Empowering Active Learning to Jointly Optimize System and User Demands
Ji-Ung Lee, Christian M. Meyer, Iryna Gurevych
NLP Applications Long Paper
Session 7B: Jul 7
(09:00-10:00 GMT / 09:00-10:00 GMT)
Session 8B: Jul 7
(13:00-14:00 GMT / 13:00-14:00 GMT)
Abstract:
Existing approaches to active learning maximize the system performance by sampling unlabeled instances for annotation that yield the most efficient training. However, when active learning is integrated with an end-user application, this can lead to frustration for participating users, as they spend time labeling instances that they would not otherwise be interested in reading. In this paper, we propose a new active learning approach that jointly optimizes the seemingly counteracting objectives of the active learning system (training efficiently) and the user (receiving useful instances). We study our approach in an educational application, which particularly benefits from this technique as the system needs to rapidly learn to predict the appropriateness of an exercise to a particular user, while the users should receive only exercises that match their skills. We evaluate multiple learning strategies and user types with data from real users and find that our joint approach better satisfies both objectives when alternative methods lead to many unsuitable exercises for end users.
You can open the
pre-recorded video
in a separate window.
NOTE: The SlidesLive video may display a random order of the authors.
The correct author list is shown at the top of this webpage.
Similar Papers
Dynamic Online Conversation Recommendation
Xingshan Zeng, Jing Li, Lu Wang, Zhiming Mao, Kam-Fai Wong,
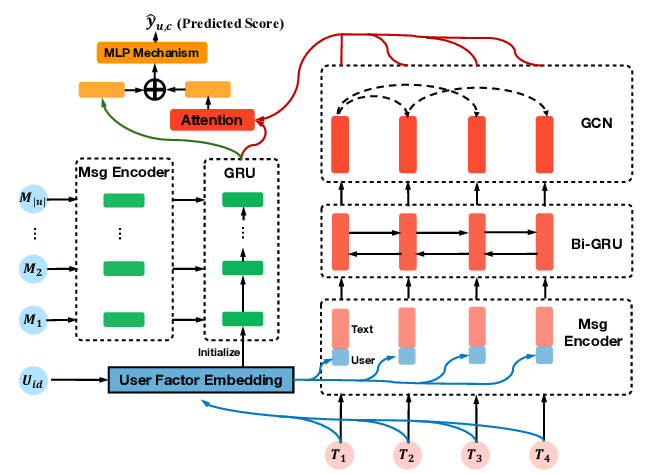
Multi-Agent Task-Oriented Dialog Policy Learning with Role-Aware Reward Decomposition
Ryuichi Takanobu, Runze Liang, Minlie Huang,
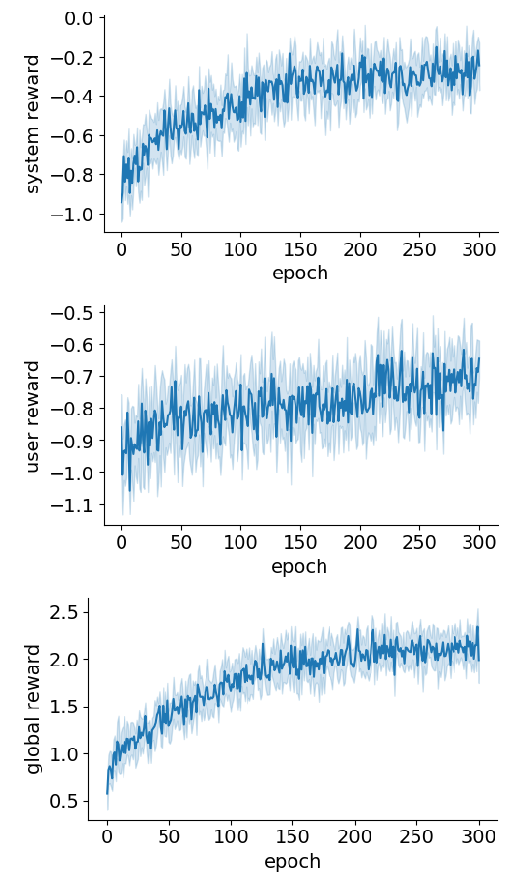
Dynamic Sampling Strategies for Multi-Task Reading Comprehension
Ananth Gottumukkala, Dheeru Dua, Sameer Singh, Matt Gardner,
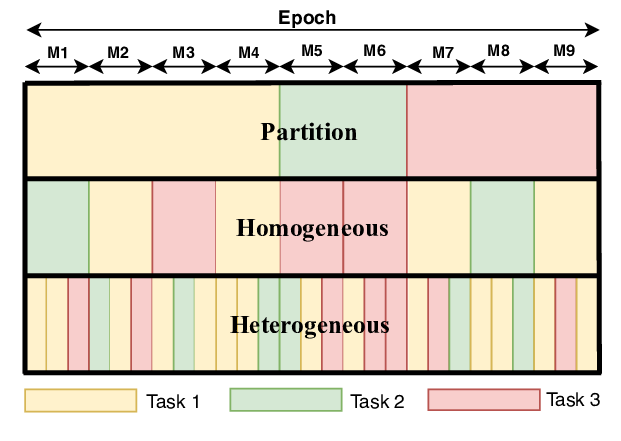