Variational Neural Machine Translation with Normalizing Flows
Hendra Setiawan, Matthias Sperber, Udhyakumar Nallasamy, Matthias Paulik
Machine Translation Short Paper
Session 13B: Jul 8
(13:00-14:00 GMT)
Session 14B: Jul 8
(18:00-19:00 GMT)
Abstract:
Variational Neural Machine Translation (VNMT) is an attractive framework for modeling the generation of target translations, conditioned not only on the source sentence but also on some latent random variables. The latent variable modeling may introduce useful statistical dependencies that can improve translation accuracy. Unfortunately, learning informative latent variables is non-trivial, as the latent space can be prohibitively large, and the latent codes are prone to be ignored by many translation models at training time. Previous works impose strong assumptions on the distribution of the latent code and limit the choice of the NMT architecture. In this paper, we propose to apply the VNMT framework to the state-of-the-art Transformer and introduce a more flexible approximate posterior based on normalizing flows. We demonstrate the efficacy of our proposal under both in-domain and out-of-domain conditions, significantly outperforming strong baselines.
You can open the
pre-recorded video
in a separate window.
NOTE: The SlidesLive video may display a random order of the authors.
The correct author list is shown at the top of this webpage.
Similar Papers
Addressing Posterior Collapse with Mutual Information for Improved Variational Neural Machine Translation
Arya D. McCarthy, Xian Li, Jiatao Gu, Ning Dong,
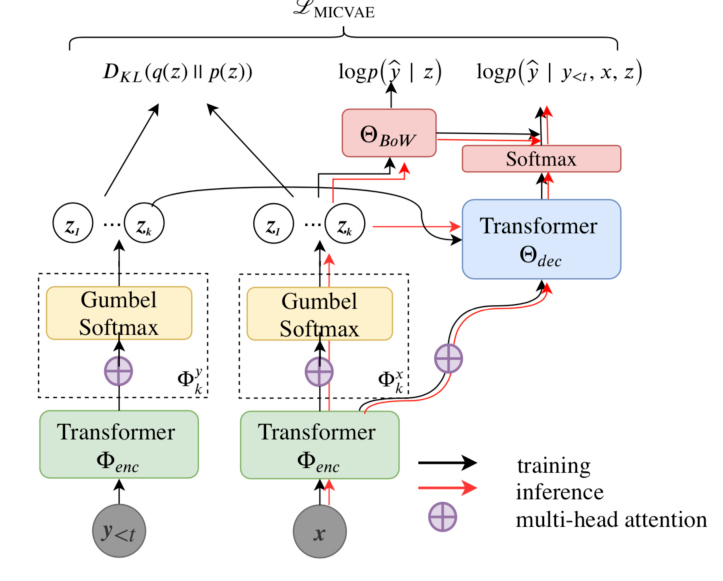
Discrete Latent Variable Representations for Low-Resource Text Classification
Shuning Jin, Sam Wiseman, Karl Stratos, Karen Livescu,
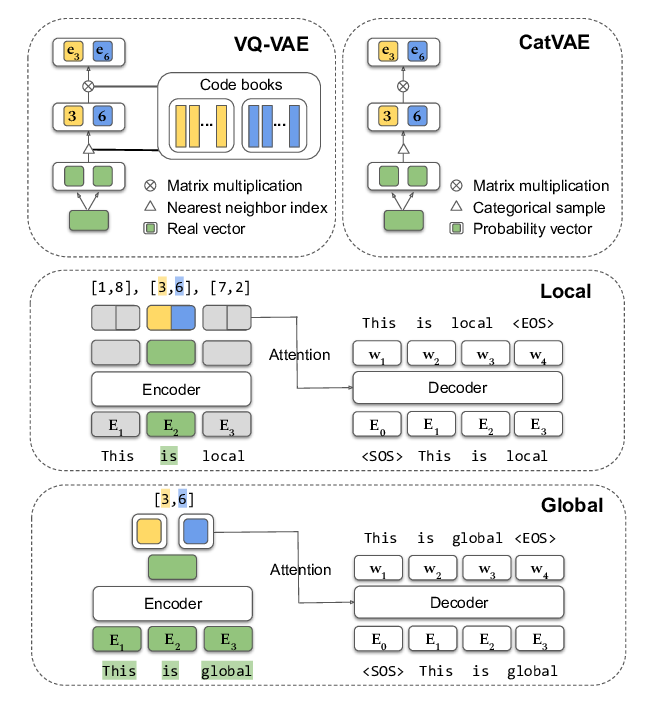
A Batch Normalized Inference Network Keeps the KL Vanishing Away
Qile Zhu, Wei Bi, Xiaojiang Liu, Xiyao Ma, Xiaolin Li, Dapeng Wu,
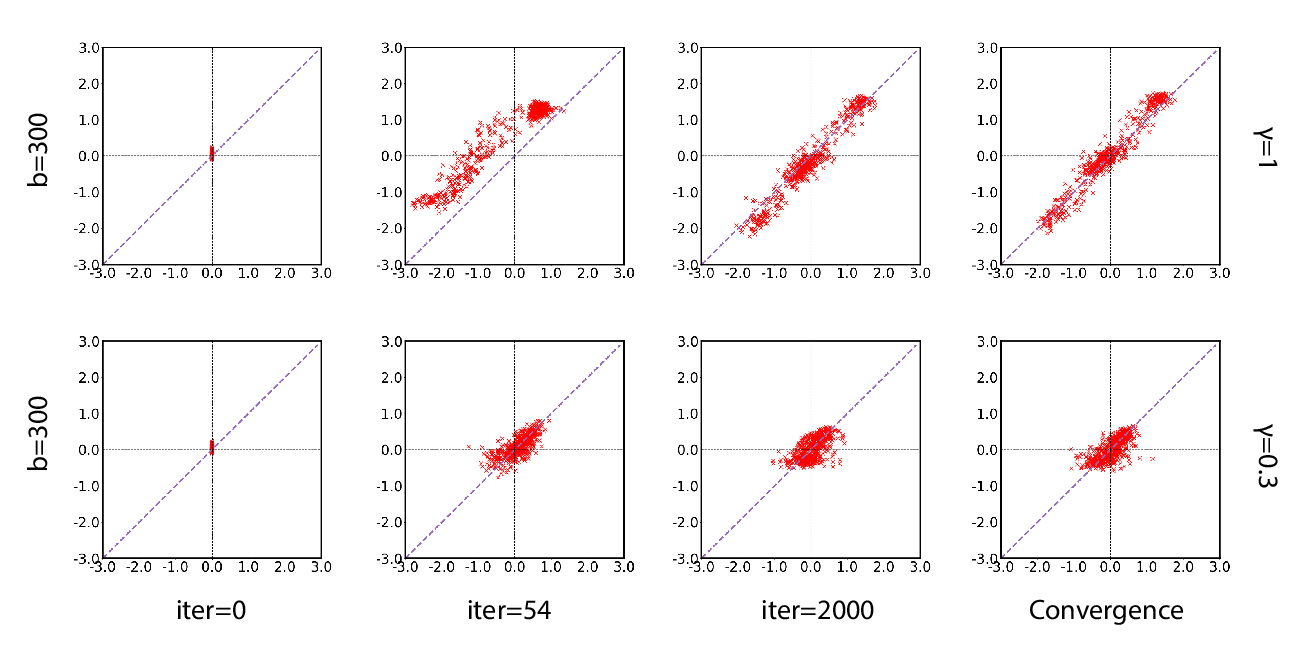
On the Encoder-Decoder Incompatibility in Variational Text Modeling and Beyond
Chen Wu, Prince Zizhuang Wang, William Yang Wang,
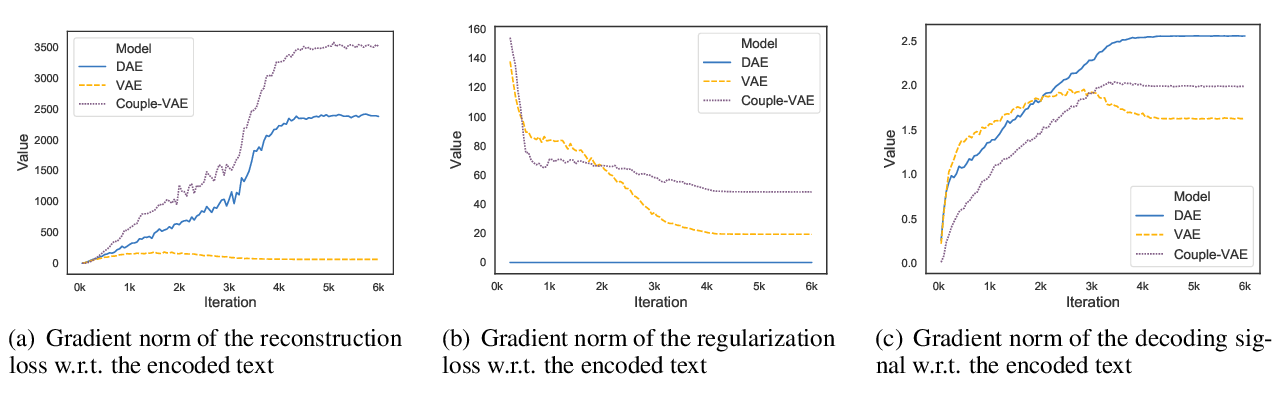