Probabilistic Assumptions Matter: Improved Models for Distantly-Supervised Document-Level Question Answering
Hao Cheng, Ming-Wei Chang, Kenton Lee, Kristina Toutanova
Question Answering Long Paper
Session 9B: Jul 7
(18:00-19:00 GMT)
Session 10A: Jul 7
(20:00-21:00 GMT)
Abstract:
We address the problem of extractive question answering using document-level distant super-vision, pairing questions and relevant documents with answer strings. We compare previously used probability space and distant supervision assumptions (assumptions on the correspondence between the weak answer string labels and possible answer mention spans). We show that these assumptions interact, and that different configurations provide complementary benefits. We demonstrate that a multi-objective model can efficiently combine the advantages of multiple assumptions and outperform the best individual formulation. Our approach outperforms previous state-of-the-art models by 4.3 points in F1 on TriviaQA-Wiki and 1.7 points in Rouge-L on NarrativeQA summaries.
You can open the
pre-recorded video
in a separate window.
NOTE: The SlidesLive video may display a random order of the authors.
The correct author list is shown at the top of this webpage.
Similar Papers
Crossing Variational Autoencoders for Answer Retrieval
Wenhao Yu, Lingfei Wu, Qingkai Zeng, Shu Tao, Yu Deng, Meng Jiang,
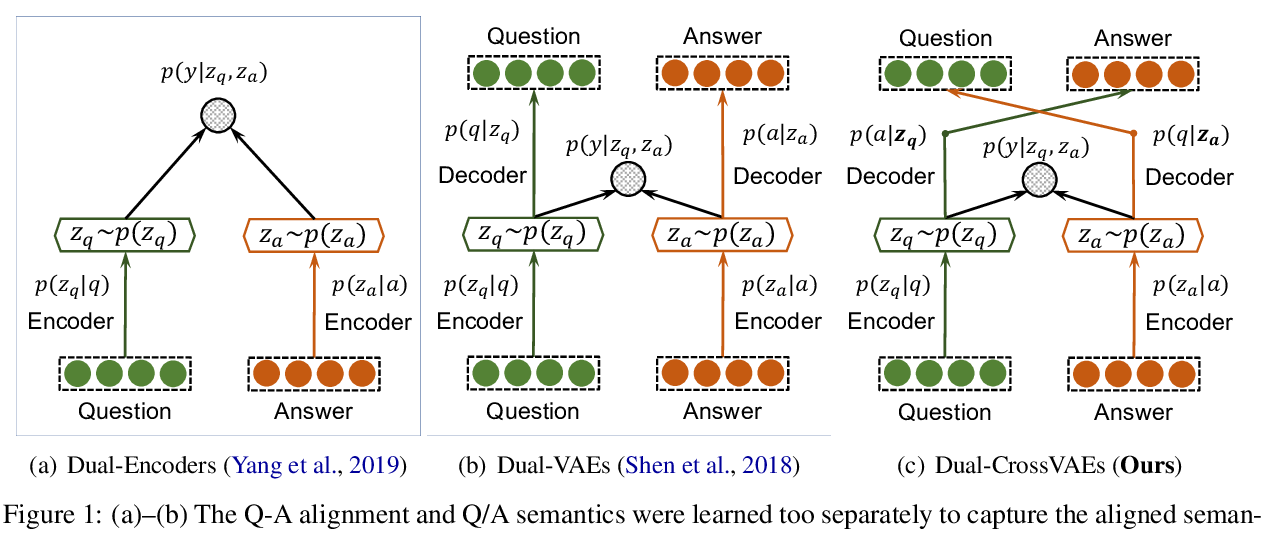
RikiNet: Reading Wikipedia Pages for Natural Question Answering
Dayiheng Liu, Yeyun Gong, Jie Fu, Yu Yan, Jiusheng Chen, Daxin Jiang, Jiancheng Lv, Nan Duan,
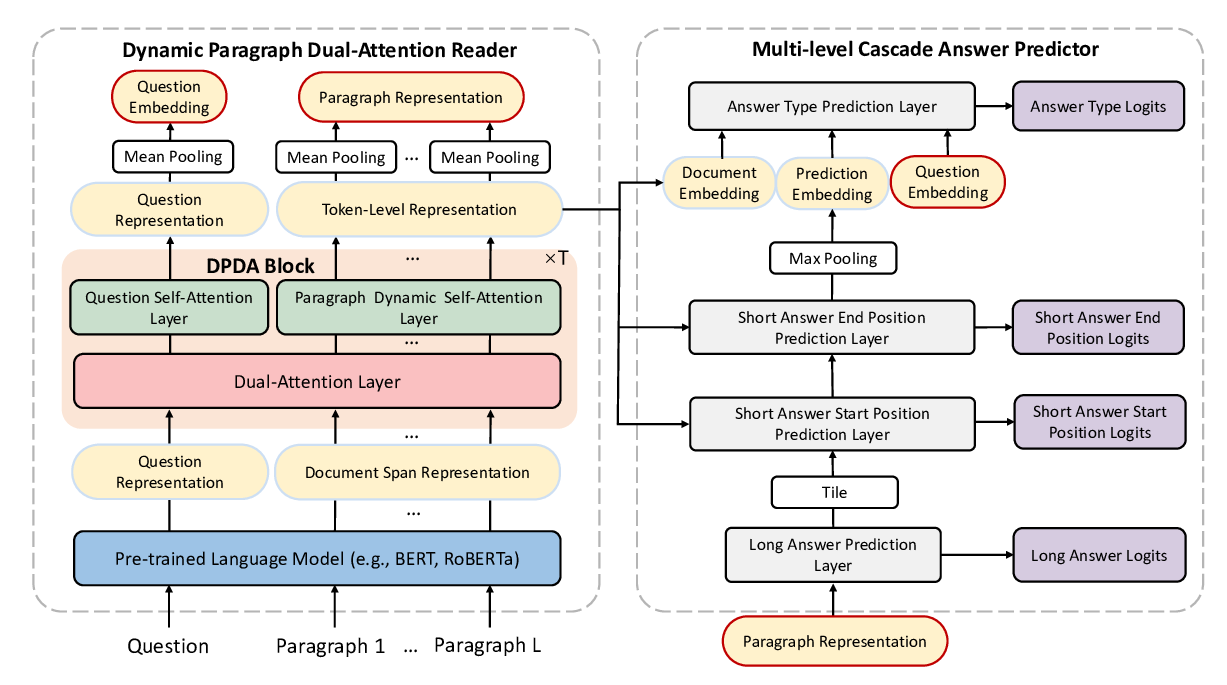
Fluent Response Generation for Conversational Question Answering
Ashutosh Baheti, Alan Ritter, Kevin Small,
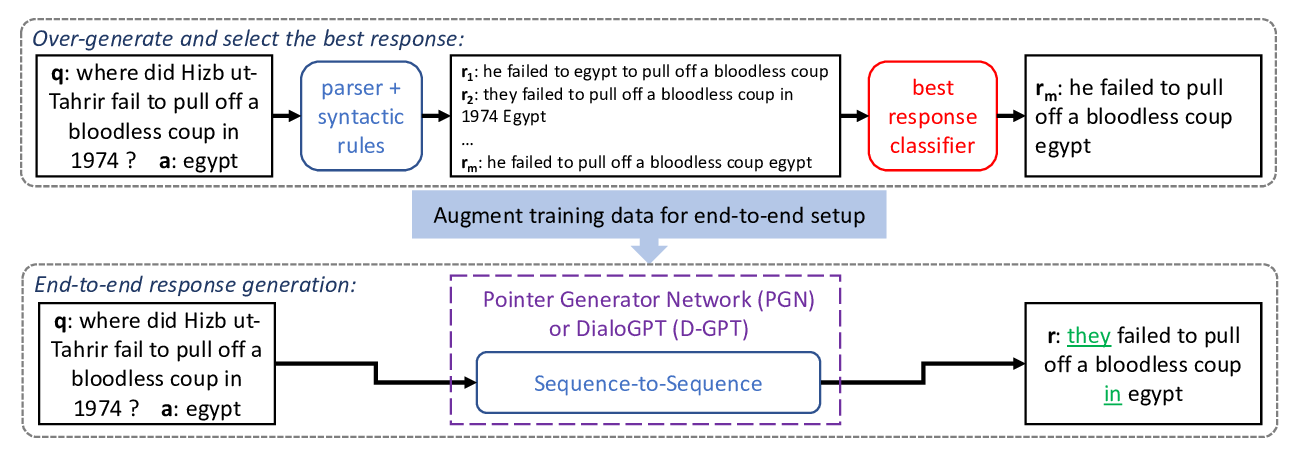
Span Selection Pre-training for Question Answering
Michael Glass, Alfio Gliozzo, Rishav Chakravarti, Anthony Ferritto, Lin Pan, G P Shrivatsa Bhargav, Dinesh Garg, Avi Sil,
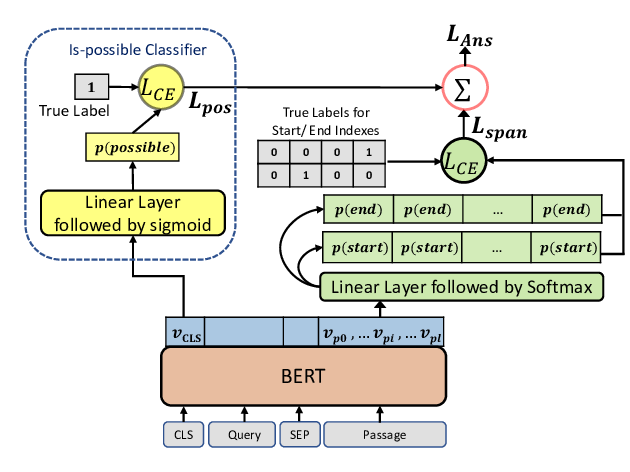