Transferring Monolingual Model to Low-Resource Language: The Case of Tigrinya
Abrhalei Frezghi Tela, Abraham Woubie Zewoudie, Ville Hautamäki
Student Research Workshop SRW Paper
Session 3B: Jul 6
(13:00-14:00 GMT)
Session 12B: Jul 8
(09:00-10:00 GMT)
Abstract:
In recent years, transformer models have achieved great success in natural language processing tasks. Most of the current state-of-the-art NLP results are achieved by using monolingual transformer models, where the model is pre-trained using a single language unlabelled text corpus. Then, the model is fine-tuned to the specific downstream task. However, the cost of pre-training a new transformer model is high for most languages. In this work, we propose a novel transfer learning method to adopt a strong source language model, trained from a large monolingual corpus to a low-resource language. Thus, using XLNet language model, we demonstrate competitive performance with mBERT and a pre-trained target language model on the Cross-lingual Sentiment (CLS) dataset and on a new sentiment analysis dataset for low-resourced language Tigrinya. With only 10k examples of the given Tigrinya sentiment analysis dataset, English XLNet has achieved 78.88% F1-Score outperforming BERT and mBERT by 10% and 7%, respectively. More interestingly, fine-tuning (English) XLNet model on the CLS dataset has promising results compared to mBERT and even outperformed mBERT for one dataset of the Japanese language.
You can open the
pre-recorded video
in a separate window.
NOTE: The SlidesLive video may display a random order of the authors.
The correct author list is shown at the top of this webpage.
Similar Papers
Integrating Multimodal Information in Large Pretrained Transformers
Wasifur Rahman, Md Kamrul Hasan, Sangwu Lee, AmirAli Bagher Zadeh, Chengfeng Mao, Louis-Philippe Morency, Ehsan Hoque,
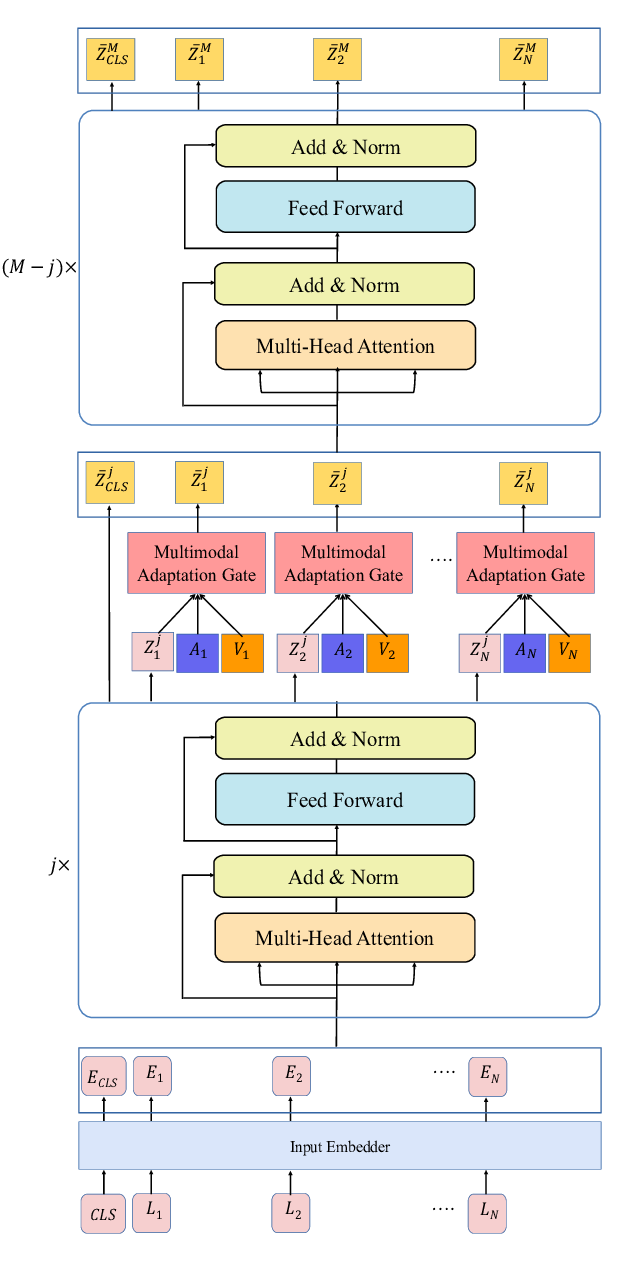
Span Selection Pre-training for Question Answering
Michael Glass, Alfio Gliozzo, Rishav Chakravarti, Anthony Ferritto, Lin Pan, G P Shrivatsa Bhargav, Dinesh Garg, Avi Sil,
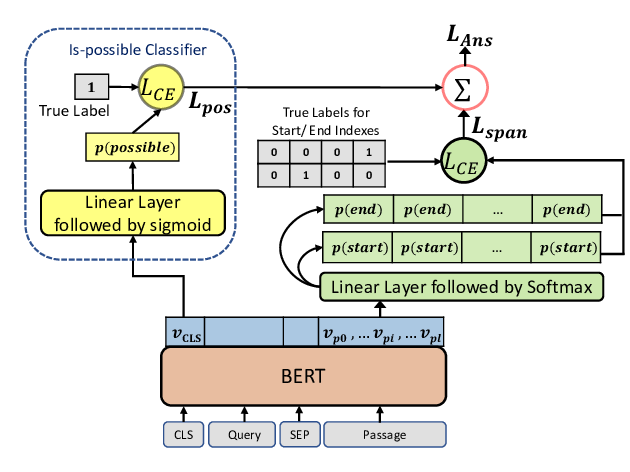
SMART: Robust and Efficient Fine-Tuning for Pre-trained Natural Language Models through Principled Regularized Optimization
Haoming Jiang, Pengcheng He, Weizhu Chen, Xiaodong Liu, Jianfeng Gao, Tuo Zhao,
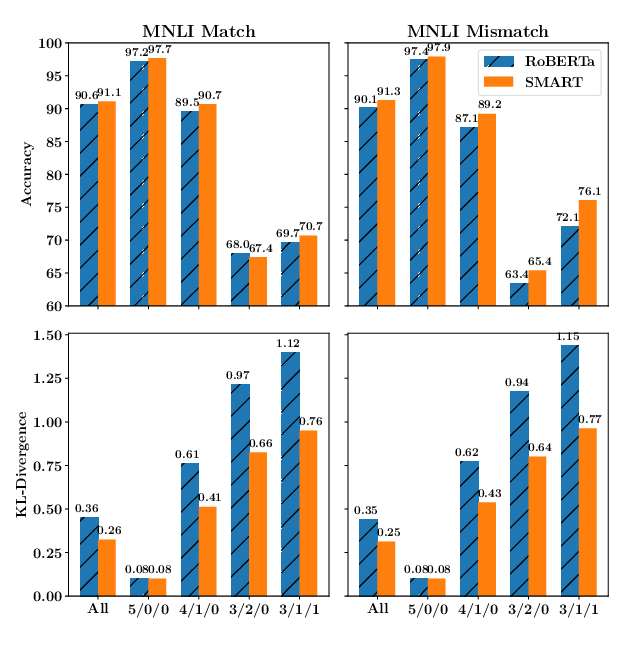
Unsupervised Cross-lingual Representation Learning at Scale
Alexis Conneau, Kartikay Khandelwal, Naman Goyal, Vishrav Chaudhary, Guillaume Wenzek, Francisco Guzmán, Edouard Grave, Myle Ott, Luke Zettlemoyer, Veselin Stoyanov,
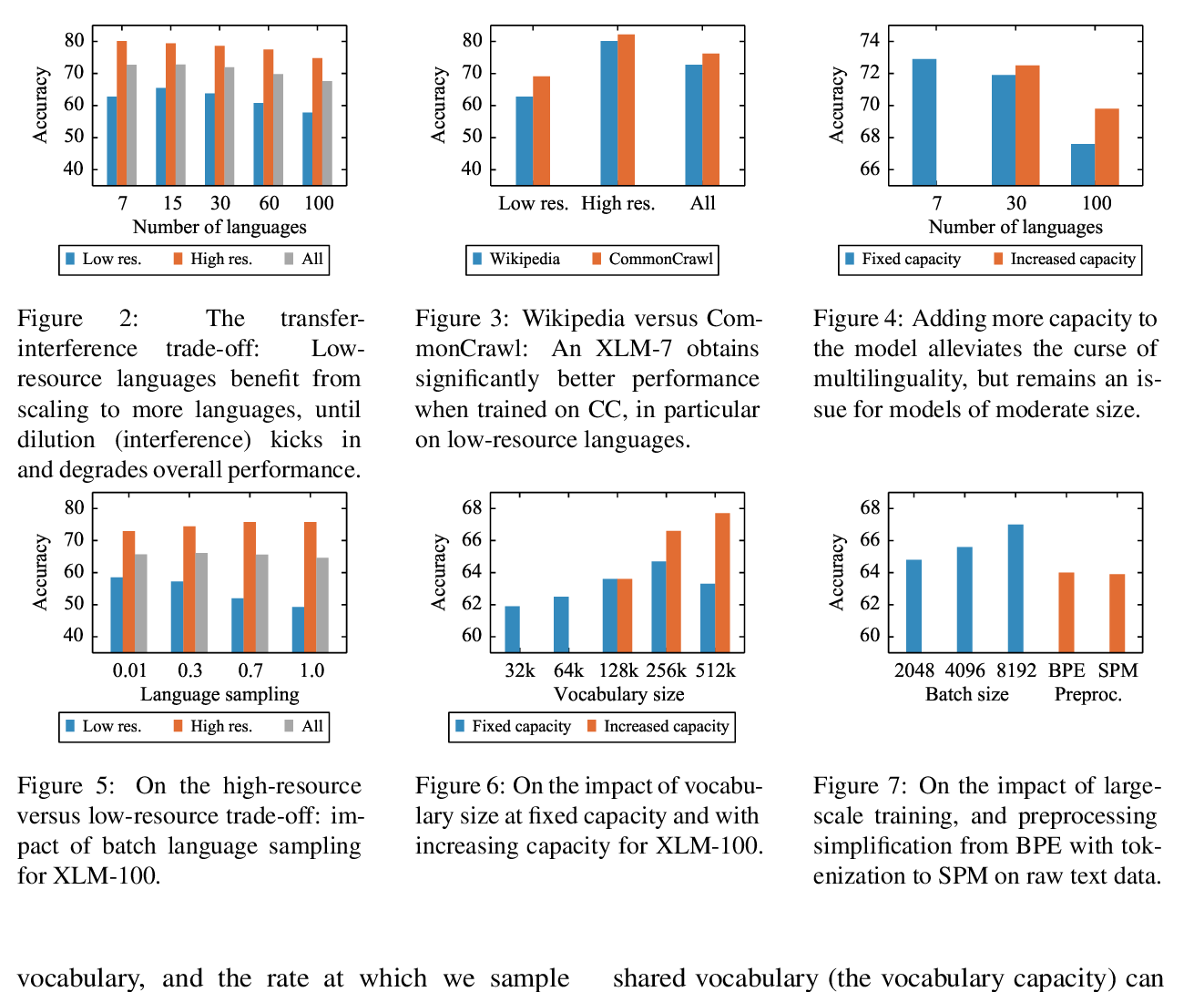