Reducing Gender Bias in Neural Machine Translation as a Domain Adaptation Problem
Danielle Saunders, Bill Byrne
Machine Translation Long Paper
Session 13B: Jul 8
(13:00-14:00 GMT)
Session 14B: Jul 8
(18:00-19:00 GMT)
Abstract:
Training data for NLP tasks often exhibits gender bias in that fewer sentences refer to women than to men. In Neural Machine Translation (NMT) gender bias has been shown to reduce translation quality, particularly when the target language has grammatical gender. The recent WinoMT challenge set allows us to measure this effect directly (Stanovsky et al, 2019) Ideally we would reduce system bias by simply debiasing all data prior to training, but achieving this effectively is itself a challenge. Rather than attempt to create a `balanced' dataset, we use transfer learning on a small set of trusted, gender-balanced examples. This approach gives strong and consistent improvements in gender debiasing with much less computational cost than training from scratch. A known pitfall of transfer learning on new domains is `catastrophic forgetting', which we address at adaptation and inference time. During adaptation we show that Elastic Weight Consolidation allows a performance trade-off between general translation quality and bias reduction. At inference time we propose a lattice-rescoring scheme which outperforms all systems evaluated in Stanovsky et al, 2019 on WinoMT with no degradation of general test set BLEU. We demonstrate our approach translating from English into three languages with varied linguistic properties and data availability.
You can open the
pre-recorded video
in a separate window.
NOTE: The SlidesLive video may display a random order of the authors.
The correct author list is shown at the top of this webpage.
Similar Papers
Double-Hard Debias: Tailoring Word Embeddings for Gender Bias Mitigation
Tianlu Wang, Xi Victoria Lin, Nazneen Fatema Rajani, Bryan McCann, Vicente Ordonez, Caiming Xiong,
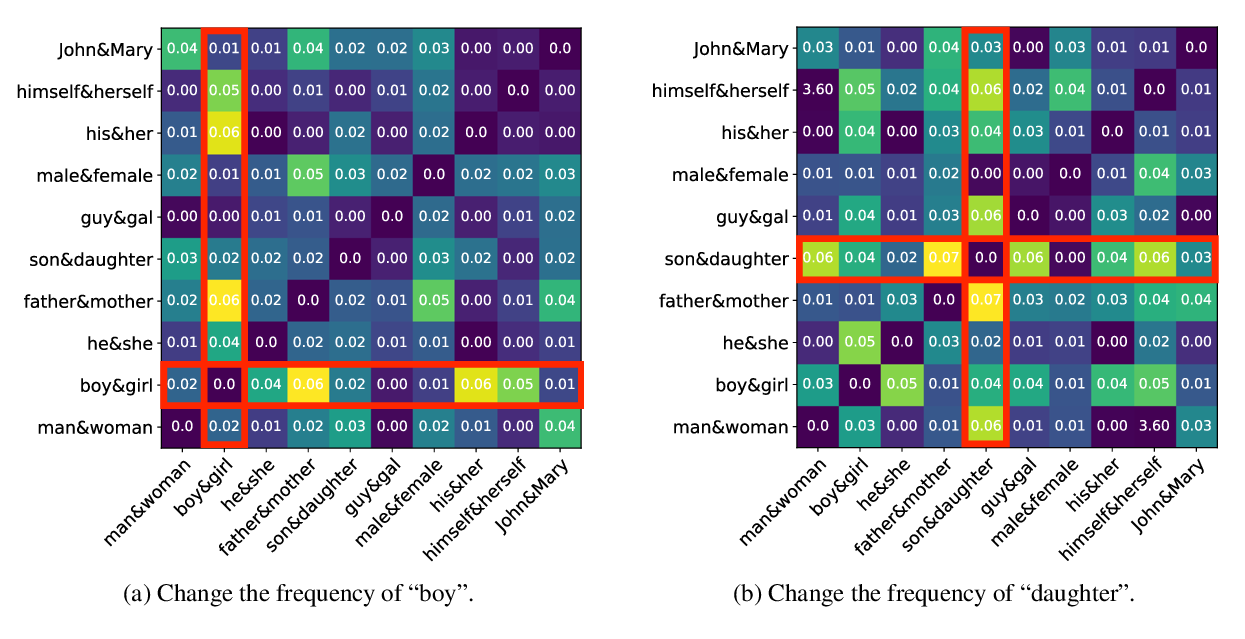
Gender in Danger? Evaluating Speech Translation Technology on the MuST-SHE Corpus
Luisa Bentivogli, Beatrice Savoldi, Matteo Negri, Mattia A. Di Gangi, Roldano Cattoni, Marco Turchi,
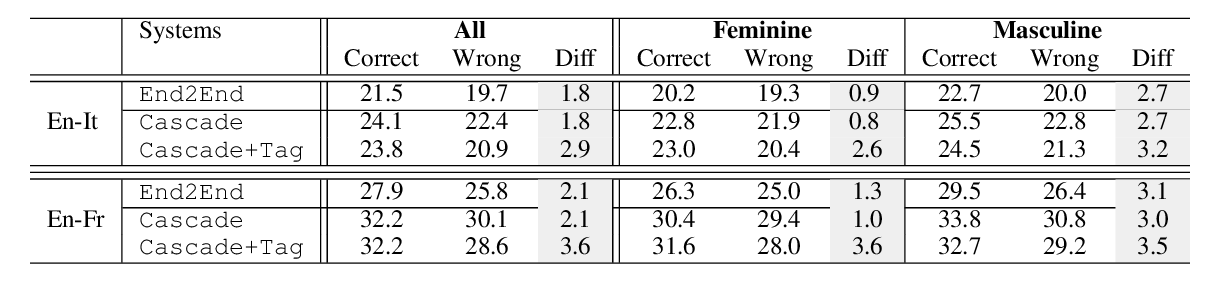
On Exposure Bias, Hallucination and Domain Shift in Neural Machine Translation
Chaojun Wang, Rico Sennrich,
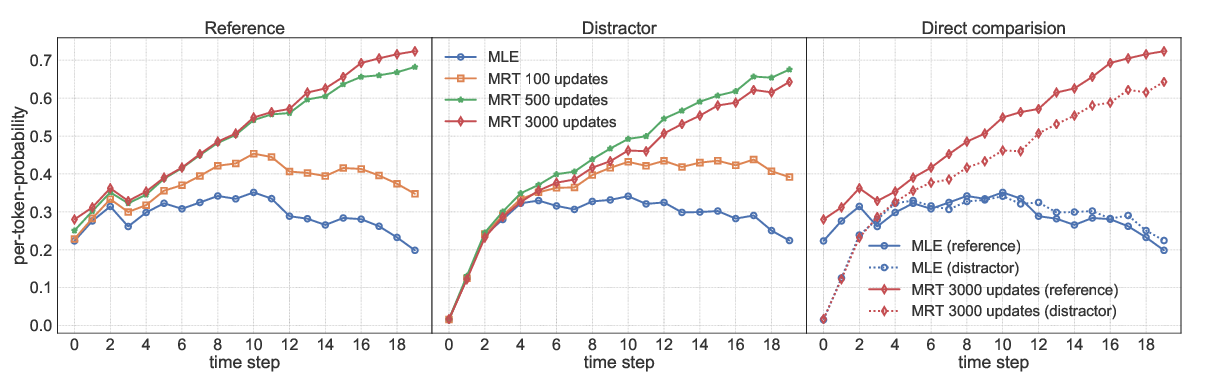