework that trains neural lattice language models to provide contextualized representations for spoken language understanding tasks. The proposed two-stage pre-training approach reduces the demands of speech data and has better efficiency. Experiments on intent detection and dialogue act recognition datasets demonstrate that our proposed method consistently outperforms strong baselines when evaluated on spoken inputs. The code is available at https://github.com/MiuLab/Lattice-ELMo.
You can open the
pre-recorded video
in a separate window.
NOTE: The SlidesLive video may display a random order of the authors.
The correct author list is shown at the top of this webpage.
Similar Papers
ESPnet-ST: All-in-One Speech Translation Toolkit
Hirofumi Inaguma, Shun Kiyono, Kevin Duh, Shigeki Karita, Nelson Yalta, Tomoki Hayashi, Shinji Watanabe,
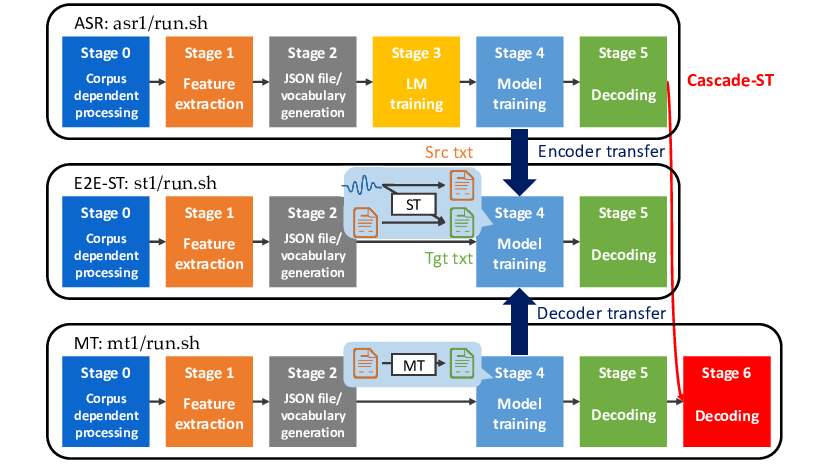
SKEP: Sentiment Knowledge Enhanced Pre-training for Sentiment Analysis
Hao Tian, Can Gao, Xinyan Xiao, Hao Liu, Bolei He, Hua Wu, Haifeng Wang, Feng Wu,
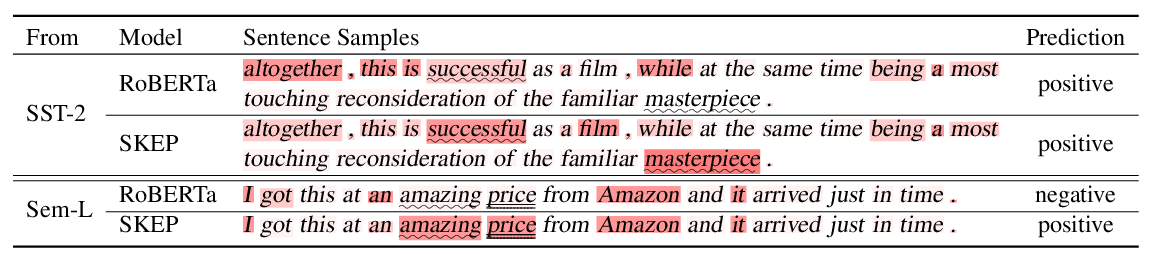
Improving Event Detection via Open-domain Trigger Knowledge
Meihan Tong, Bin Xu, Shuai Wang, Yixin Cao, Lei Hou, Juanzi Li, Jun Xie,
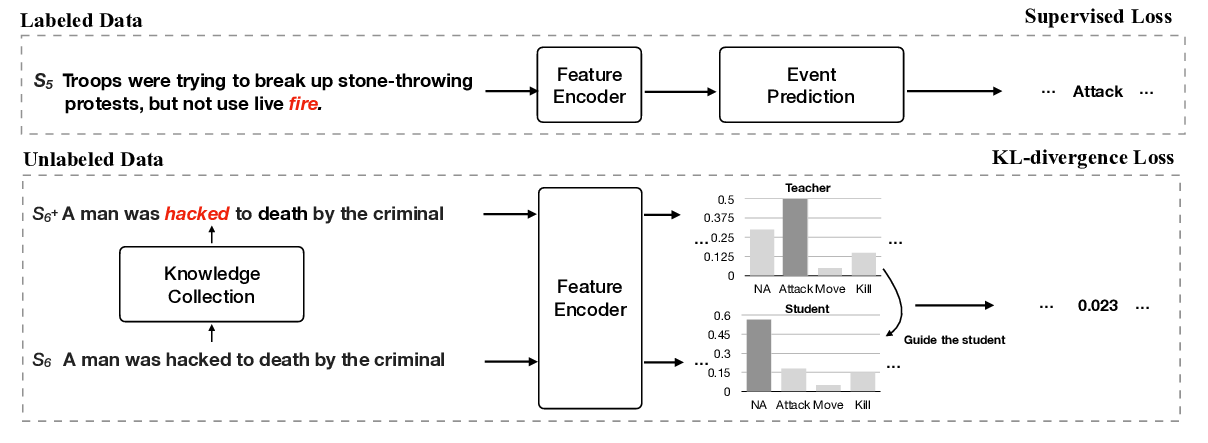
Encoder-Decoder Models Can Benefit from Pre-trained Masked Language Models in Grammatical Error Correction
Masahiro Kaneko, Masato Mita, Shun Kiyono, Jun Suzuki, Kentaro Inui,
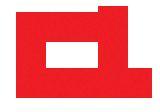