Reasoning Over Semantic-Level Graph for Fact Checking
Wanjun Zhong, Jingjing Xu, Duyu Tang, Zenan Xu, Nan Duan, Ming Zhou, Jiahai Wang, Jian Yin
Semantics: Textual Inference and Other Areas of Semantics Long Paper
Session 11A: Jul 8
(05:00-06:00 GMT)
Session 13A: Jul 8
(12:00-13:00 GMT)
Abstract:
Fact checking is a challenging task because verifying the truthfulness of a claim requires reasoning about multiple retrievable evidence. In this work, we present a method suitable for reasoning about the semantic-level structure of evidence. Unlike most previous works, which typically represent evidence sentences with either string concatenation or fusing the features of isolated evidence sentences, our approach operates on rich semantic structures of evidence obtained by semantic role labeling. We propose two mechanisms to exploit the structure of evidence while leveraging the advances of pre-trained models like BERT, GPT or XLNet. Specifically, using XLNet as the backbone, we first utilize the graph structure to re-define the relative distances of words, with the intuition that semantically related words should have short distances. Then, we adopt graph convolutional network and graph attention network to propagate and aggregate information from neighboring nodes on the graph. We evaluate our system on FEVER, a benchmark dataset for fact checking, and find that rich structural information is helpful and both our graph-based mechanisms improve the accuracy. Our model is the state-of-the-art system in terms of both official evaluation metrics, namely claim verification accuracy and FEVER score.
You can open the
pre-recorded video
in a separate window.
NOTE: The SlidesLive video may display a random order of the authors.
The correct author list is shown at the top of this webpage.
Similar Papers
Fine-grained Fact Verification with Kernel Graph Attention Network
Zhenghao Liu, Chenyan Xiong, Maosong Sun, Zhiyuan Liu,
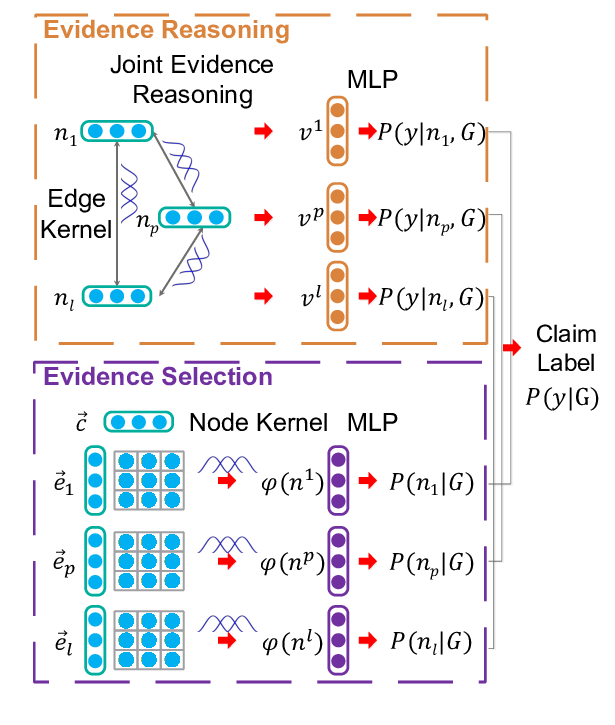
DeSePtion: Dual Sequence Prediction and Adversarial Examples for Improved Fact-Checking
Christopher Hidey, Tuhin Chakrabarty, Tariq Alhindi, Siddharth Varia, Kriste Krstovski, Mona Diab, Smaranda Muresan,
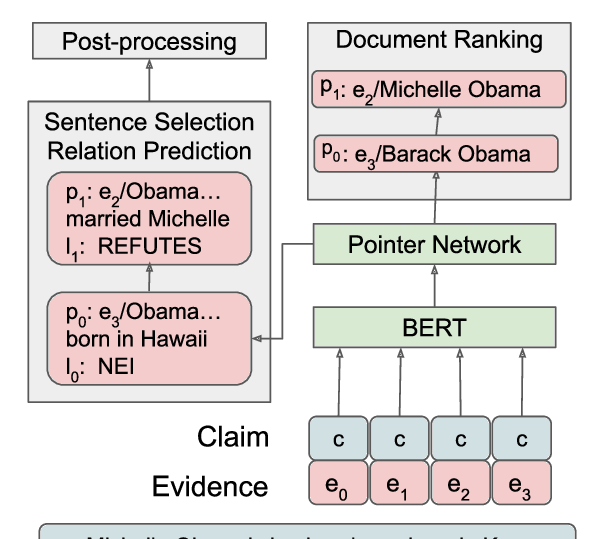
DTCA: Decision Tree-based Co-Attention Networks for Explainable Claim Verification
Lianwei Wu, Yuan Rao, Yongqiang Zhao, Hao Liang, Ambreen Nazir,
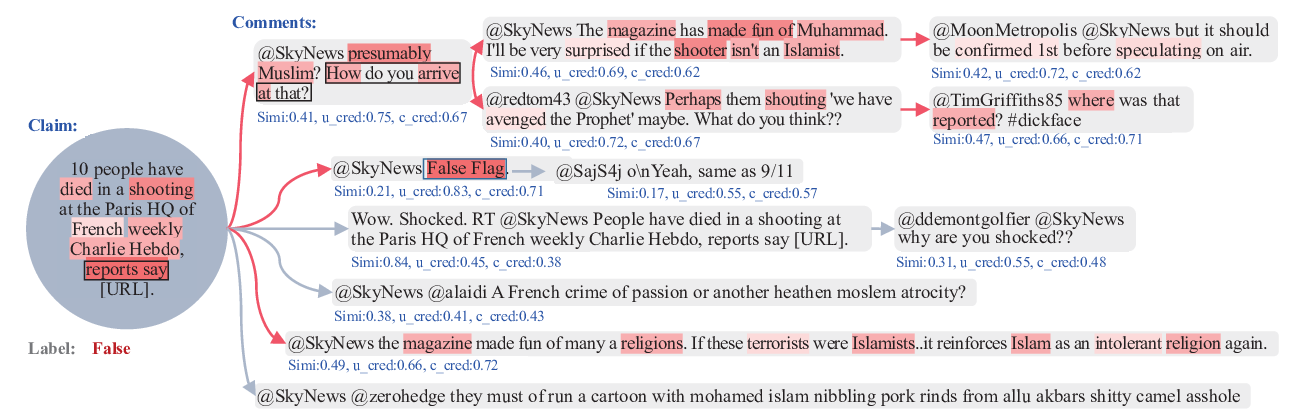
LogicalFactChecker: Leveraging Logical Operations for Fact Checking with Graph Module Network
Wanjun Zhong, Duyu Tang, Zhangyin Feng, Nan Duan, Ming Zhou, Ming Gong, Linjun Shou, Daxin Jiang, Jiahai Wang, Jian Yin,
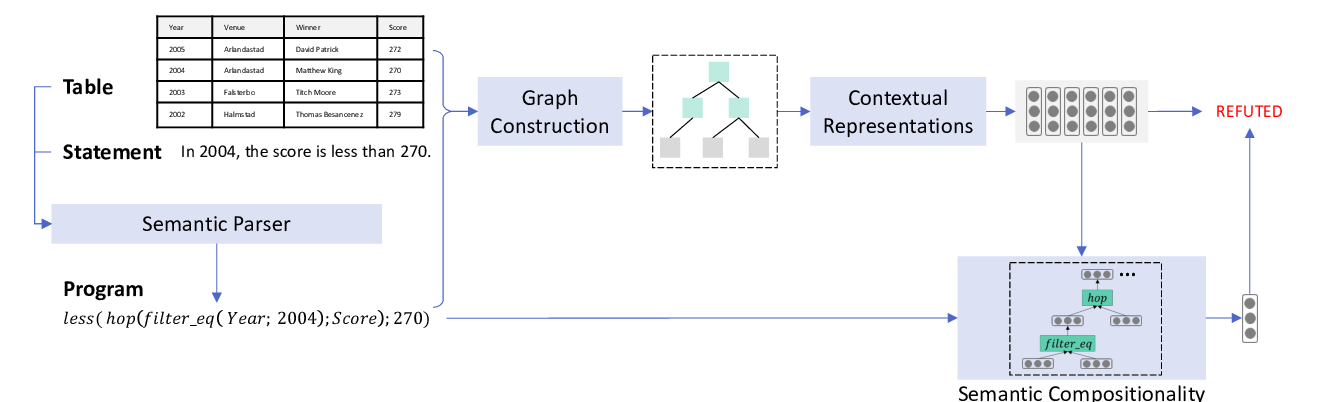