Explicit Semantic Decomposition for Definition Generation
Jiahuan Li, Yu Bao, Shujian Huang, Xinyu Dai, Jiajun Chen
Generation Long Paper
Session 1B: Jul 6
(06:00-07:00 GMT)
Session 2B: Jul 6
(09:00-10:00 GMT)
Abstract:
Definition generation, which aims to automatically generate dictionary definitions for words, has recently been proposed to assist the construction of dictionaries and help people understand unfamiliar texts. However, previous works hardly consider explicitly modeling the ``components'' of definitions, leading to under-specific generation results. In this paper, we propose ESD, namely Explicit Semantic Decomposition for definition Generation, which explicitly decomposes the meaning of words into semantic components, and models them with discrete latent variables for definition generation. Experimental results show that \method achieves top results on WordNet and Oxford benchmarks, outperforming strong previous baselines.
You can open the
pre-recorded video
in a separate window.
NOTE: The SlidesLive video may display a random order of the authors.
The correct author list is shown at the top of this webpage.
Similar Papers
Evidence-Aware Inferential Text Generation with Vector Quantised Variational AutoEncoder
Daya Guo, Duyu Tang, Nan Duan, Jian Yin, Daxin Jiang, Ming Zhou,
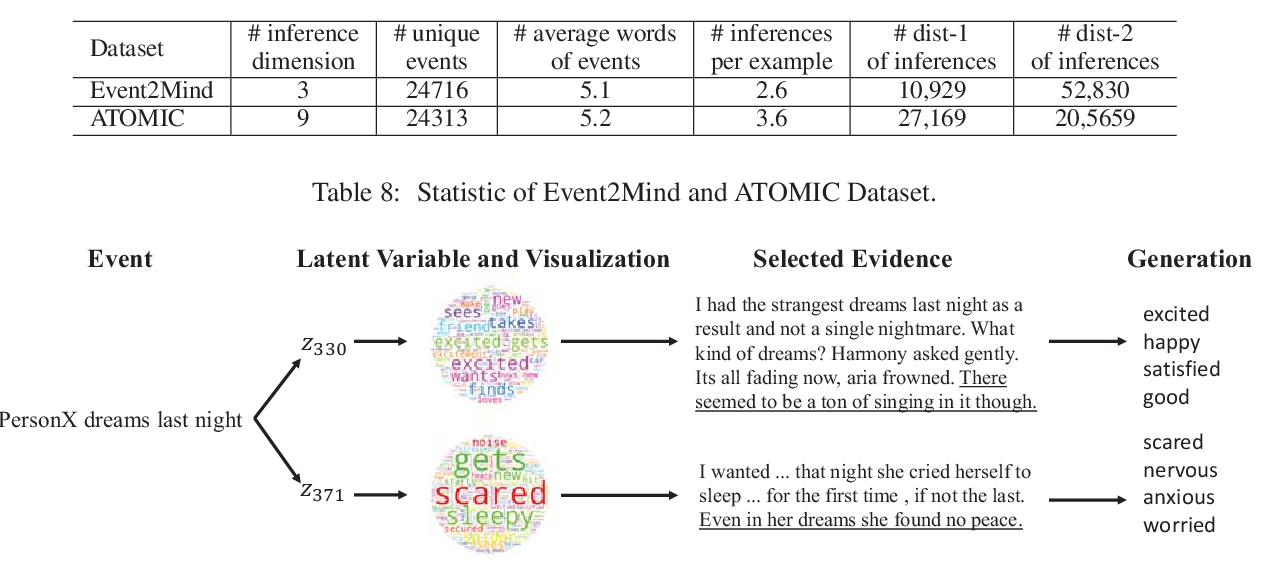
A Batch Normalized Inference Network Keeps the KL Vanishing Away
Qile Zhu, Wei Bi, Xiaojiang Liu, Xiyao Ma, Xiaolin Li, Dapeng Wu,
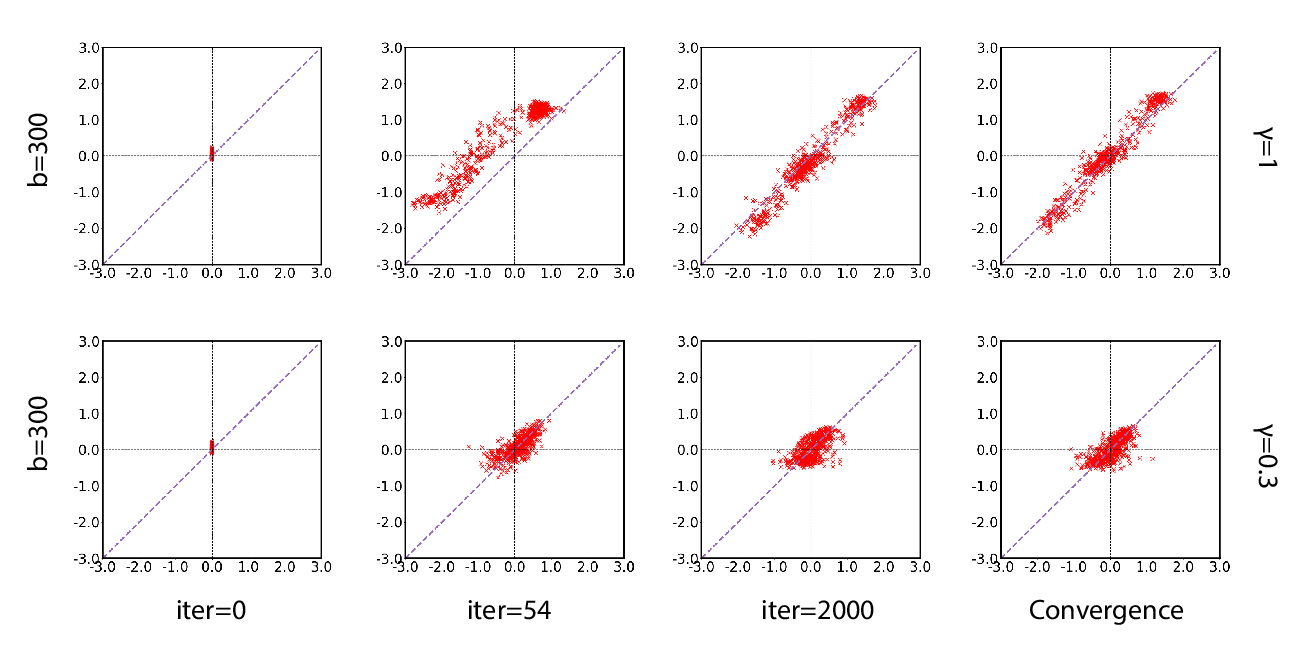
One Size Does Not Fit All: Generating and Evaluating Variable Number of Keyphrases
Xingdi Yuan, Tong Wang, Rui Meng, Khushboo Thaker, Peter Brusilovsky, Daqing He, Adam Trischler,
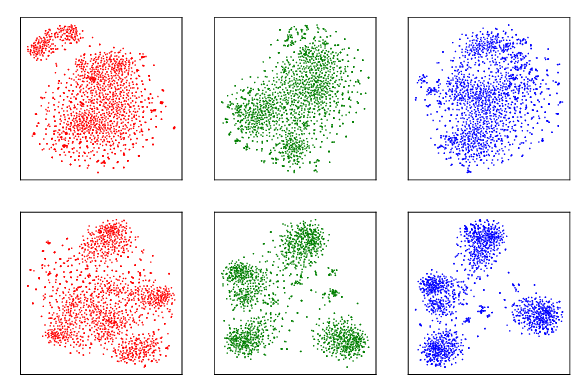
Variational Neural Machine Translation with Normalizing Flows
Hendra Setiawan, Matthias Sperber, Udhyakumar Nallasamy, Matthias Paulik,
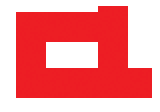