Fluent Response Generation for Conversational Question Answering
Ashutosh Baheti, Alan Ritter, Kevin Small
Generation Long Paper
Session 1A: Jul 6
(05:00-06:00 GMT)
Session 2A: Jul 6
(08:00-09:00 GMT)
Abstract:
Question answering (QA) is an important aspect of open-domain conversational agents, garnering specific research focus in the conversational QA (ConvQA) subtask. One notable limitation of recent ConvQA efforts is the response being answer span extraction from the target corpus, thus ignoring the natural language generation (NLG) aspect of high-quality conversational agents. In this work, we propose a method for situating QA responses within a SEQ2SEQ NLG approach to generate fluent grammatical answer responses while maintaining correctness. From a technical perspective, we use data augmentation to generate training data for an end-to-end system. Specifically, we develop Syntactic Transformations (STs) to produce question-specific candidate answer responses and rank them using a BERT-based classifier (Devlin et al., 2019). Human evaluation on SQuAD 2.0 data (Rajpurkar et al., 2018) demonstrate that the proposed model outperforms baseline CoQA and QuAC models in generating conversational responses. We further show our model's scalability by conducting tests on the CoQA dataset. The code and data are available at https://github.com/abaheti95/QADialogSystem.
You can open the
pre-recorded video
in a separate window.
NOTE: The SlidesLive video may display a random order of the authors.
The correct author list is shown at the top of this webpage.
Similar Papers
Template-Based Question Generation from Retrieved Sentences for Improved Unsupervised Question Answering
Alexander Fabbri, Patrick Ng, Zhiguo Wang, Ramesh Nallapati, Bing Xiang,
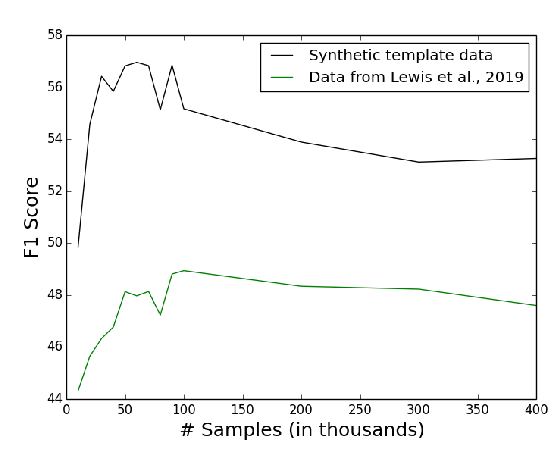
DoQA - Accessing Domain-Specific FAQs via Conversational QA
Jon Ander Campos, Arantxa Otegi, Aitor Soroa, Jan Deriu, Mark Cieliebak, Eneko Agirre,
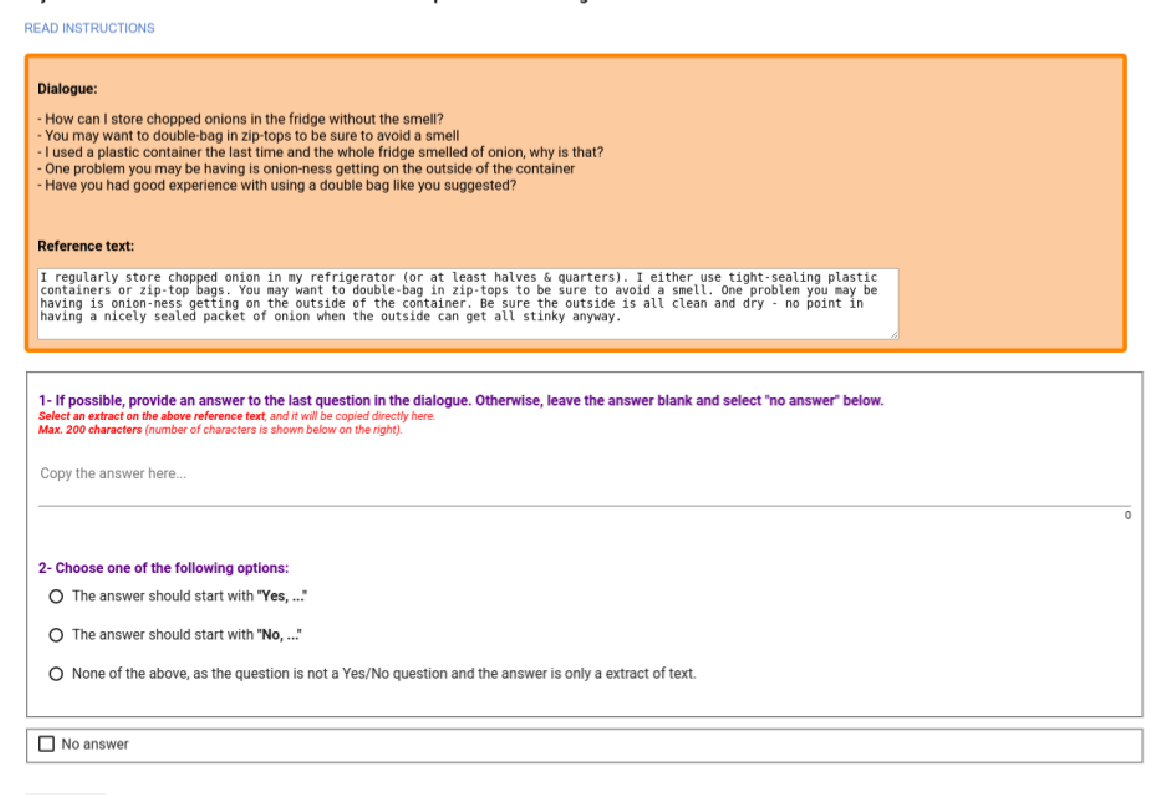
Clinical Reading Comprehension: A Thorough Analysis of the emrQA Dataset
Xiang Yue, Bernal Jimenez Gutierrez, Huan Sun,
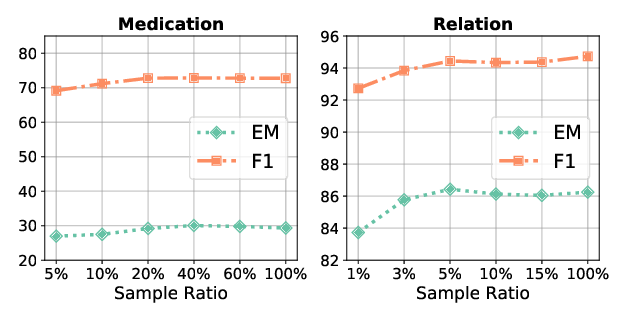
Asking and Answering Questions to Evaluate the Factual Consistency of Summaries
Alex Wang, Kyunghyun Cho, Mike Lewis,
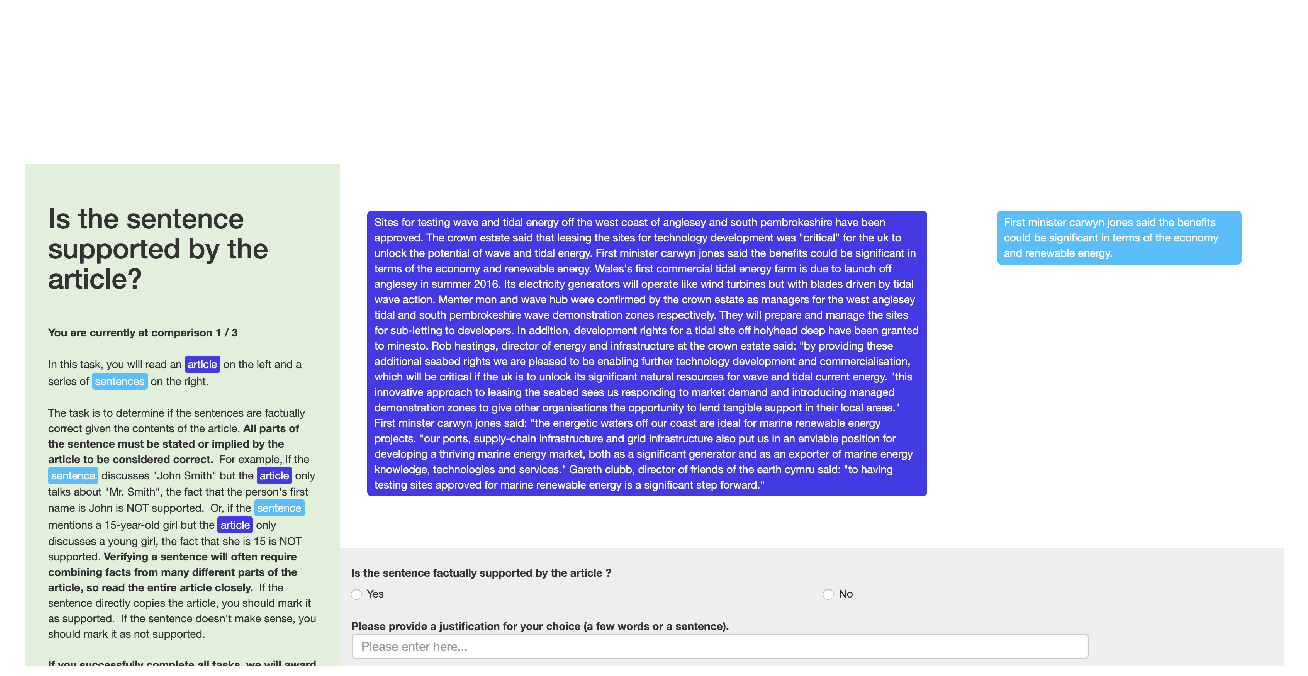