Gender in Danger? Evaluating Speech Translation Technology on the MuST-SHE Corpus
Luisa Bentivogli, Beatrice Savoldi, Matteo Negri, Mattia A. Di Gangi, Roldano Cattoni, Marco Turchi
Machine Translation Long Paper
Session 12A: Jul 8
(08:00-09:00 GMT)
Session 14B: Jul 8
(18:00-19:00 GMT)
Abstract:
Translating from languages without productive grammatical gender like English into gender-marked languages is a well-known difficulty for machines. This difficulty is also due to the fact that the training data on which models are built typically reflect the asymmetries of natural languages, gender bias included. Exclusively fed with textual data, machine translation is intrinsically constrained by the fact that the input sentence does not always contain clues about the gender identity of the referred human entities. But what happens with speech translation, where the input is an audio signal? Can audio provide additional information to reduce gender bias? We present the first thorough investigation of gender bias in speech translation, contributing with: i) the release of a benchmark useful for future studies, and ii) the comparison of different technologies (cascade and end-to-end) on two language directions (English-Italian/French).
You can open the
pre-recorded video
in a separate window.
NOTE: The SlidesLive video may display a random order of the authors.
The correct author list is shown at the top of this webpage.
Similar Papers
Reducing Gender Bias in Neural Machine Translation as a Domain Adaptation Problem
Danielle Saunders, Bill Byrne,
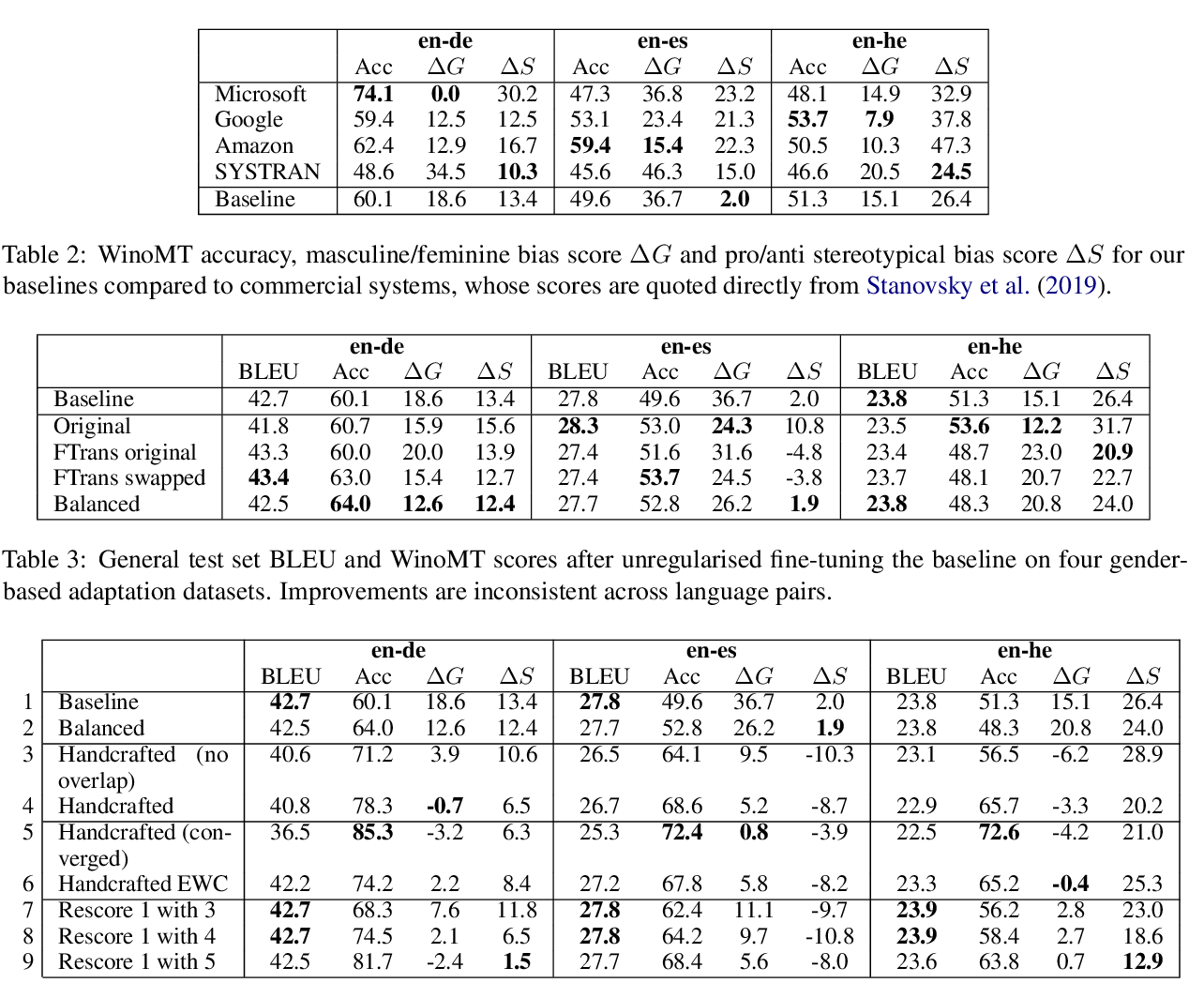
Double-Hard Debias: Tailoring Word Embeddings for Gender Bias Mitigation
Tianlu Wang, Xi Victoria Lin, Nazneen Fatema Rajani, Bryan McCann, Vicente Ordonez, Caiming Xiong,
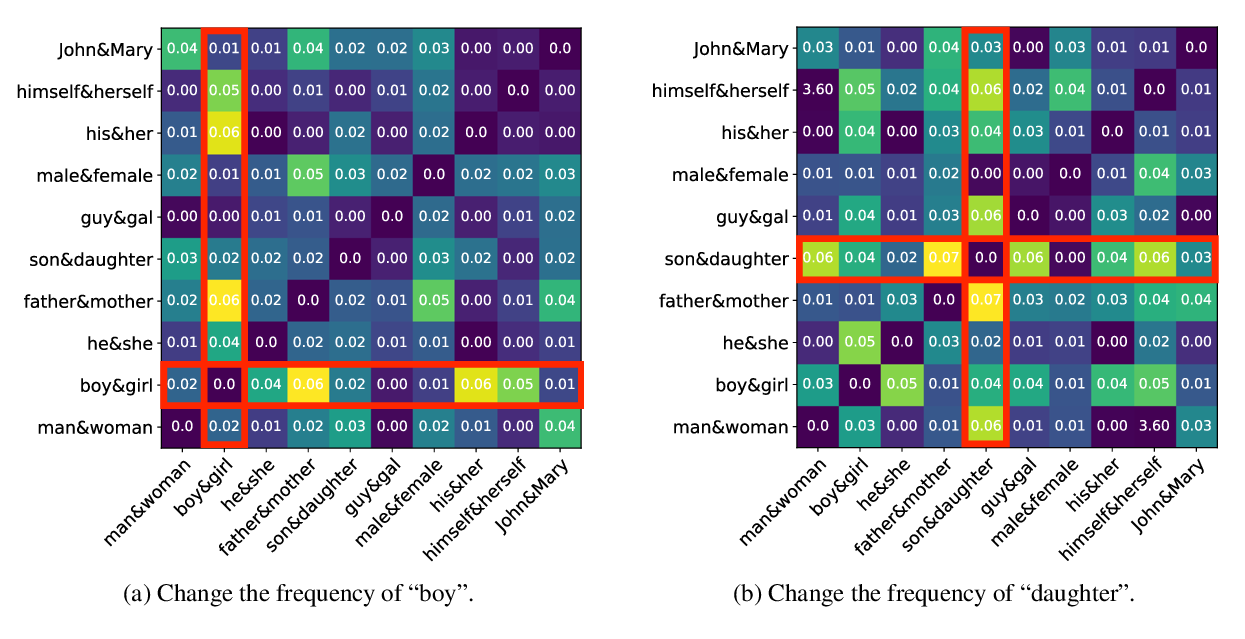
Predicting Declension Class from Form and Meaning
Adina Williams, Tiago Pimentel, Hagen Blix, Arya D. McCarthy, Eleanor Chodroff, Ryan Cotterell,
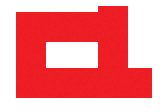