GAN-BERT: Generative Adversarial Learning for Robust Text Classification with a Bunch of Labeled Examples
Danilo Croce, Giuseppe Castellucci, Roberto Basili
Machine Learning for NLP Short Paper
Session 4A: Jul 6
(17:00-18:00 GMT)
Session 5A: Jul 6
(20:00-21:00 GMT)
Abstract:
Recent Transformer-based architectures, e.g., BERT, provide impressive results in many Natural Language Processing tasks. However, most of the adopted benchmarks are made of (sometimes hundreds of) thousands of examples. In many real scenarios, obtaining high- quality annotated data is expensive and time consuming; in contrast, unlabeled examples characterizing the target task can be, in general, easily collected. One promising method to enable semi-supervised learning has been proposed in image processing, based on Semi- Supervised Generative Adversarial Networks. In this paper, we propose GAN-BERT that ex- tends the fine-tuning of BERT-like architectures with unlabeled data in a generative adversarial setting. Experimental results show that the requirement for annotated examples can be drastically reduced (up to only 50-100 annotated examples), still obtaining good performances in several sentence classification tasks.
You can open the
pre-recorded video
in a separate window.
NOTE: The SlidesLive video may display a random order of the authors.
The correct author list is shown at the top of this webpage.
Similar Papers
A Geometry-Inspired Attack for Generating Natural Language Adversarial Examples
Zhao Meng, Roger Wattenhofer,
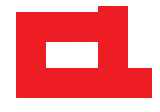
It’s Morphin’ Time! Combating Linguistic Discrimination with Inflectional Perturbations
Samson Tan, Shafiq Joty, Min-Yen Kan, Richard Socher,
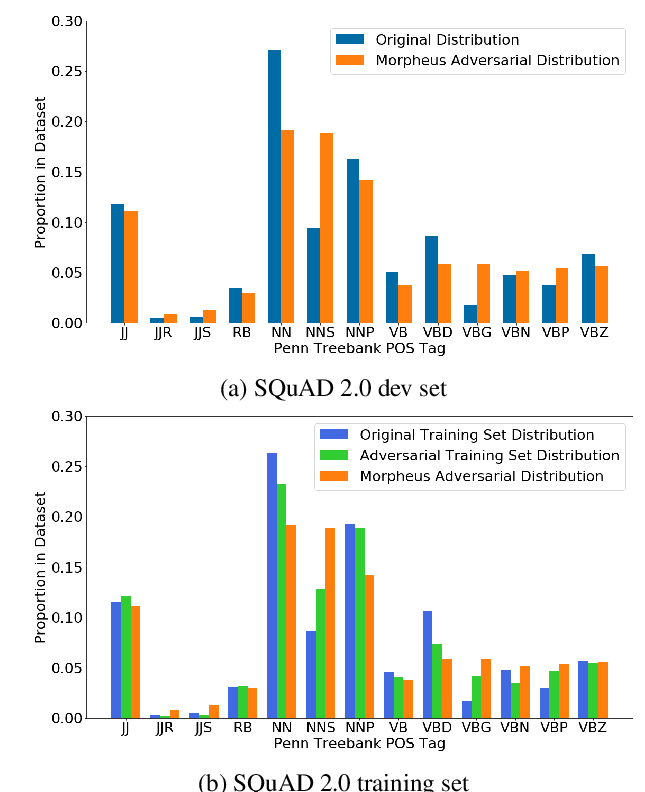
Evaluating and Enhancing the Robustness of Neural Network-based Dependency Parsing Models with Adversarial Examples
Xiaoqing Zheng, Jiehang Zeng, Yi Zhou, Cho-Jui Hsieh, Minhao Cheng, Xuanjing Huang,
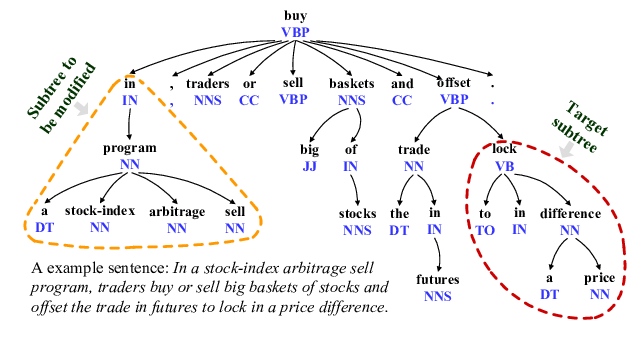
To Pretrain or Not to Pretrain: Examining the Benefits of Pretrainng on Resource Rich Tasks
Sinong Wang, Madian Khabsa, Hao Ma,
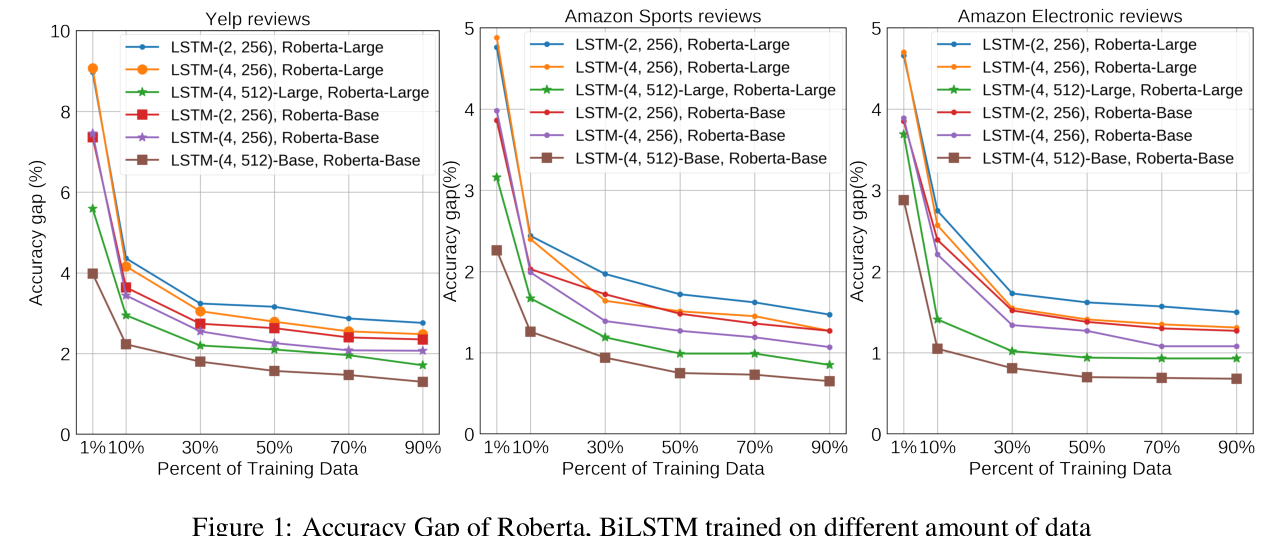