Would you Rather? A New Benchmark for Learning Machine Alignment with Cultural Values and Social Preferences
Yi Tay, Donovan Ong, Jie Fu, Alvin Chan, Nancy Chen, Anh Tuan Luu, Chris Pal
Computational Social Science and Social Media Short Paper
Session 9B: Jul 7
(18:00-19:00 GMT)
Session 10A: Jul 7
(20:00-21:00 GMT)
Abstract:
Understanding human preferences, along with cultural and social nuances, lives at the heart of natural language understanding. Concretely, we present a new task and corpus for learning alignments between machine and human preferences. Our newly introduced problem is concerned with predicting the preferable options from two sentences describing scenarios that may involve social, cultural, ethical, or moral situations. Our problem is framed as a natural language inference task with crowd-sourced preference votes by human players, obtained from a gamified voting platform. Along with the release of a new dataset of 200K data points, we benchmark several state-of-the-art neural models, along with BERT and friends on this task. Our experimental results show that current state-of-the-art NLP models still leave much room for improvement.
You can open the
pre-recorded video
in a separate window.
NOTE: The SlidesLive video may display a random order of the authors.
The correct author list is shown at the top of this webpage.
Similar Papers
Social Bias Frames: Reasoning about Social and Power Implications of Language
Maarten Sap, Saadia Gabriel, Lianhui Qin, Dan Jurafsky, Noah A. Smith, Yejin Choi,
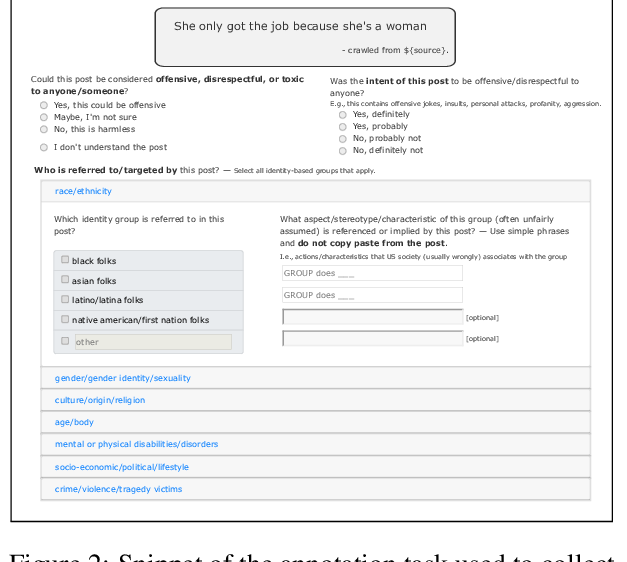
Adversarial NLI: A New Benchmark for Natural Language Understanding
Yixin Nie, Adina Williams, Emily Dinan, Mohit Bansal, Jason Weston, Douwe Kiela,
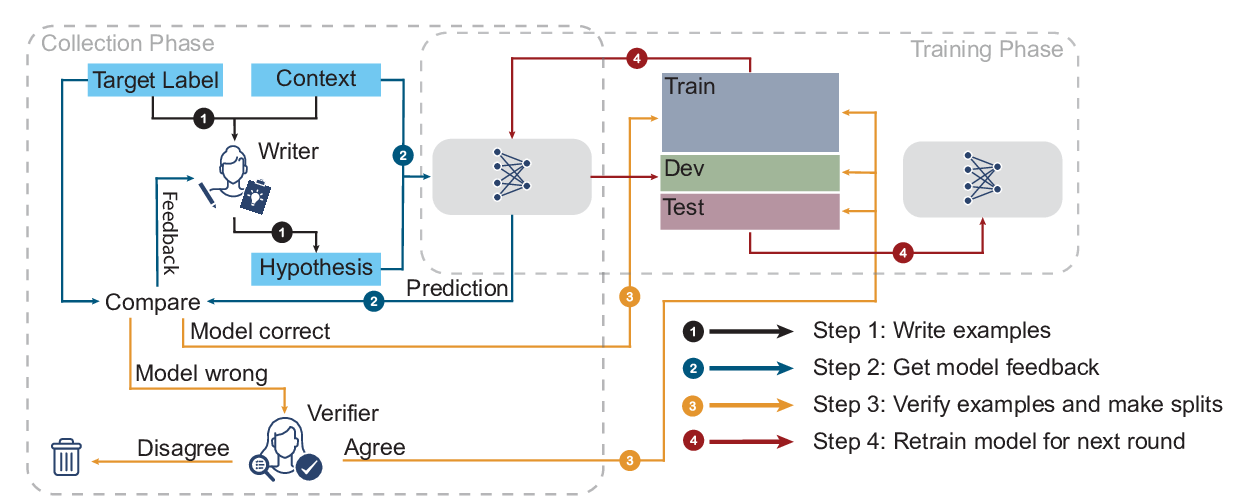
Expertise Style Transfer: A New Task Towards Better Communication between Experts and Laymen
Yixin Cao, Ruihao Shui, Liangming Pan, Min-Yen Kan, Zhiyuan Liu, Tat-Seng Chua,
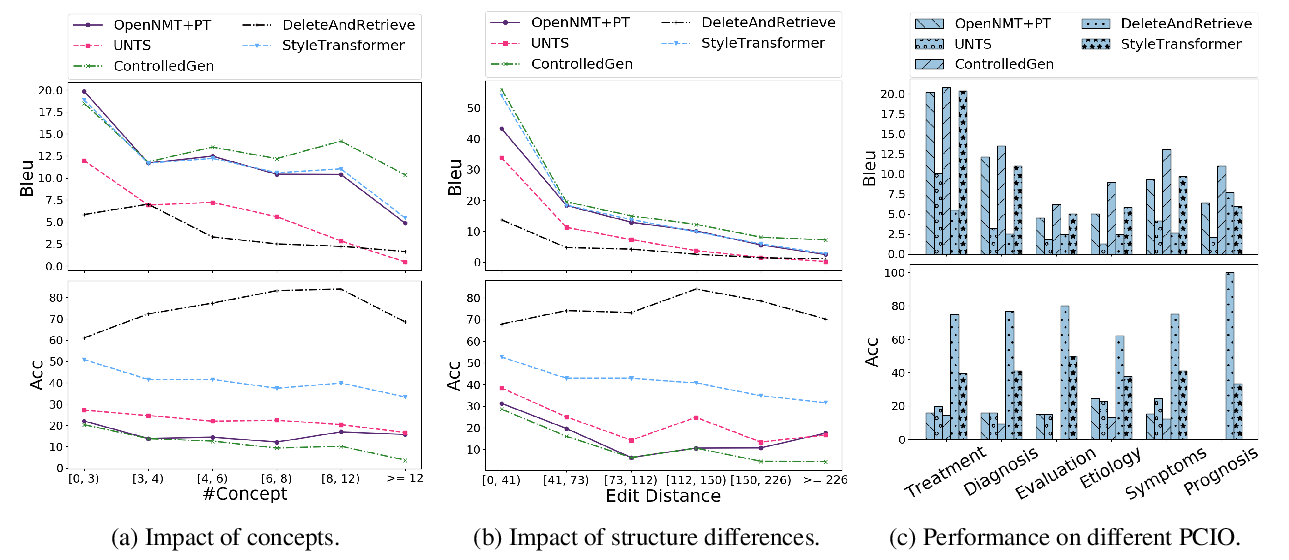
Will-They-Won't-They: A Very Large Dataset for Stance Detection on Twitter
Costanza Conforti, Jakob Berndt, Mohammad Taher Pilehvar, Chryssi Giannitsarou, Flavio Toxvaerd, Nigel Collier,
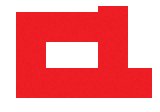