Multi-Task Neural Model for Agglutinative Language Translation
Yirong Pan, Xiao Li, Yating Yang, Rui Dong
Student Research Workshop SRW Paper
Session 2B: Jul 6
(09:00-10:00 GMT)
Session 11B: Jul 8
(06:00-07:00 GMT)
Abstract:
Neural machine translation (NMT) has achieved impressive performance recently by using large-scale parallel corpora. However, it struggles in the low-resource and morphologically-rich scenarios of agglutinative language translation task. Inspired by the finding that monolingual data can greatly improve the NMT performance, we propose a multi-task neural model that jointly learns to perform bi-directional translation and agglutinative language stemming. Our approach employs the shared encoder and decoder to train a single model without changing the standard NMT architecture but instead adding a token before each source-side sentence to specify the desired target outputs of the two different tasks. Experimental results on Turkish-English and Uyghur-Chinese show that our proposed approach can significantly improve the translation performance on agglutinative languages by using a small amount of monolingual data.
You can open the
pre-recorded video
in a separate window.
NOTE: The SlidesLive video may display a random order of the authors.
The correct author list is shown at the top of this webpage.
Similar Papers
Leveraging Monolingual Data with Self-Supervision for Multilingual Neural Machine Translation
Aditya Siddhant, Ankur Bapna, Yuan Cao, Orhan Firat, Mia Chen, Sneha Kudugunta, Naveen Arivazhagan, Yonghui Wu,
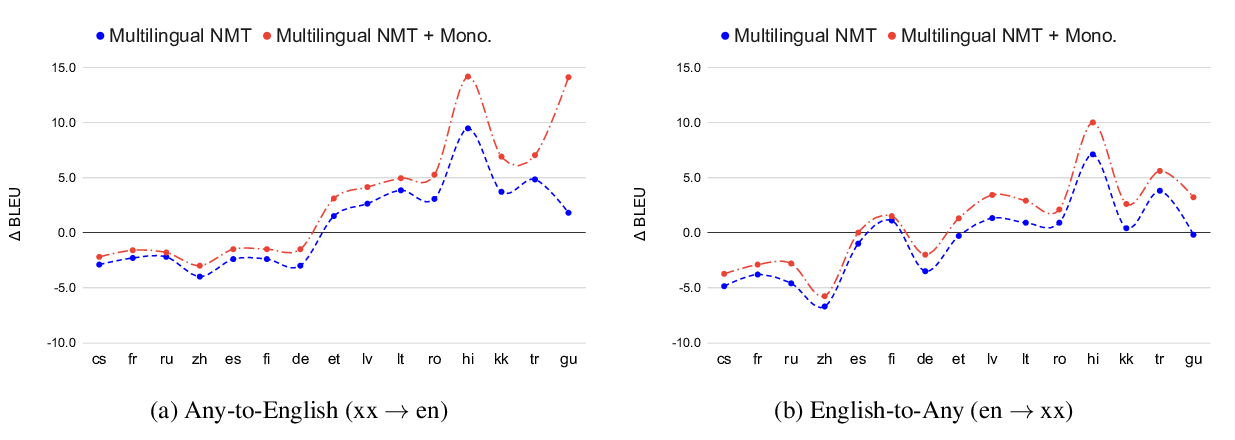
Tagged Back-translation Revisited: Why Does It Really Work?
Benjamin Marie, Raphael Rubino, Atsushi Fujita,
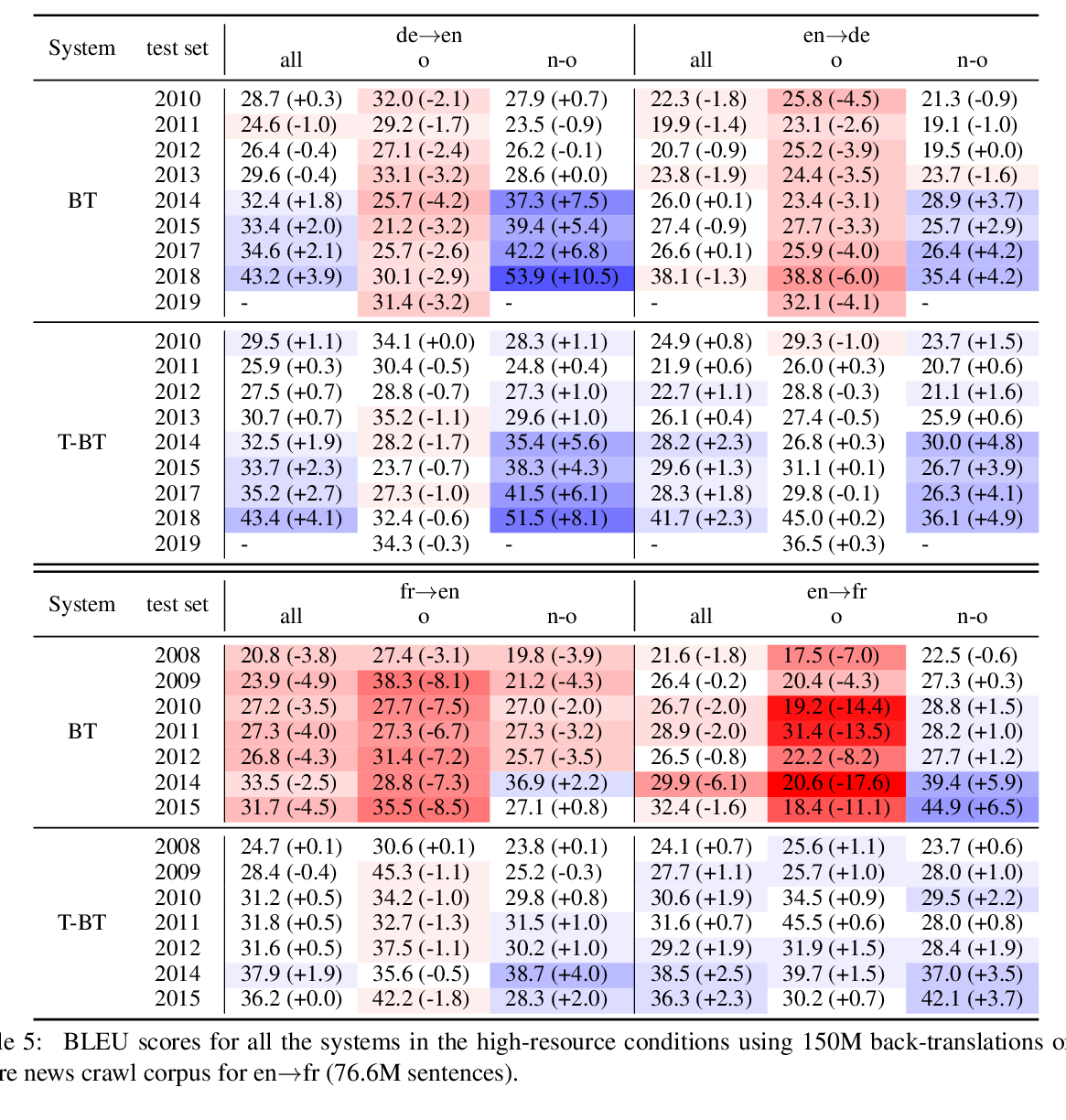
Multiscale Collaborative Deep Models for Neural Machine Translation
Xiangpeng Wei, Heng Yu, Yue Hu, Yue Zhang, Rongxiang Weng, Weihua Luo,
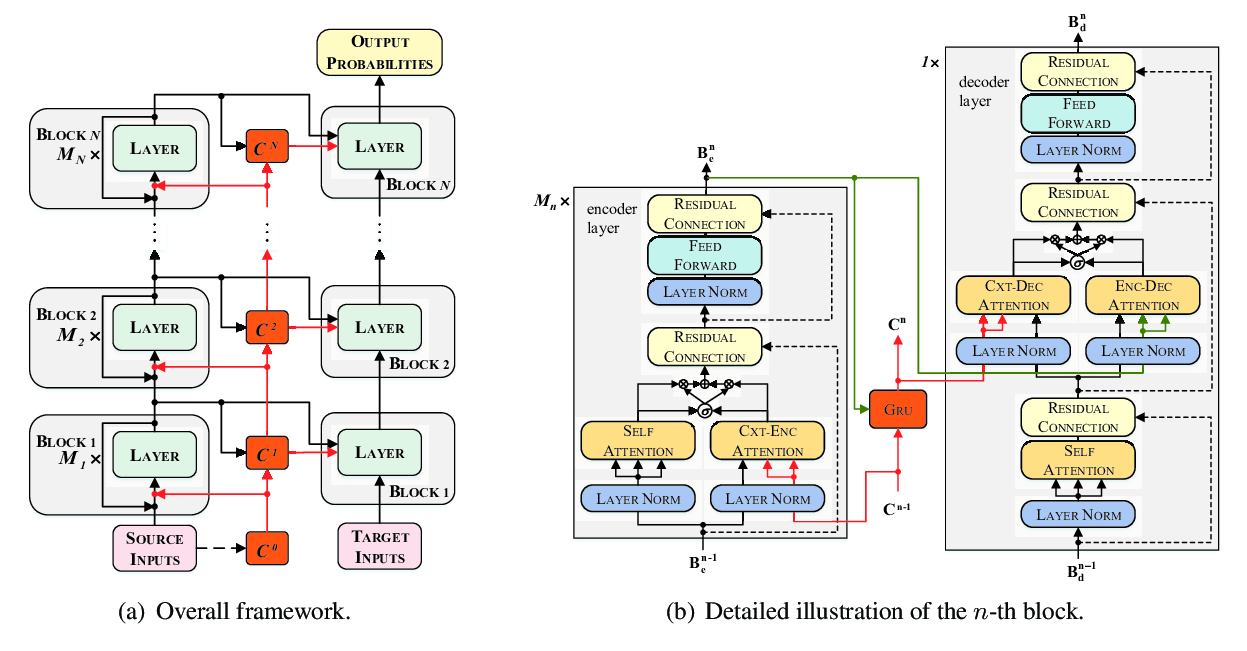