Abstract:
Cross-domain NER is a challenging yet practical problem. Entity mentions can be highly different across domains. However, the correlations between entity types can be relatively more stable across domains. We investigate a multi-cell compositional LSTM structure for multi-task learning, modeling each entity type using a separate cell state. With the help of entity typed units, cross-domain knowledge transfer can be made in an entity type level. Theoretically, the resulting distinct feature distributions for each entity type make it more powerful for cross-domain transfer. Empirically, experiments on four few-shot and zero-shot datasets show our method significantly outperforms a series of multi-task learning methods and achieves the best results.
You can open the
pre-recorded video
in a separate window.
NOTE: The SlidesLive video may display a random order of the authors.
The correct author list is shown at the top of this webpage.
Similar Papers
Zero-Shot Transfer Learning with Synthesized Data for Multi-Domain Dialogue State Tracking
Giovanni Campagna, Agata Foryciarz, Mehrad Moradshahi, Monica Lam,
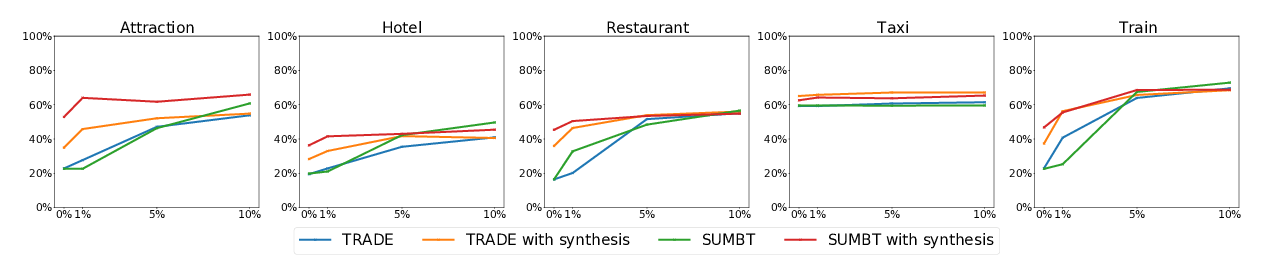
Connecting Embeddings for Knowledge Graph Entity Typing
Yu Zhao, anxiang zhang, Ruobing Xie, Kang Liu, Xiaojie WANG,
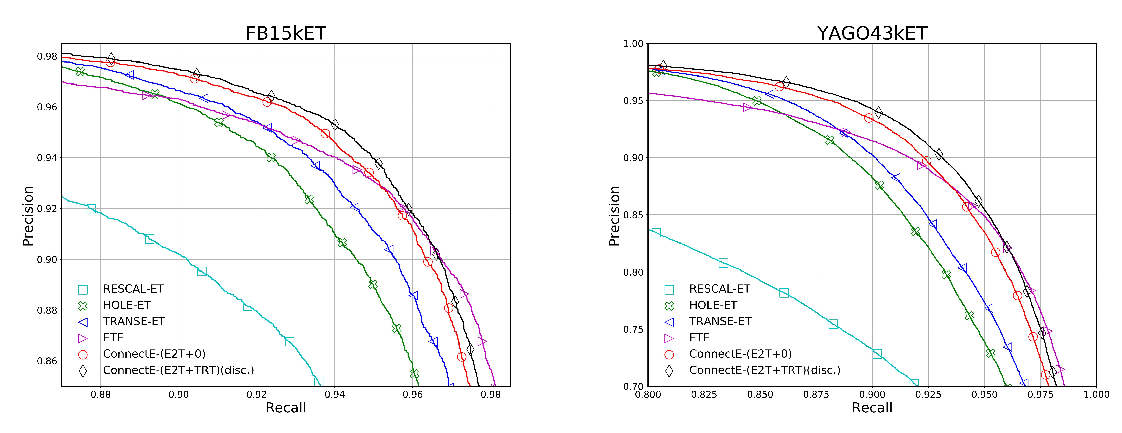
Neighborhood Matching Network for Entity Alignment
Yuting Wu, Xiao Liu, Yansong Feng, Zheng Wang, Dongyan Zhao,
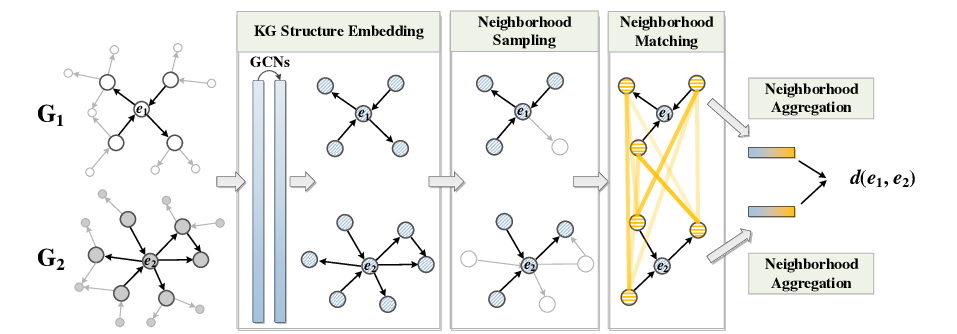
On the Cross-lingual Transferability of Monolingual Representations
Mikel Artetxe, Sebastian Ruder, Dani Yogatama,
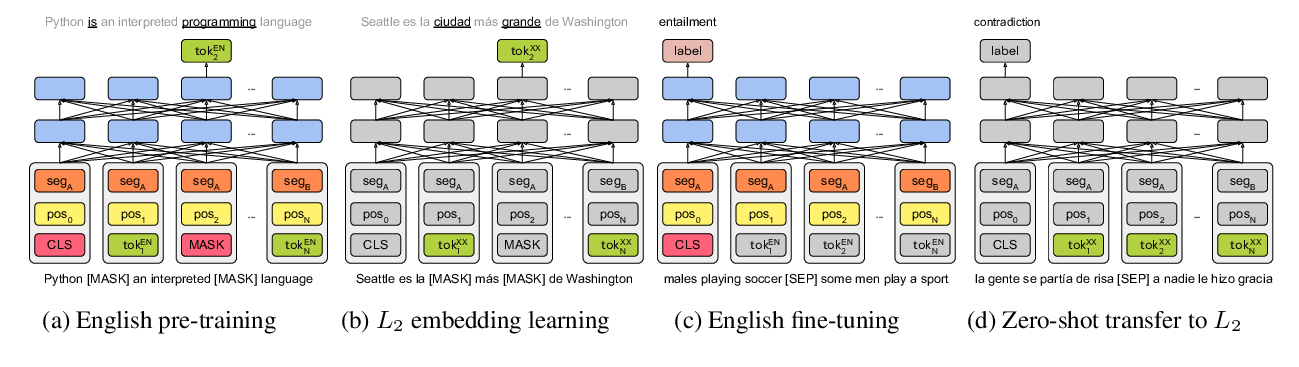