Neighborhood Matching Network for Entity Alignment
Yuting Wu, Xiao Liu, Yansong Feng, Zheng Wang, Dongyan Zhao
Information Extraction Long Paper
Session 11B: Jul 8
(06:00-07:00 GMT)
Session 12A: Jul 8
(08:00-09:00 GMT)
Abstract:
Structural heterogeneity between knowledge graphs is an outstanding challenge for entity alignment. This paper presents Neighborhood Matching Network (NMN), a novel entity alignment framework for tackling the structural heterogeneity challenge. NMN estimates the similarities between entities to capture both the topological structure and the neighborhood difference. It provides two innovative components for better learning representations for entity alignment. It first uses a novel graph sampling method to distill a discriminative neighborhood for each entity. It then adopts a cross-graph neighborhood matching module to jointly encode the neighborhood difference for a given entity pair. Such strategies allow NMN to effectively construct matching-oriented entity representations while ignoring noisy neighbors that have a negative impact on the alignment task. Extensive experiments performed on three entity alignment datasets show that NMN can well estimate the neighborhood similarity in more tough cases and significantly outperforms 12 previous state-of-the-art methods.
You can open the
pre-recorded video
in a separate window.
NOTE: The SlidesLive video may display a random order of the authors.
The correct author list is shown at the top of this webpage.
Similar Papers
Improving Entity Linking through Semantic Reinforced Entity Embeddings
Feng Hou, Ruili Wang, Jun He, Yi Zhou,
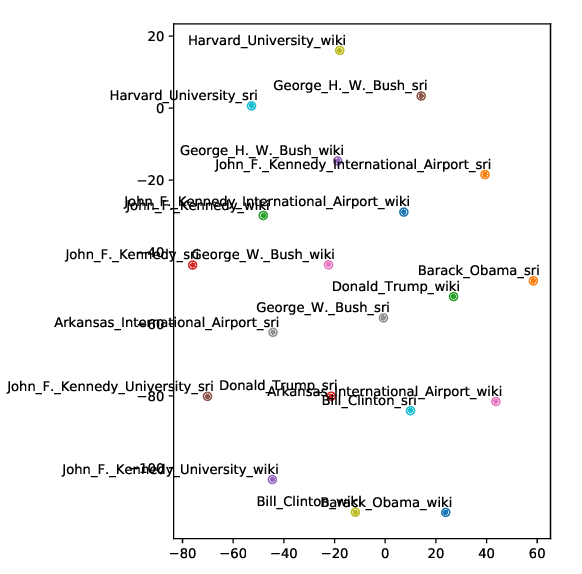
Connecting Embeddings for Knowledge Graph Entity Typing
Yu Zhao, anxiang zhang, Ruobing Xie, Kang Liu, Xiaojie WANG,
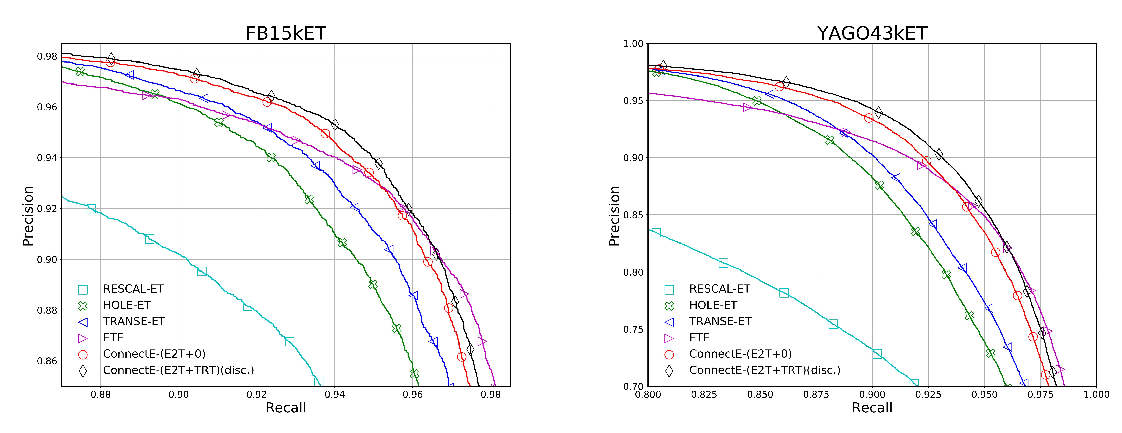
Entity-Aware Dependency-Based Deep Graph Attention Network for Comparative Preference Classification
Nianzu Ma, Sahisnu Mazumder, Hao Wang, Bing Liu,
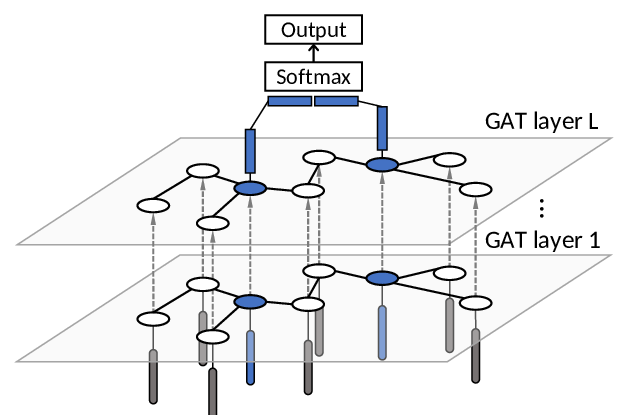