Adversarial NLI: A New Benchmark for Natural Language Understanding
Yixin Nie, Adina Williams, Emily Dinan, Mohit Bansal, Jason Weston, Douwe Kiela
Resources and Evaluation Long Paper
Session 9A: Jul 7
(17:00-18:00 GMT)
Session 10B: Jul 7
(21:00-22:00 GMT)
Abstract:
We introduce a new large-scale NLI benchmark dataset, collected via an iterative, adversarial human-and-model-in-the-loop procedure. We show that training models on this new dataset leads to state-of-the-art performance on a variety of popular NLI benchmarks, while posing a more difficult challenge with its new test set. Our analysis sheds light on the shortcomings of current state-of-the-art models, and shows that non-expert annotators are successful at finding their weaknesses. The data collection method can be applied in a never-ending learning scenario, becoming a moving target for NLU, rather than a static benchmark that will quickly saturate.
You can open the
pre-recorded video
in a separate window.
NOTE: The SlidesLive video may display a random order of the authors.
The correct author list is shown at the top of this webpage.
Similar Papers
SMART: Robust and Efficient Fine-Tuning for Pre-trained Natural Language Models through Principled Regularized Optimization
Haoming Jiang, Pengcheng He, Weizhu Chen, Xiaodong Liu, Jianfeng Gao, Tuo Zhao,
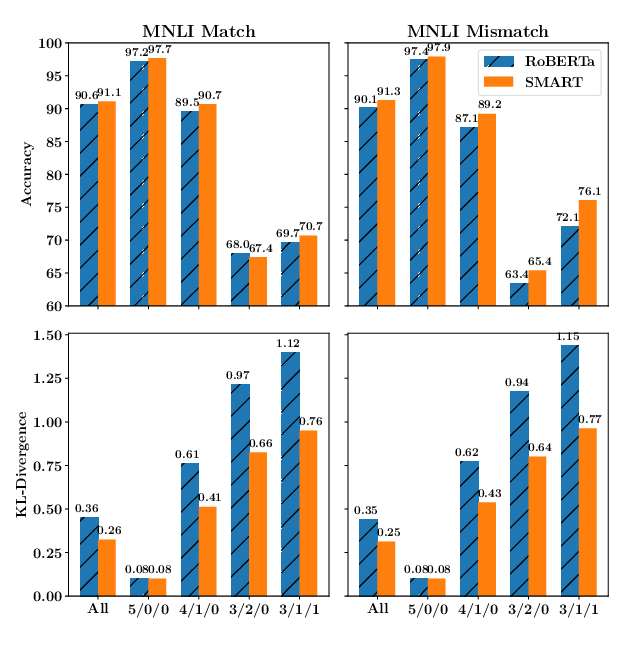
PuzzLing Machines: A Challenge on Learning From Small Data
Gözde Gül Şahin, Yova Kementchedjhieva, Phillip Rust, Iryna Gurevych,
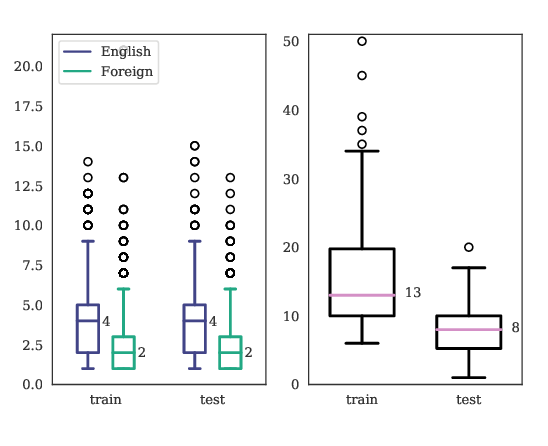
Would you Rather? A New Benchmark for Learning Machine Alignment with Cultural Values and Social Preferences
Yi Tay, Donovan Ong, Jie Fu, Alvin Chan, Nancy Chen, Anh Tuan Luu, Chris Pal,
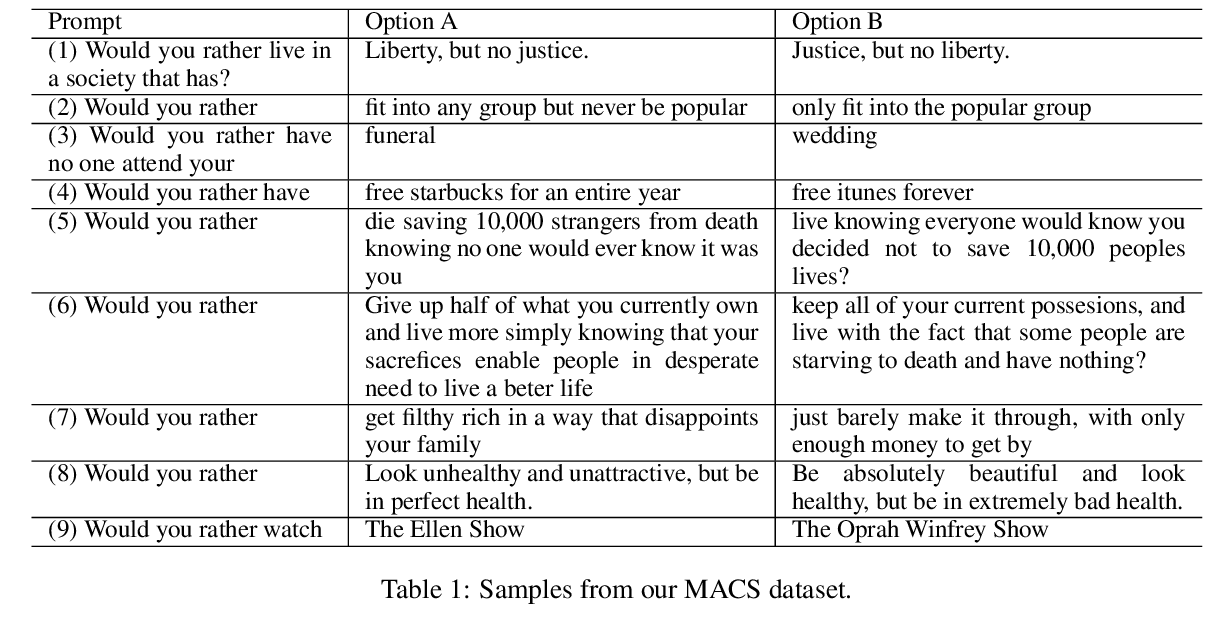
Mind the Trade-off: Debiasing NLU Models without Degrading the In-distribution Performance
Prasetya Ajie Utama, Nafise Sadat Moosavi, Iryna Gurevych,
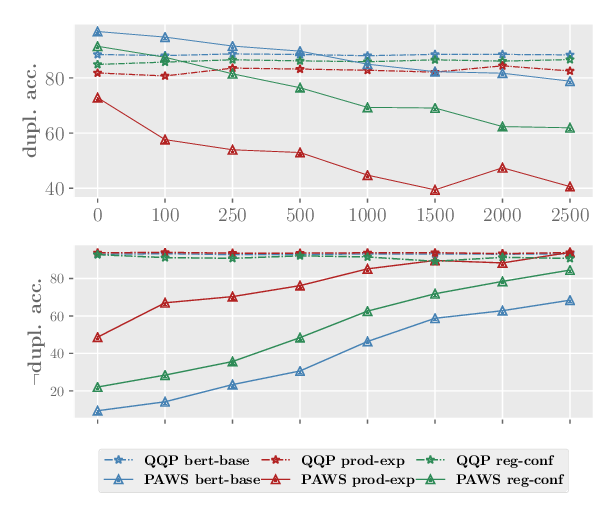