Discrete Latent Variable Representations for Low-Resource Text Classification
Shuning Jin, Sam Wiseman, Karl Stratos, Karen Livescu
Machine Learning for NLP Long Paper
Session 9A: Jul 7
(17:00-18:00 GMT)
Session 10B: Jul 7
(21:00-22:00 GMT)
Abstract:
While much work on deep latent variable models of text uses continuous latent variables, discrete latent variables are interesting because they are more interpretable and typically more space efficient. We consider several approaches to learning discrete latent variable models for text in the case where exact marginalization over these variables is intractable. We compare the performance of the learned representations as features for low-resource document and sentence classification. Our best models outperform the previous best reported results with continuous representations in these low-resource settings, while learning significantly more compressed representations. Interestingly, we find that an amortized variant of Hard EM performs particularly well in the lowest-resource regimes.
You can open the
pre-recorded video
in a separate window.
NOTE: The SlidesLive video may display a random order of the authors.
The correct author list is shown at the top of this webpage.
Similar Papers
Variational Neural Machine Translation with Normalizing Flows
Hendra Setiawan, Matthias Sperber, Udhyakumar Nallasamy, Matthias Paulik,
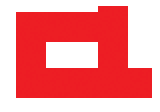
Addressing Posterior Collapse with Mutual Information for Improved Variational Neural Machine Translation
Arya D. McCarthy, Xian Li, Jiatao Gu, Ning Dong,
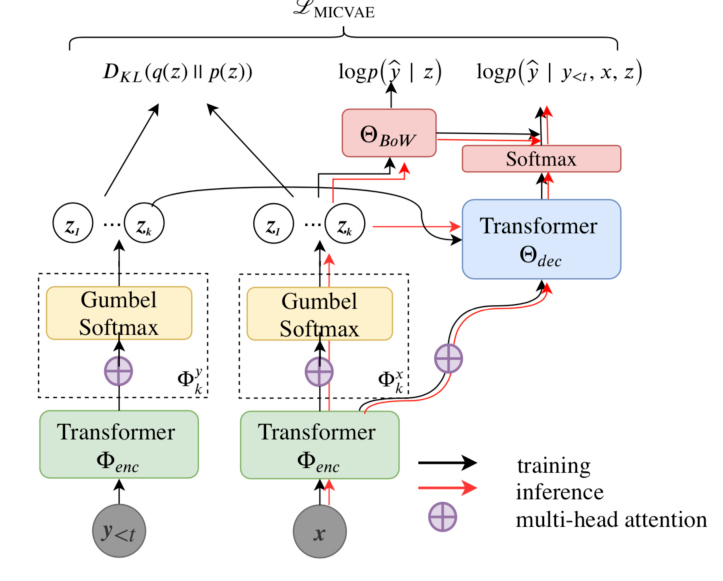
Hypernymy Detection for Low-Resource Languages via Meta Learning
Changlong Yu, Jialong Han, Haisong Zhang, Wilfred Ng,
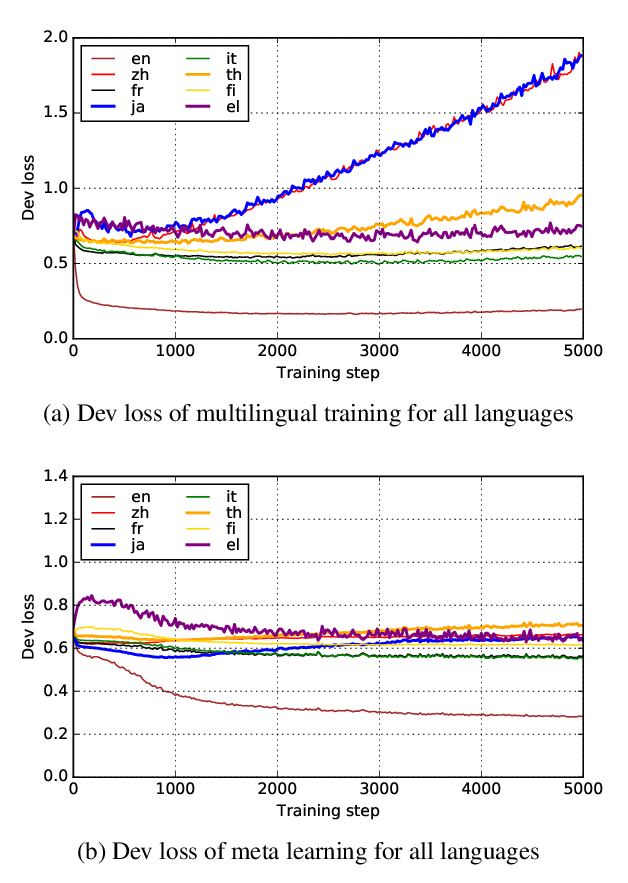
Predicting Depression in Screening Interviews from Latent Categorization of Interview Prompts
Alex Rinaldi, Jean Fox Tree, Snigdha Chaturvedi,
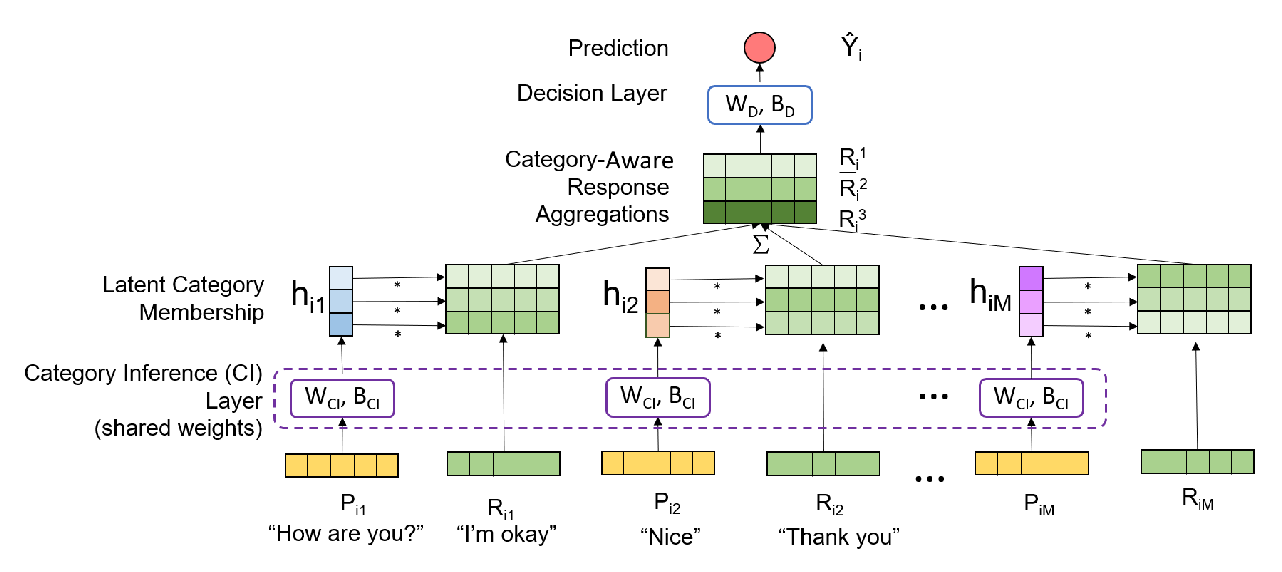