Improving Neural Machine Translation with Soft Template Prediction
Jian Yang, Shuming Ma, Dongdong Zhang, Zhoujun Li, Ming Zhou
Machine Translation Long Paper
Session 11A: Jul 8
(05:00-06:00 GMT)
Session 12A: Jul 8
(08:00-09:00 GMT)
Abstract:
Although neural machine translation (NMT) has achieved significant progress in recent years, most previous NMT models only depend on the source text to generate translation. Inspired by the success of template-based and syntax-based approaches in other fields, we propose to use extracted templates from tree structures as soft target templates to guide the translation procedure. In order to learn the syntactic structure of the target sentences, we adopt constituency-based parse tree to generate candidate templates. We incorporate the template information into the encoder-decoder framework to jointly utilize the templates and source text. Experiments show that our model significantly outperforms the baseline models on four benchmarks and demonstrates the effectiveness of soft target templates.
You can open the
pre-recorded video
in a separate window.
NOTE: The SlidesLive video may display a random order of the authors.
The correct author list is shown at the top of this webpage.
Similar Papers
Regularized Context Gates on Transformer for Machine Translation
Xintong Li, Lemao Liu, Rui Wang, Guoping Huang, Max Meng,
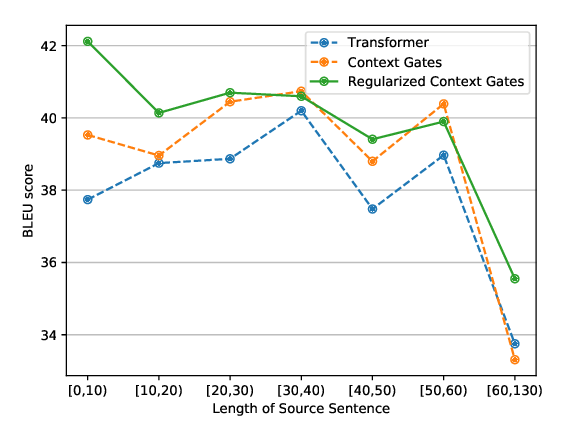
Multi-Task Neural Model for Agglutinative Language Translation
Yirong Pan, Xiao Li, Yating Yang, Rui Dong,
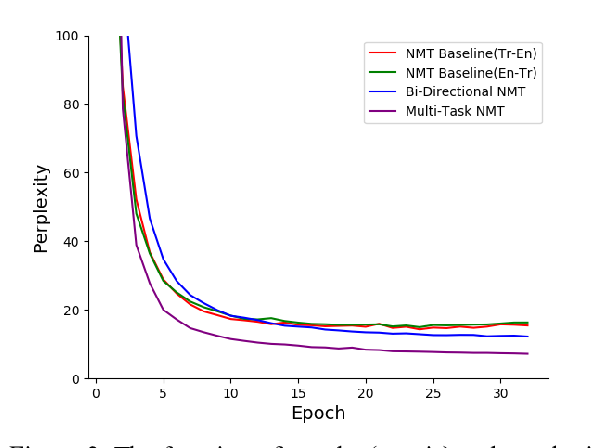
Neural Data-to-Text Generation via Jointly Learning the Segmentation and Correspondence
Xiaoyu Shen, Ernie Chang, Hui Su, Cheng Niu, Dietrich Klakow,
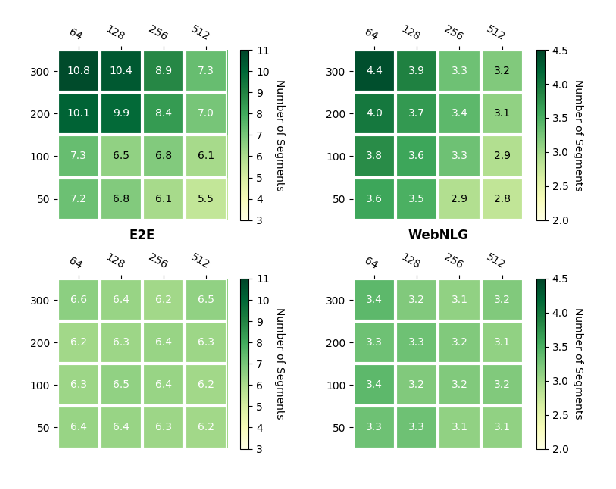