ASSET: A Dataset for Tuning and Evaluation of Sentence Simplification Models with Multiple Rewriting Transformations
Fernando Alva-Manchego, Louis Martin, Antoine Bordes, Carolina Scarton, Benoît Sagot, Lucia Specia
Resources and Evaluation Long Paper
Session 8B: Jul 7
(13:00-14:00 GMT)
Session 9A: Jul 7
(17:00-18:00 GMT)
Abstract:
In order to simplify a sentence, human editors perform multiple rewriting transformations: they split it into several shorter sentences, paraphrase words (i.e. replacing complex words or phrases by simpler synonyms), reorder components, and/or delete information deemed unnecessary. Despite these varied range of possible text alterations, current models for automatic sentence simplification are evaluated using datasets that are focused on a single transformation, such as lexical paraphrasing or splitting. This makes it impossible to understand the ability of simplification models in more realistic settings. To alleviate this limitation, this paper introduces ASSET, a new dataset for assessing sentence simplification in English. ASSET is a crowdsourced multi-reference corpus where each simplification was produced by executing several rewriting transformations. Through quantitative and qualitative experiments, we show that simplifications in ASSET are better at capturing characteristics of simplicity when compared to other standard evaluation datasets for the task. Furthermore, we motivate the need for developing better methods for automatic evaluation using ASSET, since we show that current popular metrics may not be suitable when multiple simplification transformations are performed.
You can open the
pre-recorded video
in a separate window.
NOTE: The SlidesLive video may display a random order of the authors.
The correct author list is shown at the top of this webpage.
Similar Papers
Neural CRF Model for Sentence Alignment in Text Simplification
Chao Jiang, Mounica Maddela, Wuwei Lan, Yang Zhong, Wei Xu,
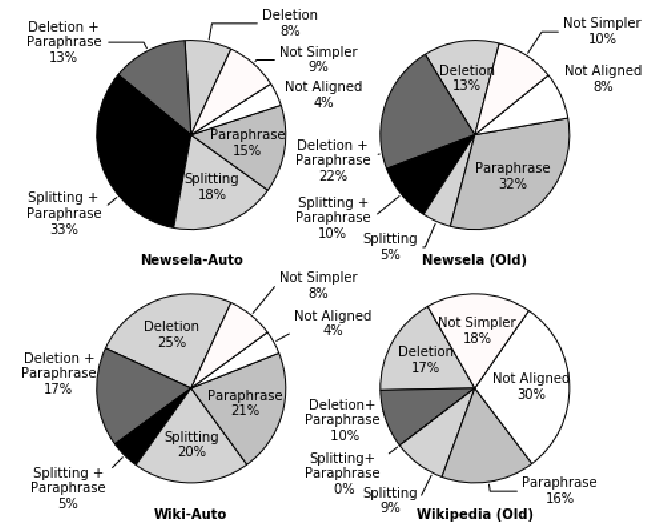
Iterative Edit-Based Unsupervised Sentence Simplification
Dhruv Kumar, Lili Mou, Lukasz Golab, Olga Vechtomova,
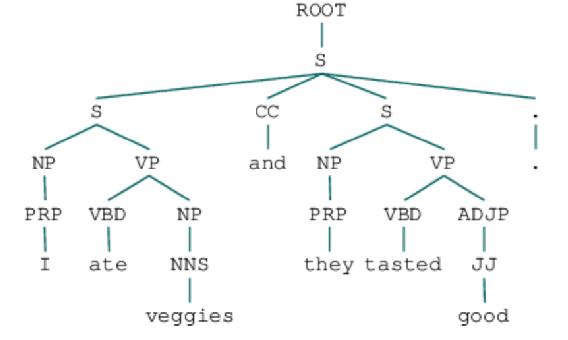
On Faithfulness and Factuality in Abstractive Summarization
Joshua Maynez, Shashi Narayan, Bernd Bohnet, Ryan McDonald,
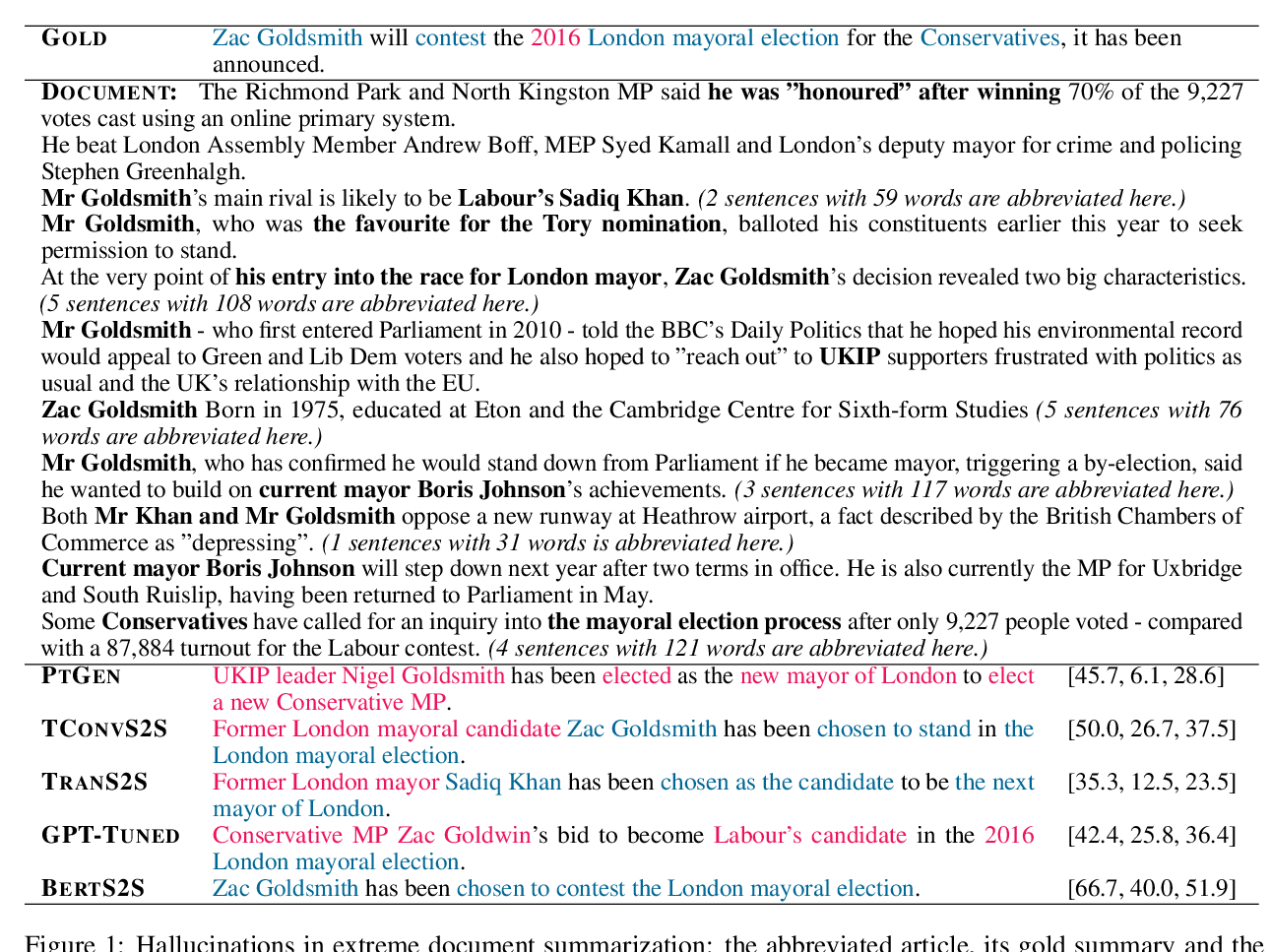
Tangled up in BLEU: Reevaluating the Evaluation of Automatic Machine Translation Evaluation Metrics
Nitika Mathur, Timothy Baldwin, Trevor Cohn,
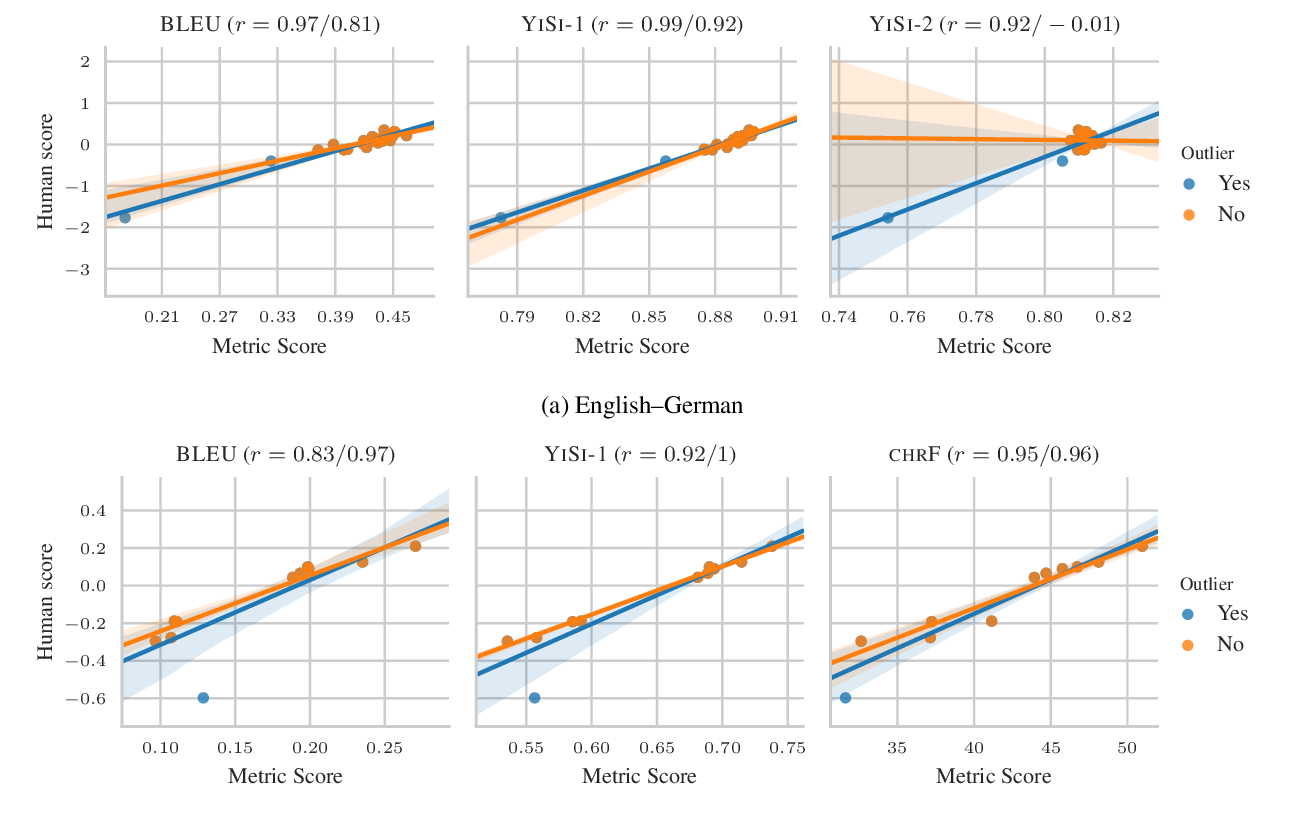