Improving Multi-hop Question Answering over Knowledge Graphs using Knowledge Base Embeddings
Apoorv Saxena, Aditay Tripathi, Partha Talukdar
Question Answering Long Paper
Session 8A: Jul 7
(12:00-13:00 GMT)
Session 9B: Jul 7
(18:00-19:00 GMT)
Abstract:
Knowledge Graphs (KG) are multi-relational graphs consisting of entities as nodes and relations among them as typed edges. Goal of the Question Answering over KG (KGQA) task is to answer natural language queries posed over the KG. Multi-hop KGQA requires reasoning over multiple edges of the KG to arrive at the right answer. KGs are often incomplete with many missing links, posing additional challenges for KGQA, especially for multi-hop KGQA. Recent research on multi-hop KGQA has attempted to handle KG sparsity using relevant external text, which isn't always readily available. In a separate line of research, KG embedding methods have been proposed to reduce KG sparsity by performing missing link prediction. Such KG embedding methods, even though highly relevant, have not been explored for multi-hop KGQA so far. We fill this gap in this paper and propose EmbedKGQA. EmbedKGQA is particularly effective in performing multi-hop KGQA over sparse KGs. EmbedKGQA also relaxes the requirement of answer selection from a pre-specified neighborhood, a sub-optimal constraint enforced by previous multi-hop KGQA methods. Through extensive experiments on multiple benchmark datasets, we demonstrate EmbedKGQA's effectiveness over other state-of-the-art baselines.
You can open the
pre-recorded video
in a separate window.
NOTE: The SlidesLive video may display a random order of the authors.
The correct author list is shown at the top of this webpage.
Similar Papers
Low-Dimensional Hyperbolic Knowledge Graph Embeddings
Ines Chami, Adva Wolf, Da-Cheng Juan, Frederic Sala, Sujith Ravi, Christopher Ré,
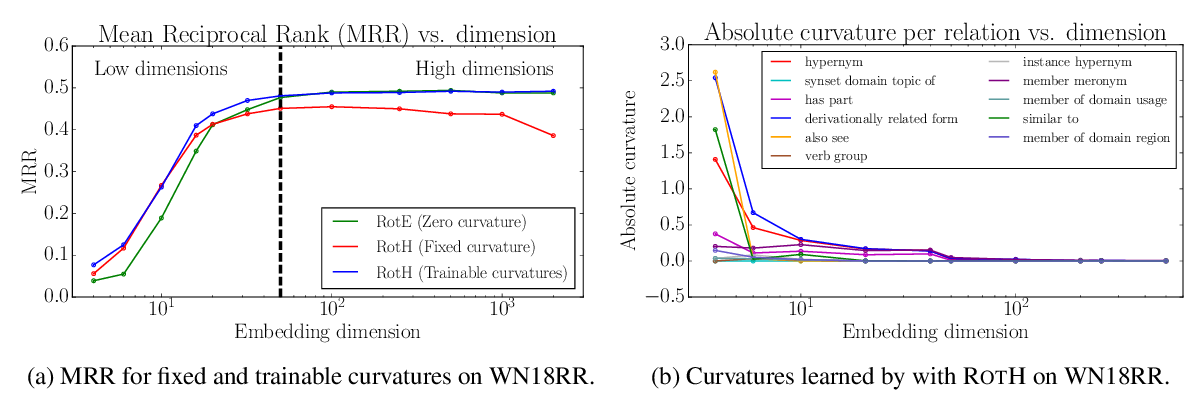
Connecting Embeddings for Knowledge Graph Entity Typing
Yu Zhao, anxiang zhang, Ruobing Xie, Kang Liu, Xiaojie WANG,
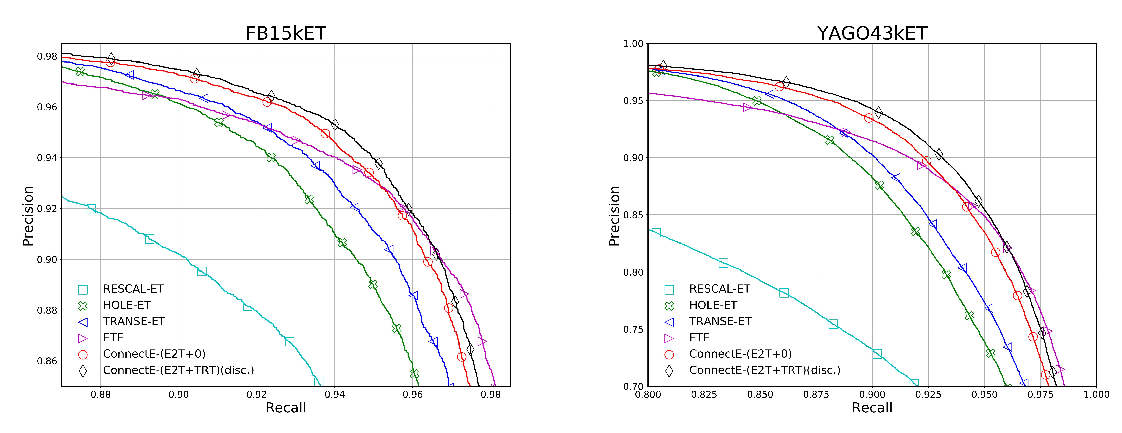
MLQA: Evaluating Cross-lingual Extractive Question Answering
Patrick Lewis, Barlas Oguz, Ruty Rinott, Sebastian Riedel, Holger Schwenk,
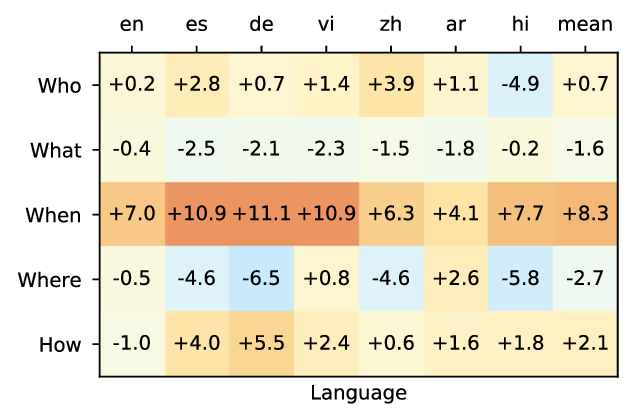