Learning Efficient Dialogue Policy from Demonstrations through Shaping
Huimin Wang, Baolin Peng, Kam-Fai Wong
Dialogue and Interactive Systems Long Paper
Session 11B: Jul 8
(06:00-07:00 GMT)
Session 12B: Jul 8
(09:00-10:00 GMT)
Abstract:
Training a task-oriented dialogue agent with reinforcement learning is prohibitively expensive since it requires a large volume of interactions with users. Human demonstrations can be used to accelerate learning progress. However, how to effectively leverage demonstrations to learn dialogue policy remains less explored. In this paper, we present S^2Agent that efficiently learns dialogue policy from demonstrations through policy shaping and reward shaping. We use an imitation model to distill knowledge from demonstrations, based on which policy shaping estimates feedback on how the agent should act in policy space. Reward shaping is then incorporated to bonus state-actions similar to demonstrations explicitly in value space encouraging better exploration. The effectiveness of the proposed S^2Agentt is demonstrated in three dialogue domains and a challenging domain adaptation task with both user simulator evaluation and human evaluation.
You can open the
pre-recorded video
in a separate window.
NOTE: The SlidesLive video may display a random order of the authors.
The correct author list is shown at the top of this webpage.
Similar Papers
Semi-Supervised Dialogue Policy Learning via Stochastic Reward Estimation
Xinting Huang, Jianzhong Qi, Yu Sun, Rui Zhang,
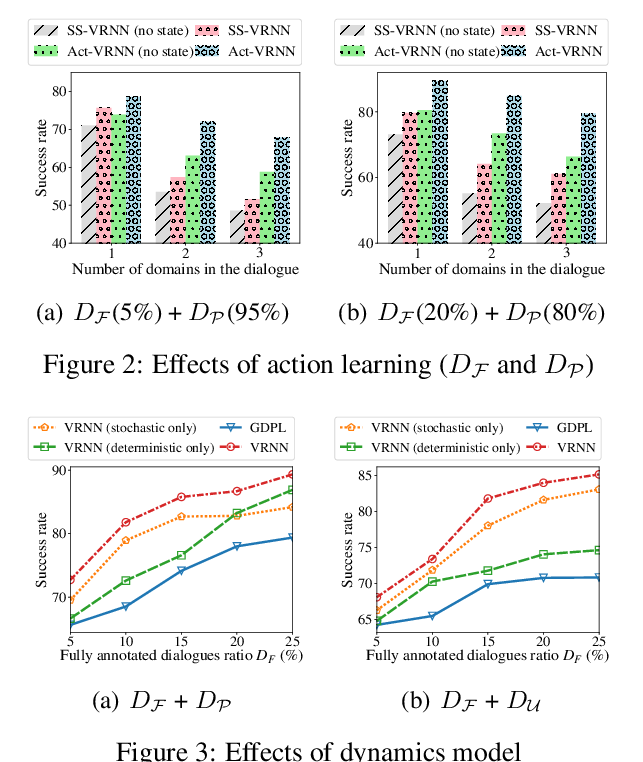
Multi-Agent Task-Oriented Dialog Policy Learning with Role-Aware Reward Decomposition
Ryuichi Takanobu, Runze Liang, Minlie Huang,
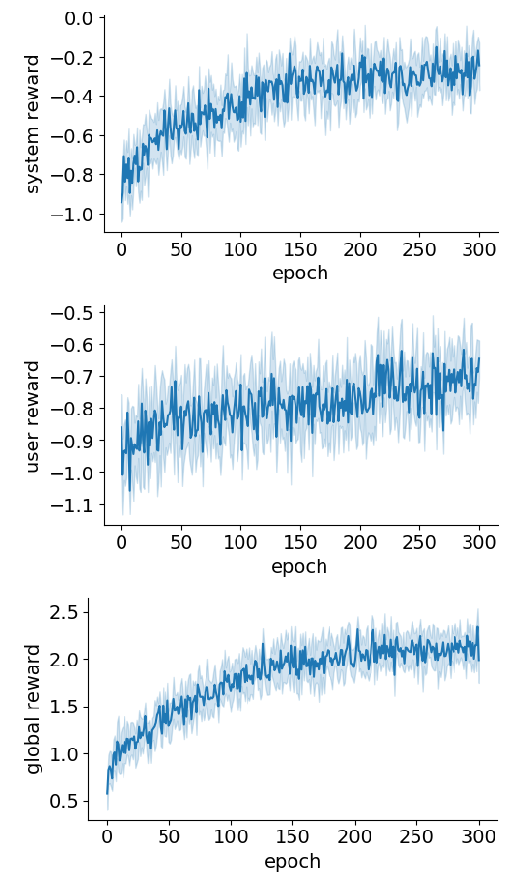
Conversational Graph Grounded Policy Learning for Open-Domain Conversation Generation
Jun Xu, Haifeng Wang, Zheng-Yu Niu, Hua Wu, Wanxiang Che, Ting Liu,
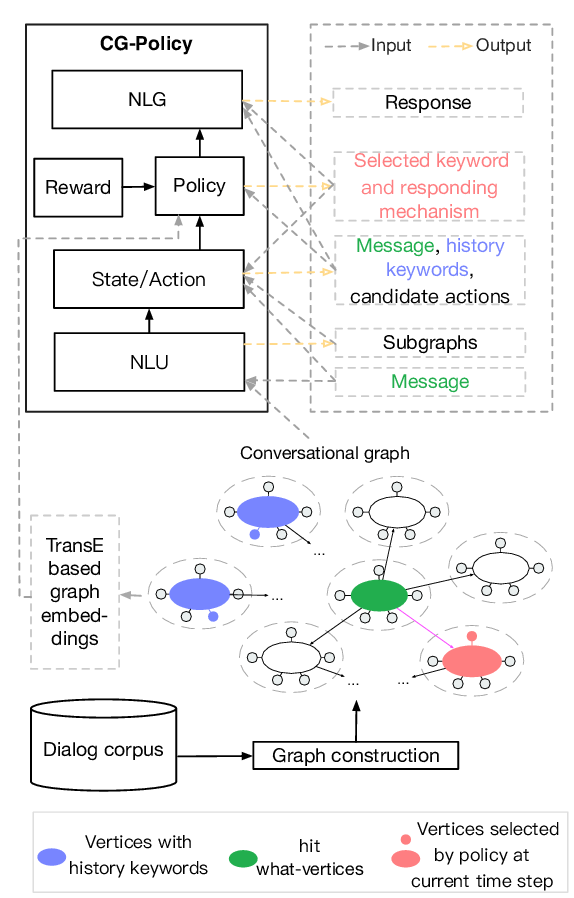
CrossWOZ: A Large-Scale Chinese Cross-Domain Task-Oriented Dialogue Dataset
Qi Zhu, Kaili Huang, Zheng Zhang, Xiaoyan Zhu, Minlie Huang,
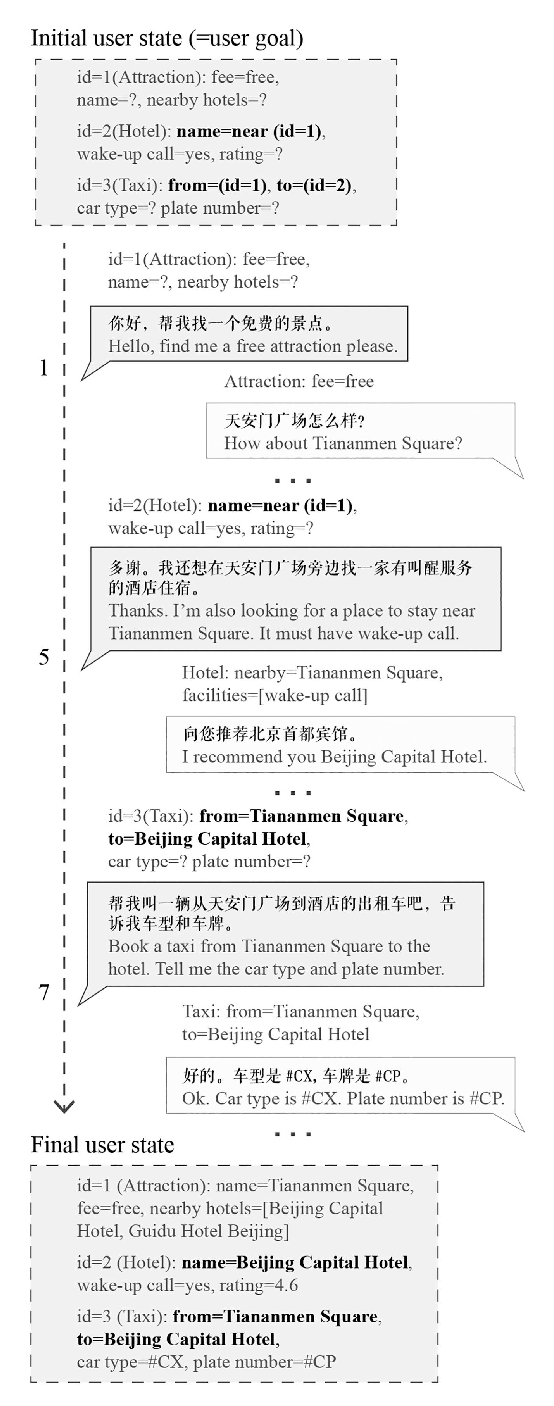