Optimizing the Factual Correctness of a Summary: A Study of Summarizing Radiology Reports
Yuhao Zhang, Derek Merck, Emily Tsai, Christopher D. Manning, Curtis Langlotz
Summarization Long Paper
Session 9A: Jul 7
(17:00-18:00 GMT)
Session 10B: Jul 7
(21:00-22:00 GMT)
Abstract:
Neural abstractive summarization models are able to generate summaries which have high overlap with human references. However, existing models are not optimized for factual correctness, a critical metric in real-world applications. In this work, we develop a general framework where we evaluate the factual correctness of a generated summary by fact-checking it automatically against its reference using an information extraction module. We further propose a training strategy which optimizes a neural summarization model with a factual correctness reward via reinforcement learning. We apply the proposed method to the summarization of radiology reports, where factual correctness is a key requirement. On two separate datasets collected from hospitals, we show via both automatic and human evaluation that the proposed approach substantially improves the factual correctness and overall quality of outputs over a competitive neural summarization system, producing radiology summaries that approach the quality of human-authored ones.
You can open the
pre-recorded video
in a separate window.
NOTE: The SlidesLive video may display a random order of the authors.
The correct author list is shown at the top of this webpage.
Similar Papers
FEQA: A Question Answering Evaluation Framework for Faithfulness Assessment in Abstractive Summarization
Esin Durmus, He He, Mona Diab,
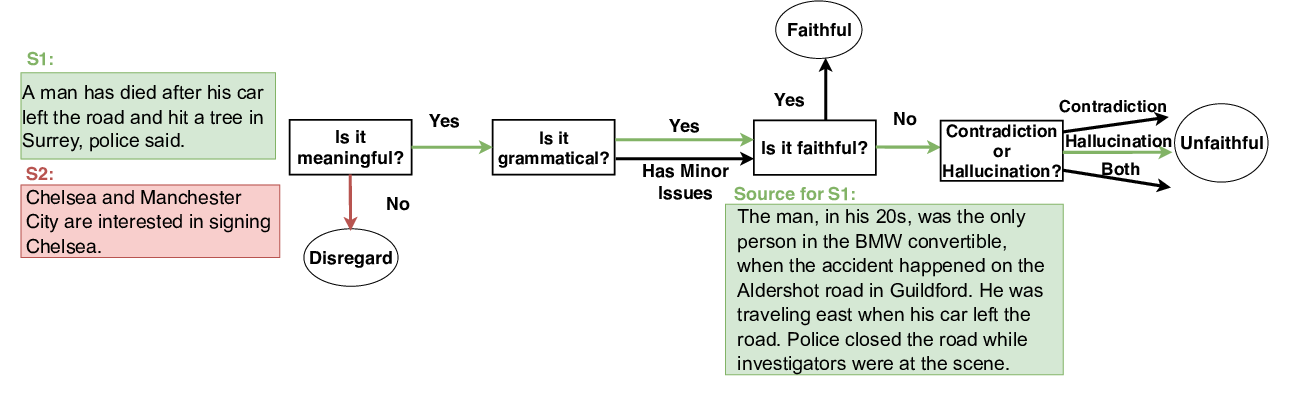
On Faithfulness and Factuality in Abstractive Summarization
Joshua Maynez, Shashi Narayan, Bernd Bohnet, Ryan McDonald,
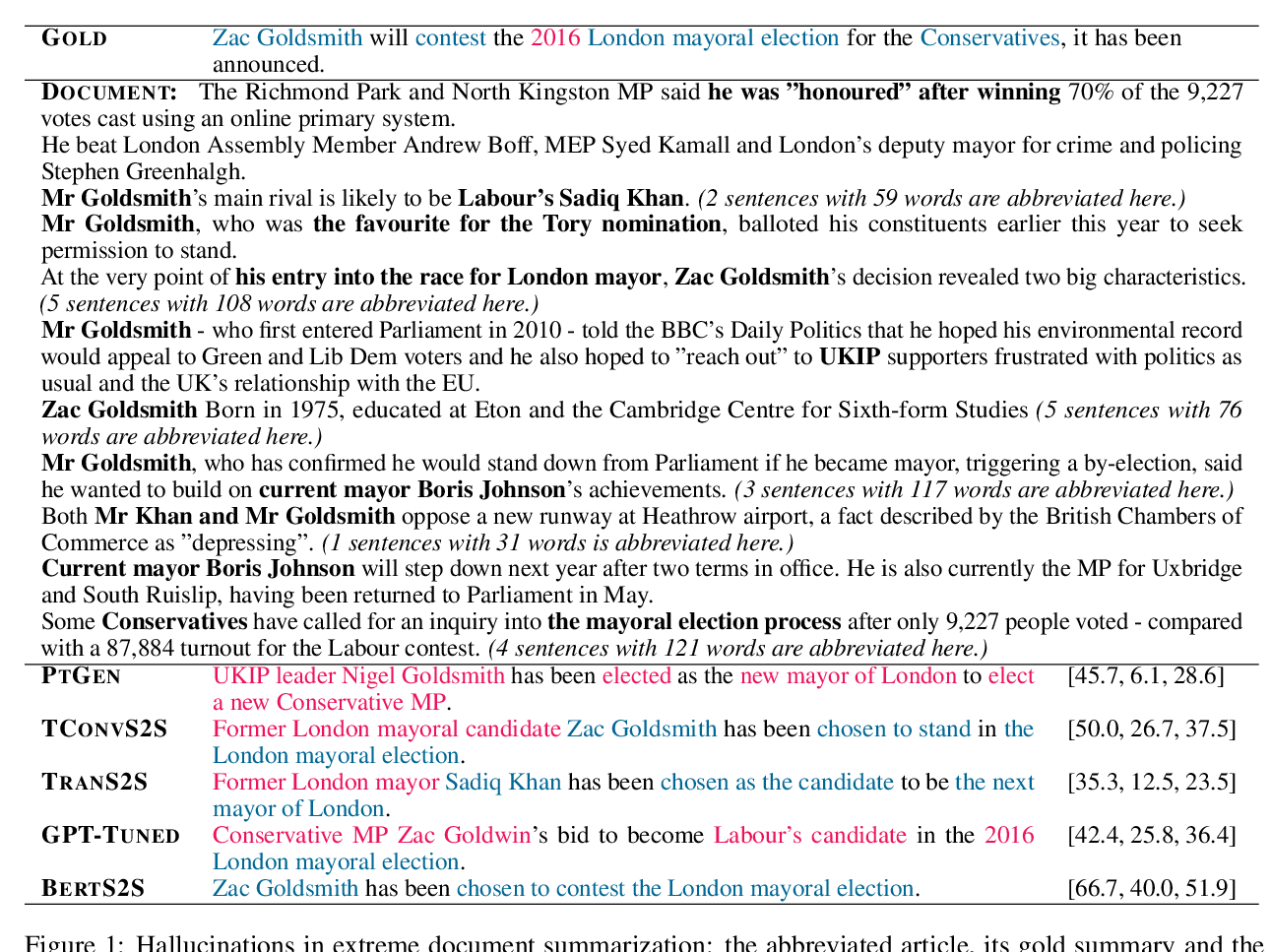
Asking and Answering Questions to Evaluate the Factual Consistency of Summaries
Alex Wang, Kyunghyun Cho, Mike Lewis,
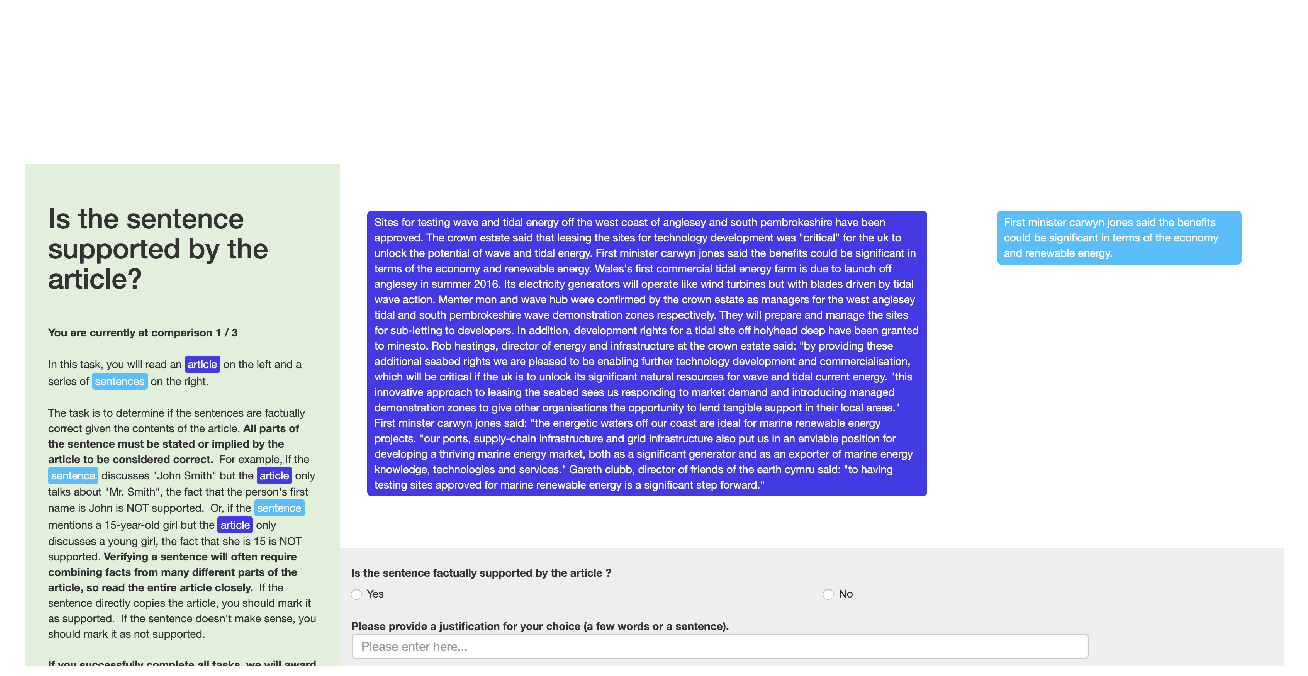
Discrete Optimization for Unsupervised Sentence Summarization with Word-Level Extraction
Raphael Schumann, Lili Mou, Yao Lu, Olga Vechtomova, Katja Markert,
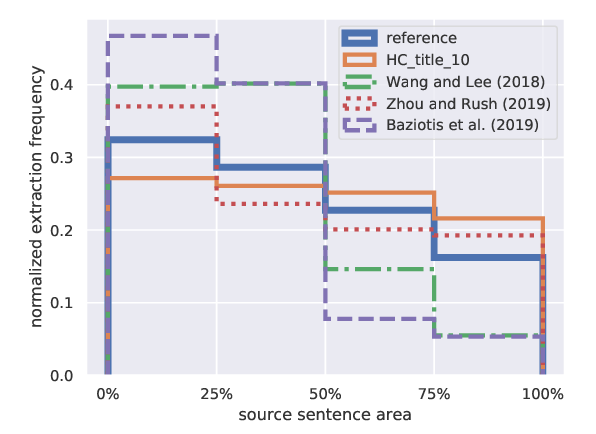