Discrete Optimization for Unsupervised Sentence Summarization with Word-Level Extraction
Raphael Schumann, Lili Mou, Yao Lu, Olga Vechtomova, Katja Markert
Summarization Long Paper
Session 9A: Jul 7
(17:00-18:00 GMT)
Session 10B: Jul 7
(21:00-22:00 GMT)
Abstract:
Automatic sentence summarization produces a shorter version of a sentence, while preserving its most important information. A good summary is characterized by language fluency and high information overlap with the source sentence. We model these two aspects in an unsupervised objective function, consisting of language modeling and semantic similarity metrics. We search for a high-scoring summary by discrete optimization. Our proposed method achieves a new state-of-the art for unsupervised sentence summarization according to ROUGE scores. Additionally, we demonstrate that the commonly reported ROUGE F1 metric is sensitive to summary length. Since this is unwillingly exploited in recent work, we emphasize that future evaluation should explicitly group summarization systems by output length brackets.
You can open the
pre-recorded video
in a separate window.
NOTE: The SlidesLive video may display a random order of the authors.
The correct author list is shown at the top of this webpage.
Similar Papers
The Summary Loop: Learning to Write Abstractive Summaries Without Examples
Philippe Laban, Andrew Hsi, John Canny, Marti A. Hearst,
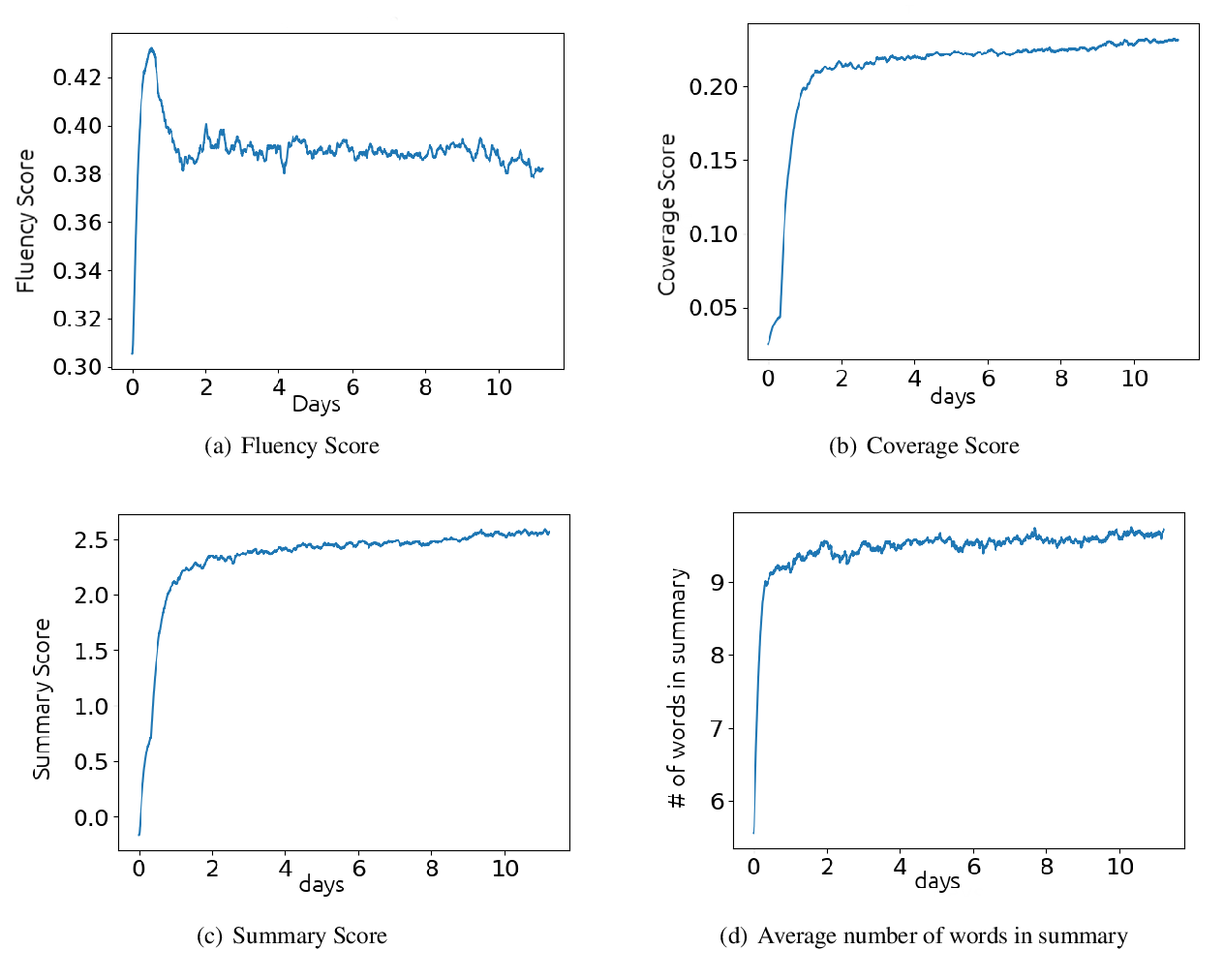
Optimizing the Factual Correctness of a Summary: A Study of Summarizing Radiology Reports
Yuhao Zhang, Derek Merck, Emily Tsai, Christopher D. Manning, Curtis Langlotz,
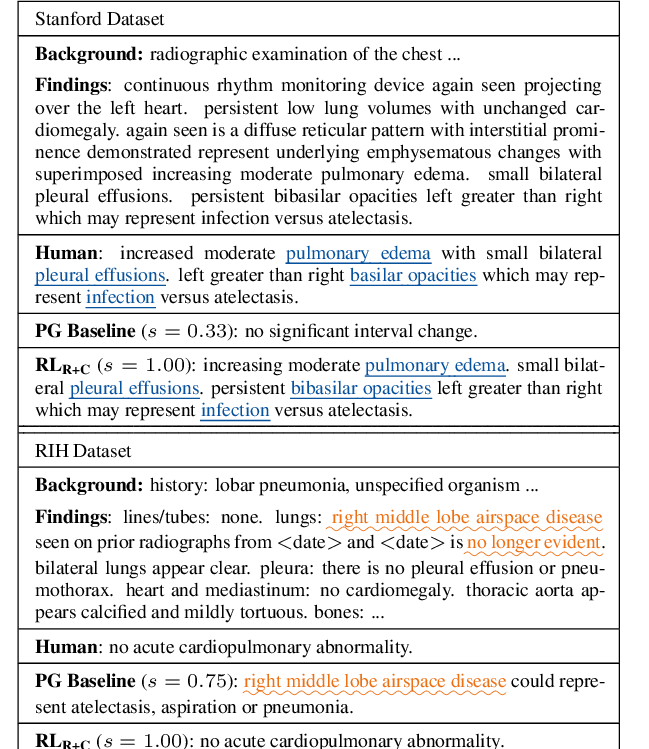
Exploring Content Selection in Summarization of Novel Chapters
Faisal Ladhak, Bryan Li, Yaser Al-Onaizan, Kathy McKeown,
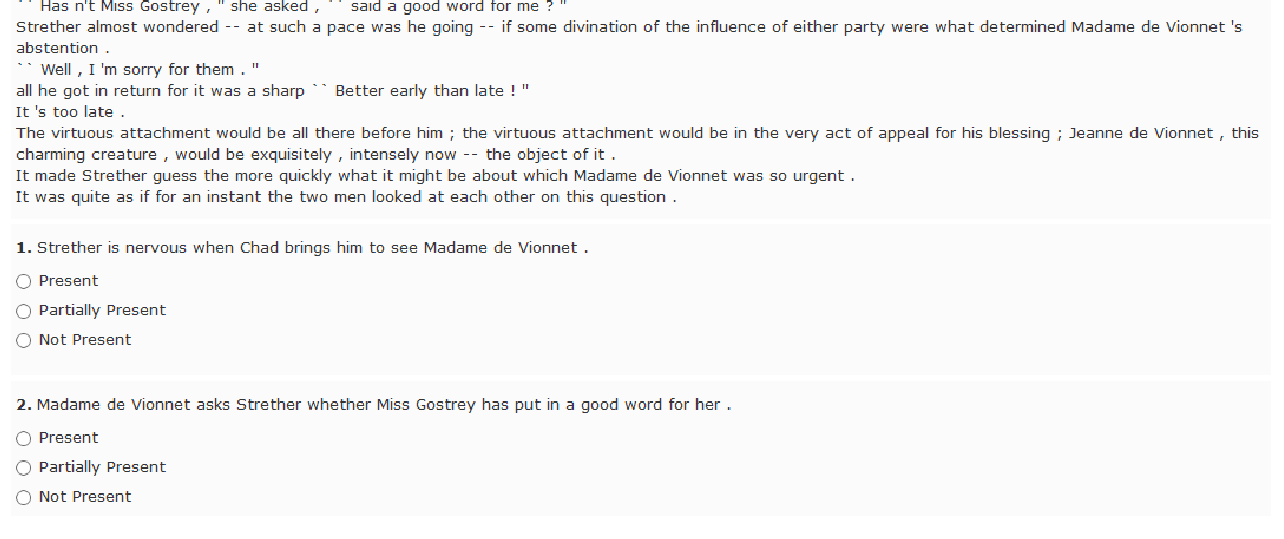
On Faithfulness and Factuality in Abstractive Summarization
Joshua Maynez, Shashi Narayan, Bernd Bohnet, Ryan McDonald,
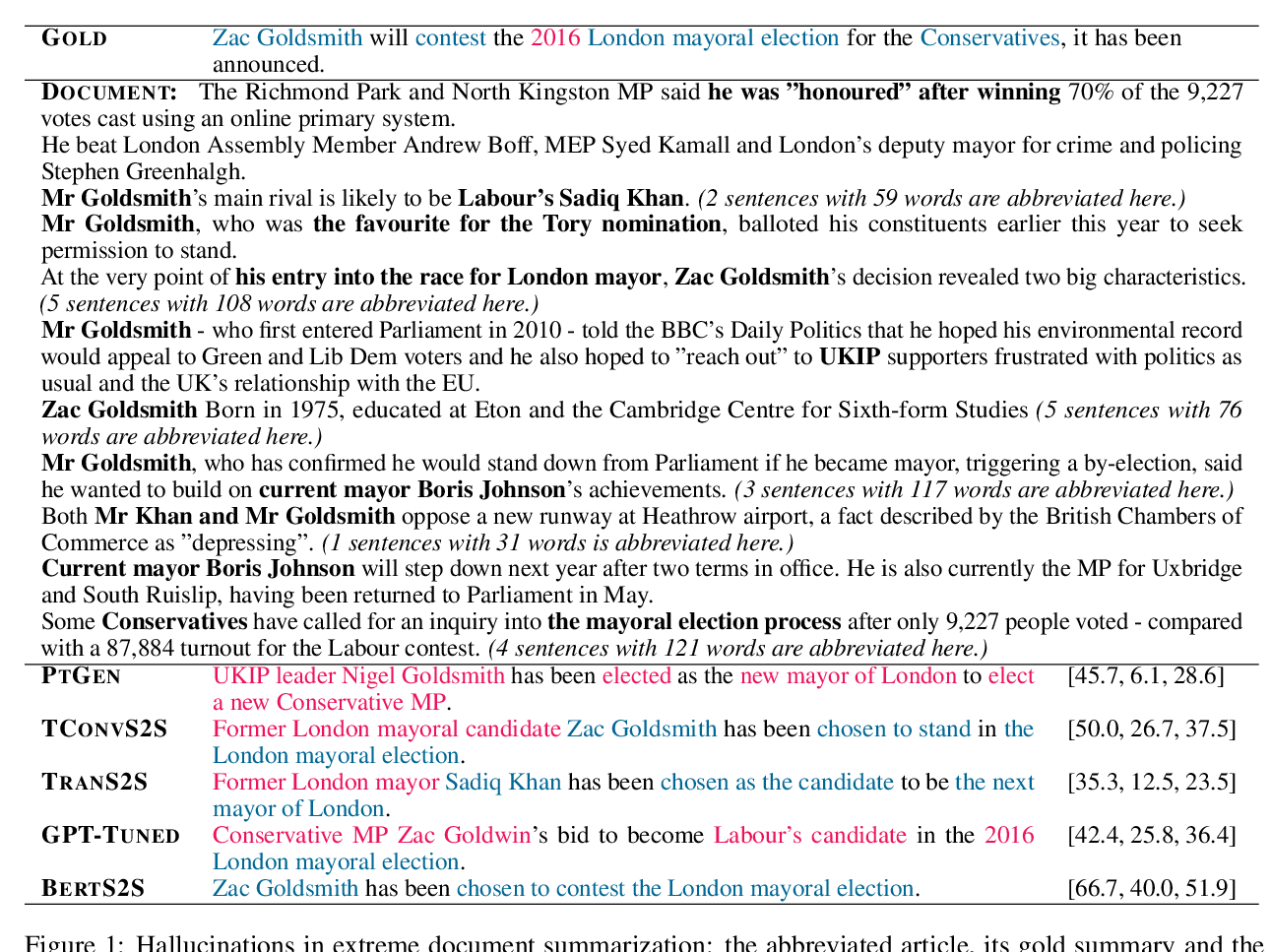