Fine-grained Fact Verification with Kernel Graph Attention Network
Zhenghao Liu, Chenyan Xiong, Maosong Sun, Zhiyuan Liu
Semantics: Textual Inference and Other Areas of Semantics Long Paper
Session 12B: Jul 8
(09:00-10:00 GMT)
Session 13A: Jul 8
(12:00-13:00 GMT)
Abstract:
Fact Verification requires fine-grained natural language inference capability that finds subtle clues to identify the syntactical and semantically correct but not well-supported claims. This paper presents Kernel Graph Attention Network (KGAT), which conducts more fine-grained fact verification with kernel-based attentions. Given a claim and a set of potential evidence sentences that form an evidence graph, KGAT introduces node kernels, which better measure the importance of the evidence node, and edge kernels, which conduct fine-grained evidence propagation in the graph, into Graph Attention Networks for more accurate fact verification. KGAT achieves a 70.38% FEVER score and significantly outperforms existing fact verification models on FEVER, a large-scale benchmark for fact verification. Our analyses illustrate that, compared to dot-product attentions, the kernel-based attention concentrates more on relevant evidence sentences and meaningful clues in the evidence graph, which is the main source of KGAT's effectiveness. All source codes of this work are available at https://github.com/thunlp/KernelGAT.
You can open the
pre-recorded video
in a separate window.
NOTE: The SlidesLive video may display a random order of the authors.
The correct author list is shown at the top of this webpage.
Similar Papers
Reasoning Over Semantic-Level Graph for Fact Checking
Wanjun Zhong, Jingjing Xu, Duyu Tang, Zenan Xu, Nan Duan, Ming Zhou, Jiahai Wang, Jian Yin,
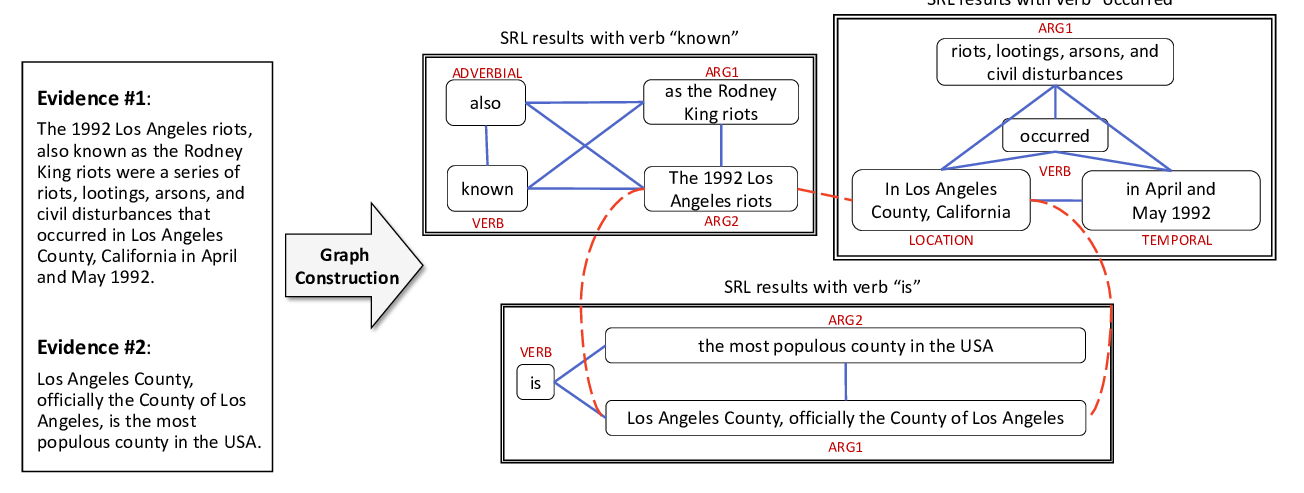
DeSePtion: Dual Sequence Prediction and Adversarial Examples for Improved Fact-Checking
Christopher Hidey, Tuhin Chakrabarty, Tariq Alhindi, Siddharth Varia, Kriste Krstovski, Mona Diab, Smaranda Muresan,
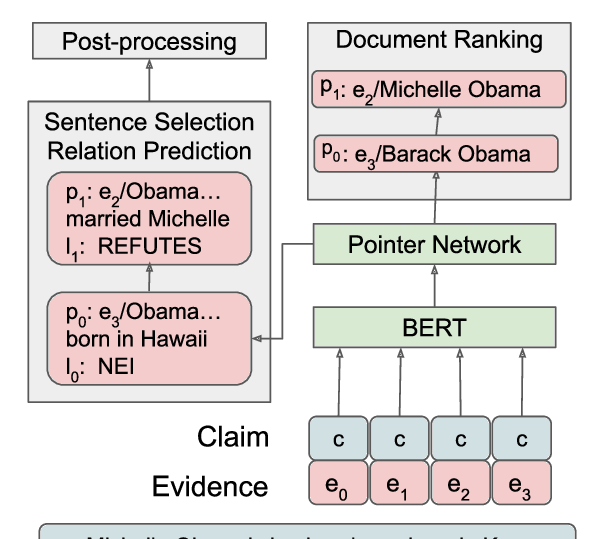
DTCA: Decision Tree-based Co-Attention Networks for Explainable Claim Verification
Lianwei Wu, Yuan Rao, Yongqiang Zhao, Hao Liang, Ambreen Nazir,
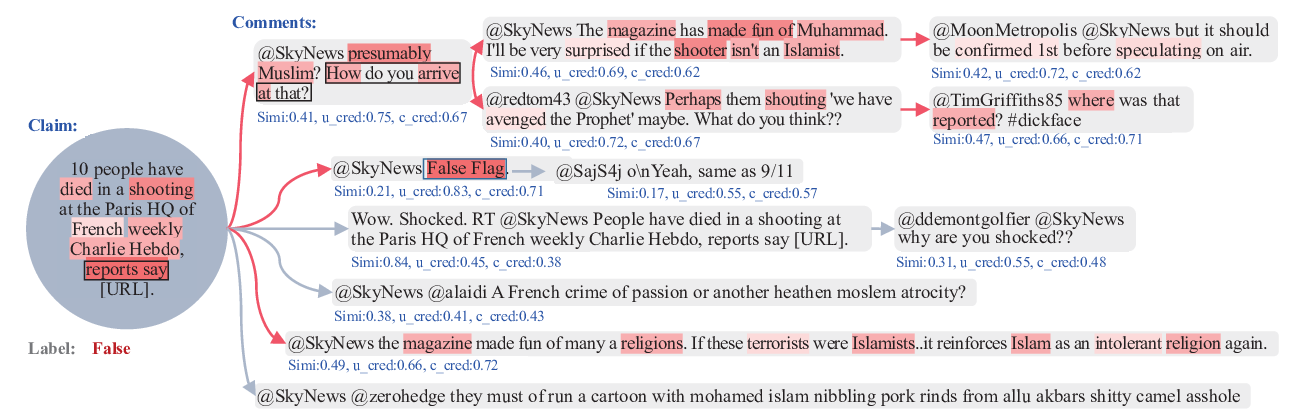
Connecting Embeddings for Knowledge Graph Entity Typing
Yu Zhao, anxiang zhang, Ruobing Xie, Kang Liu, Xiaojie WANG,
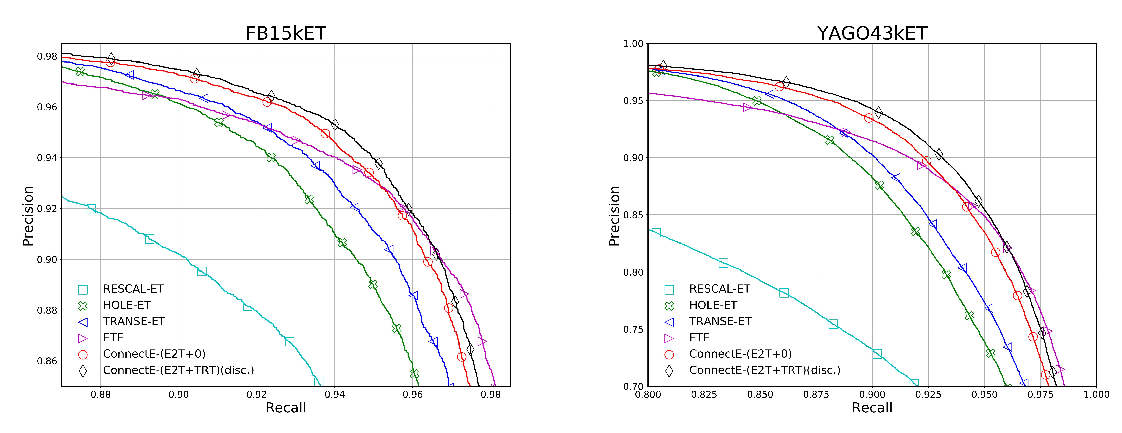