Cross-Lingual Unsupervised Sentiment Classification with Multi-View Transfer Learning
Hongliang Fei, Ping Li
Sentiment Analysis, Stylistic Analysis, and Argument Mining Long Paper
Session 9B: Jul 7
(18:00-19:00 GMT)
Session 10A: Jul 7
(20:00-21:00 GMT)
Abstract:
Recent neural network models have achieved impressive performance on sentiment classification in English as well as other languages. Their success heavily depends on the availability of a large amount of labeled data or parallel corpus. In this paper, we investigate an extreme scenario of cross-lingual sentiment classification, in which the low-resource language does not have any labels or parallel corpus. We propose an unsupervised cross-lingual sentiment classification model named multi-view encoder-classifier (MVEC) that leverages an unsupervised machine translation (UMT) system and a language discriminator. Unlike previous language model (LM) based fine-tuning approaches that adjust parameters solely based on the classification error on training data, we employ the encoder-decoder framework of a UMT as a regularization component on the shared network parameters. In particular, the cross-lingual encoder of our model learns a shared representation, which is effective for both reconstructing input sentences of two languages and generating more representative views from the input for classification. Extensive experiments on five language pairs verify that our model significantly outperforms other models for 8/11 sentiment classification tasks.
You can open the
pre-recorded video
in a separate window.
NOTE: The SlidesLive video may display a random order of the authors.
The correct author list is shown at the top of this webpage.
Similar Papers
On the Cross-lingual Transferability of Monolingual Representations
Mikel Artetxe, Sebastian Ruder, Dani Yogatama,
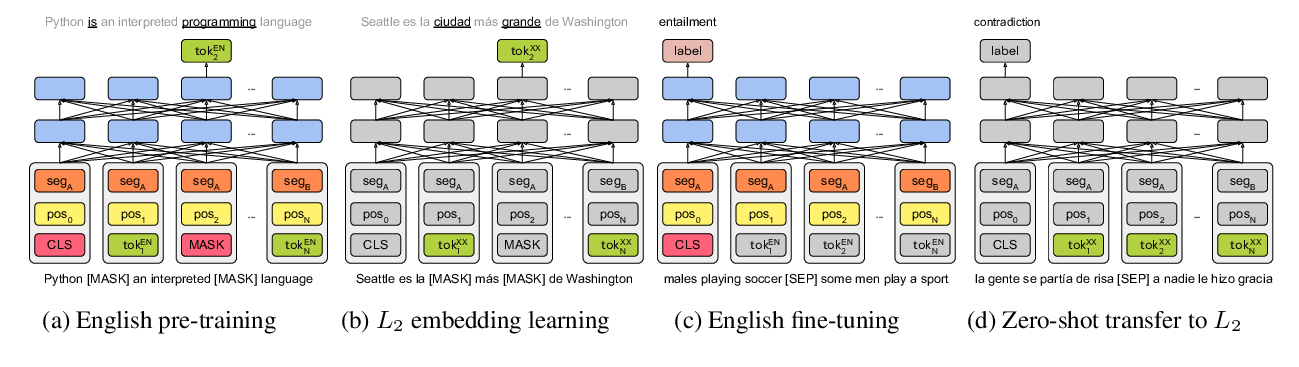
A Call for More Rigor in Unsupervised Cross-lingual Learning
Mikel Artetxe, Sebastian Ruder, Dani Yogatama, Gorka Labaka, Eneko Agirre,
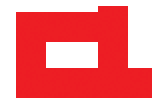
Jointly Learning to Align and Summarize for Neural Cross-Lingual Summarization
Yue Cao, Hui Liu, Xiaojun Wan,
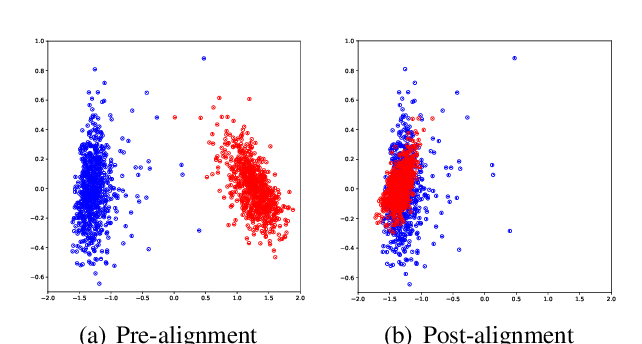
Transferring Monolingual Model to Low-Resource Language: The Case of Tigrinya
Abrhalei Frezghi Tela, Abraham Woubie Zewoudie, Ville Hautamäki,
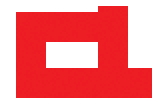