Towards Transparent and Explainable Attention Models
Akash Kumar Mohankumar, Preksha Nema, Sharan Narasimhan, Mitesh M. Khapra, Balaji Vasan Srinivasan, Balaraman Ravindran
Interpretability and Analysis of Models for NLP Long Paper
Session 7B: Jul 7
(09:00-10:00 GMT)
Session 8A: Jul 7
(12:00-13:00 GMT)
Abstract:
Recent studies on interpretability of attention distributions have led to notions of faithful and plausible explanations for a model's predictions. Attention distributions can be considered a faithful explanation if a higher attention weight implies a greater impact on the model's prediction. They can be considered a plausible explanation if they provide a human-understandable justification for the model's predictions. In this work, we first explain why current attention mechanisms in LSTM based encoders can neither provide a faithful nor a plausible explanation of the model's predictions. We observe that in LSTM based encoders the hidden representations at different time-steps are very similar to each other (high conicity) and attention weights in these situations do not carry much meaning because even a random permutation of the attention weights does not affect the model's predictions. Based on experiments on a wide variety of tasks and datasets, we observe attention distributions often attribute the model's predictions to unimportant words such as punctuation and fail to offer a plausible explanation for the predictions. To make attention mechanisms more faithful and plausible, we propose a modified LSTM cell with a diversity-driven training objective that ensures that the hidden representations learned at different time steps are diverse. We show that the resulting attention distributions offer more transparency as they (i) provide a more precise importance ranking of the hidden states (ii) are better indicative of words important for the model's predictions (iii) correlate better with gradient-based attribution methods. Human evaluations indicate that the attention distributions learned by our model offer a plausible explanation of the model's predictions. Our code has been made publicly available at https://github.com/akashkm99/Interpretable-Attention
You can open the
pre-recorded video
in a separate window.
NOTE: The SlidesLive video may display a random order of the authors.
The correct author list is shown at the top of this webpage.
Similar Papers
Learning to Deceive with Attention-Based Explanations
Danish Pruthi, Mansi Gupta, Bhuwan Dhingra, Graham Neubig, Zachary C. Lipton,
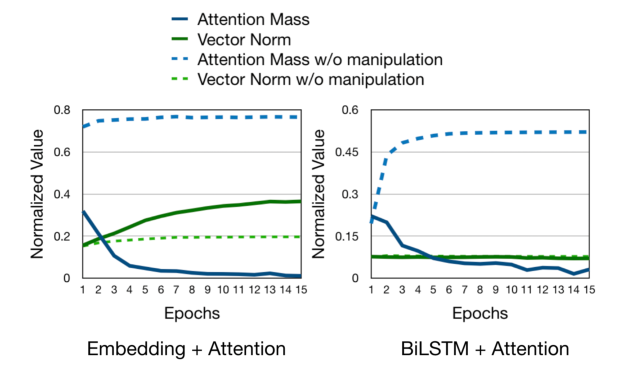
Human Attention Maps for Text Classification: Do Humans and Neural Networks Focus on the Same Words?
Cansu Sen, Thomas Hartvigsen, Biao Yin, Xiangnan Kong, Elke Rundensteiner,
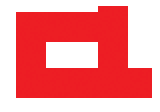