Meta-Reinforced Multi-Domain State Generator for Dialogue Systems
Yi Huang, Junlan Feng, Min Hu, Xiaoting Wu, Xiaoyu Du, Shuo Ma
Dialogue and Interactive Systems Long Paper
Session 12B: Jul 8
(09:00-10:00 GMT)
Session 13B: Jul 8
(13:00-14:00 GMT)
Abstract:
A Dialogue State Tracker (DST) is a core component of a modular task-oriented dialogue system. Tremendous progress has been made in recent years. However, the major challenges remain. The state-of-the-art accuracy for DST is below 50% for a multi-domain dialogue task. A learnable DST for any new domain requires a large amount of labeled in-domain data and training from scratch. In this paper, we propose a Meta-Reinforced Multi-Domain State Generator (MERET). Our first contribution is to improve the DST accuracy. We enhance a neural model based DST generator with a reward manager, which is built on policy gradient reinforcement learning (RL) to fine-tune the generator. With this change, we are able to improve the joint accuracy of DST from 48.79% to 50.91% on the MultiWOZ corpus. Second, we explore to train a DST meta-learning model with a few domains as source domains and a new domain as target domain. We apply the model-agnostic meta-learning algorithm (MAML) to DST and the obtained meta-learning model is used for new domain adaptation. Our experimental results show this solution is able to outperform the traditional training approach with extremely less training data in target domain.
You can open the
pre-recorded video
in a separate window.
NOTE: The SlidesLive video may display a random order of the authors.
The correct author list is shown at the top of this webpage.
Similar Papers
Efficient Dialogue State Tracking by Selectively Overwriting Memory
Sungdong Kim, Sohee Yang, Gyuwan Kim, Sang-Woo Lee,
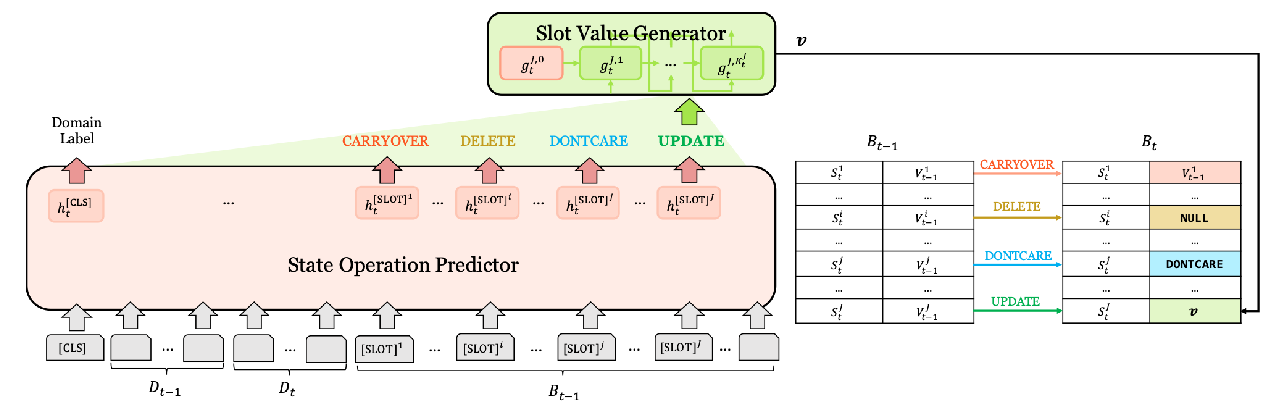
Dialogue State Tracking with Explicit Slot Connection Modeling
Yawen Ouyang, Moxin Chen, Xinyu Dai, Yinggong Zhao, Shujian Huang, Jiajun Chen,
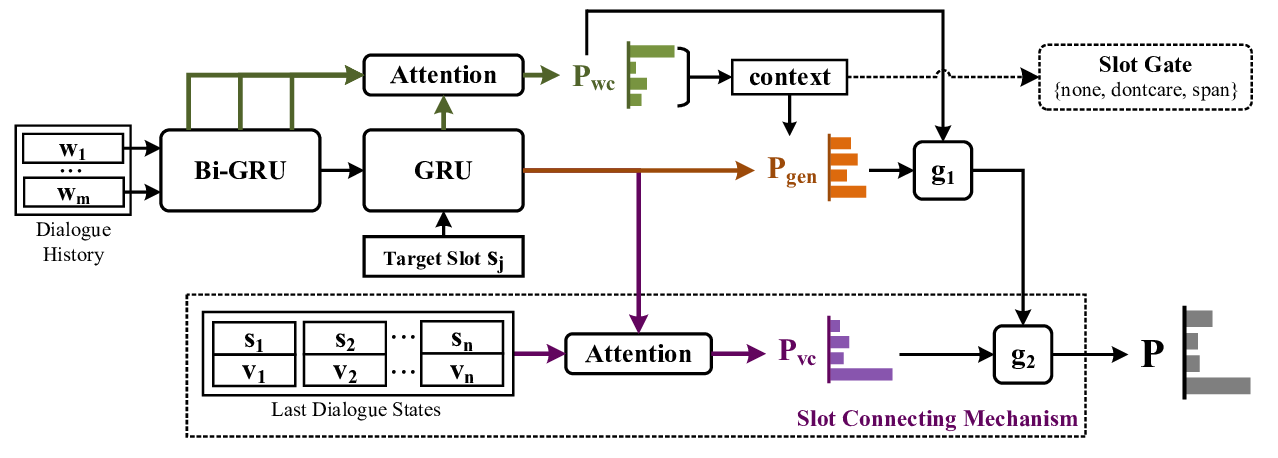
Zero-Shot Transfer Learning with Synthesized Data for Multi-Domain Dialogue State Tracking
Giovanni Campagna, Agata Foryciarz, Mehrad Moradshahi, Monica Lam,
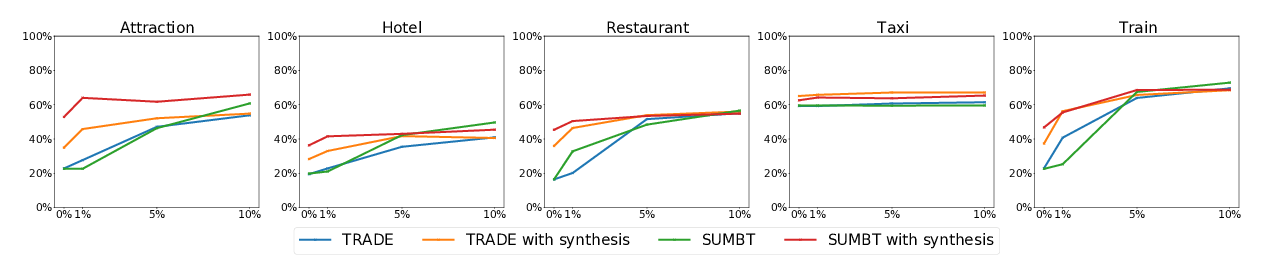
Learning Dialog Policies from Weak Demonstrations
Gabriel Gordon-Hall, Philip John Gorinski, Shay B. Cohen,
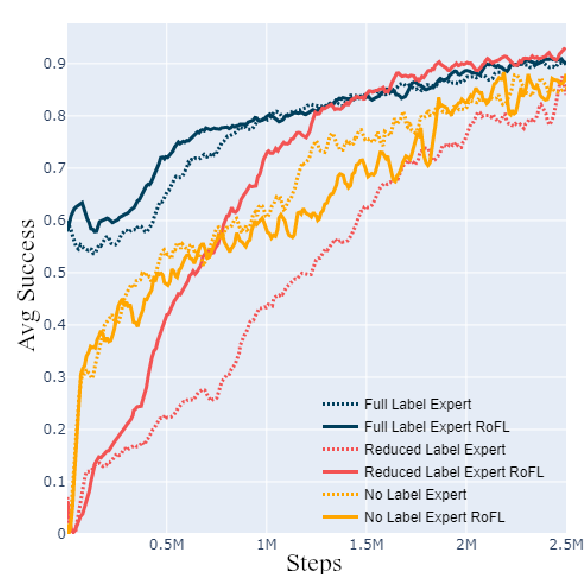