Pyramid: A Layered Model for Nested Named Entity Recognition
Jue Wang, Lidan Shou, Ke Chen, Gang Chen
Information Extraction Long Paper
Session 11A: Jul 8
(05:00-06:00 GMT)
Session 12A: Jul 8
(08:00-09:00 GMT)
Abstract:
This paper presents Pyramid, a novel layered model for Nested Named Entity Recognition (nested NER). In our approach, token or text region embeddings are recursively inputted into L flat NER layers, from bottom to top, stacked in a pyramid shape. Each time an embedding passes through a layer of the pyramid, its length is reduced by one. Its hidden state at layer l represents an l-gram in the input text, which is labeled only if its corresponding text region represents a complete entity mention. We also design an inverse pyramid to allow bidirectional interaction between layers. The proposed method achieves state-of-the-art F1 scores in nested NER on ACE-2004, ACE-2005, GENIA, and NNE, which are 80.27, 79.42, 77.78, and 93.70 with conventional embeddings, and 87.74, 86.34, 79.31, and 94.68 with pre-trained contextualized embeddings. In addition, our model can be used for the more general task of Overlapping Named Entity Recognition. A preliminary experiment confirms the effectiveness of our method in overlapping NER.
You can open the
pre-recorded video
in a separate window.
NOTE: The SlidesLive video may display a random order of the authors.
The correct author list is shown at the top of this webpage.
Similar Papers
A Unified MRC Framework for Named Entity Recognition
Xiaoya Li, Jingrong Feng, Yuxian Meng, Qinghong Han, Fei Wu, Jiwei Li,
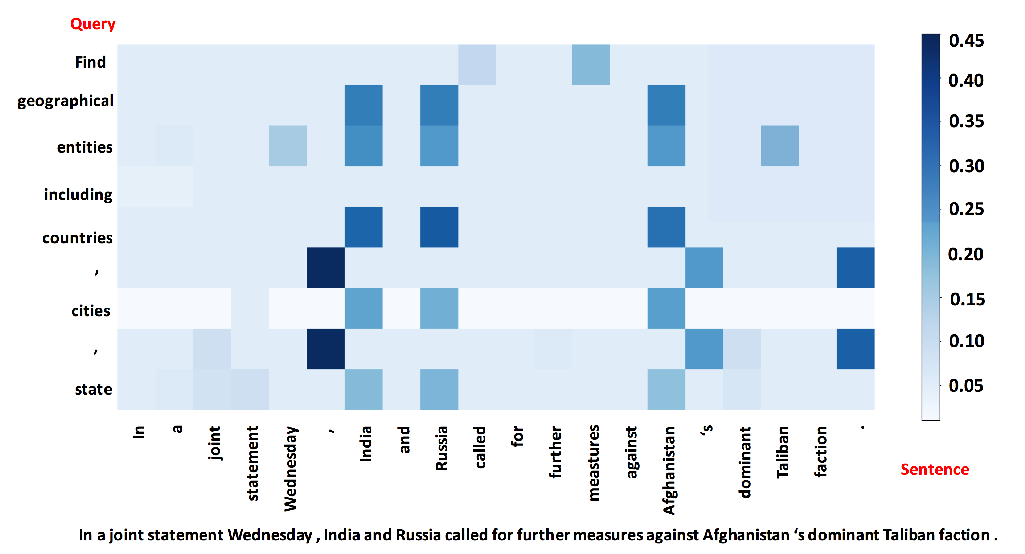
Simplify the Usage of Lexicon in Chinese NER
Ruotian Ma, Minlong Peng, Qi Zhang, Zhongyu Wei, Xuanjing Huang,
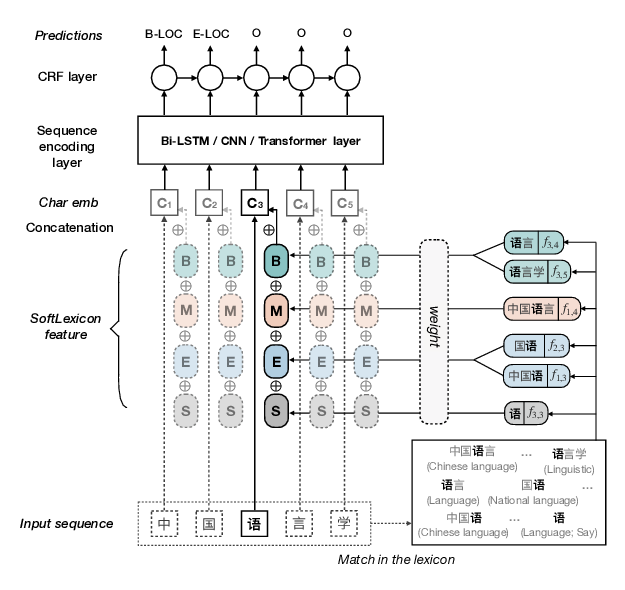
Improving Entity Linking through Semantic Reinforced Entity Embeddings
Feng Hou, Ruili Wang, Jun He, Yi Zhou,
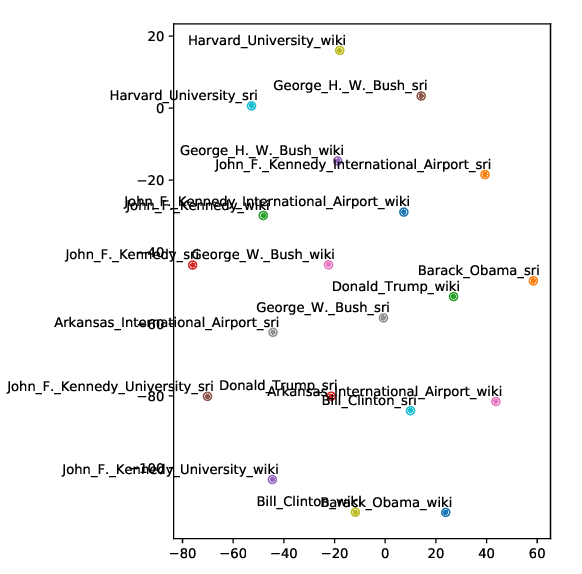