Contextualizing Hate Speech Classifiers with Post-hoc Explanation
Brendan Kennedy, Xisen Jin, Aida Mostafazadeh Davani, Morteza Dehghani, Xiang Ren
Ethics and NLP Short Paper
Session 9B: Jul 7
(18:00-19:00 GMT)
Session 10B: Jul 7
(21:00-22:00 GMT)
Abstract:
Hate speech classifiers trained on imbalanced datasets struggle to determine if group identifiers like "gay" or "black" are used in offensive or prejudiced ways. Such biases manifest in false positives when these identifiers are present, due to models' inability to learn the contexts which constitute a hateful usage of identifiers. We extract post-hoc explanations from fine-tuned BERT classifiers to detect bias towards identity terms. Then, we propose a novel regularization technique based on these explanations that encourages models to learn from the context of group identifiers in addition to the identifiers themselves. Our approach improved over baselines in limiting false positives on out-of-domain data while maintaining and in cases improving in-domain performance.
You can open the
pre-recorded video
in a separate window.
NOTE: The SlidesLive video may display a random order of the authors.
The correct author list is shown at the top of this webpage.
Similar Papers
End-to-End Bias Mitigation by Modelling Biases in Corpora
Rabeeh Karimi Mahabadi, Yonatan Belinkov, James Henderson,
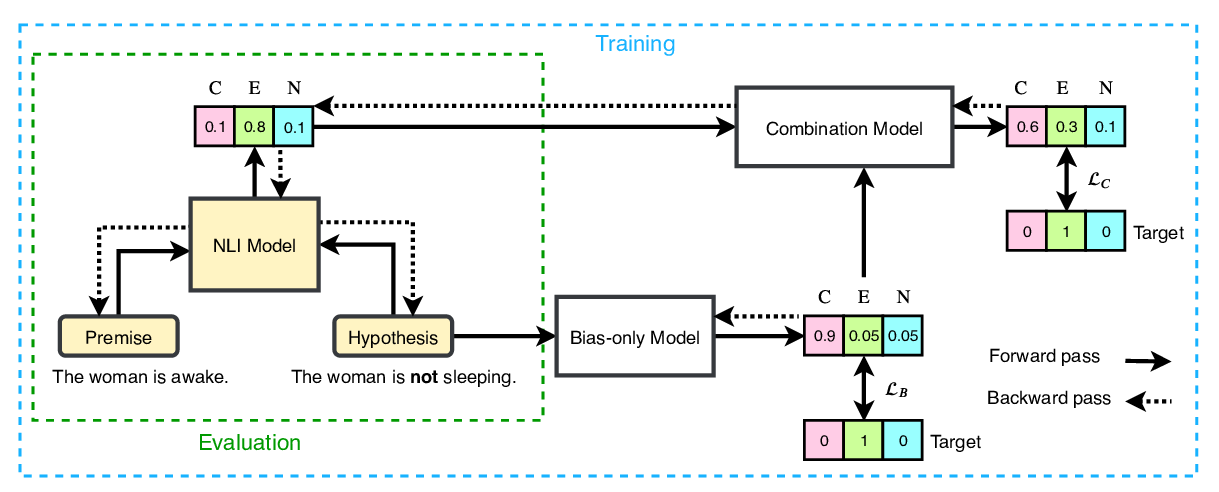
Language (Technology) is Power: A Critical Survey of "Bias" in NLP
Su Lin Blodgett, Solon Barocas, Hal Daumé III, Hanna Wallach,
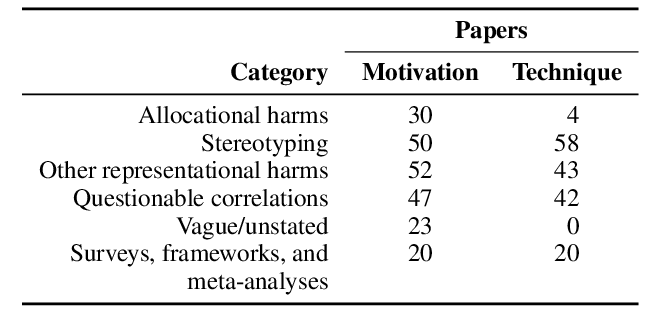
Demographics Should Not Be the Reason of Toxicity: Mitigating Discrimination in Text Classifications with Instance Weighting
Guanhua Zhang, Bing Bai, Junqi Zhang, Kun Bai, Conghui Zhu, Tiejun Zhao,
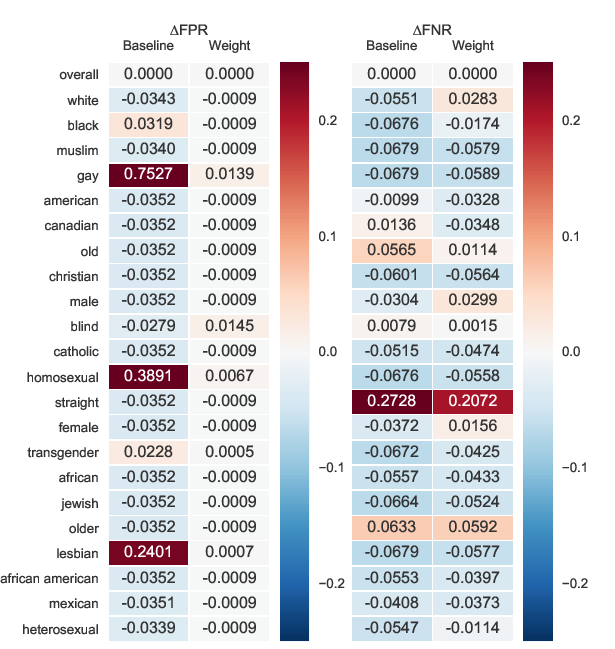
Mind the Trade-off: Debiasing NLU Models without Degrading the In-distribution Performance
Prasetya Ajie Utama, Nafise Sadat Moosavi, Iryna Gurevych,
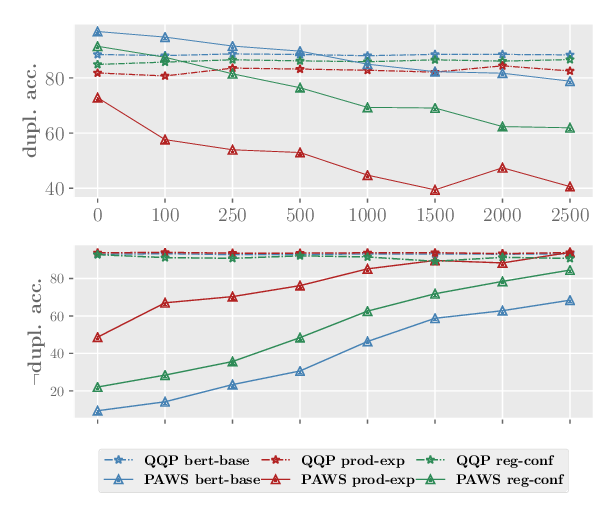