Premise Selection in Natural Language Mathematical Texts
Deborah Ferreira, André Freitas
Semantics: Textual Inference and Other Areas of Semantics Long Paper
Session 12B: Jul 8
(09:00-10:00 GMT)
Session 13A: Jul 8
(12:00-13:00 GMT)
Abstract:
The discovery of supporting evidence for addressing complex mathematical problems is a semantically challenging task, which is still unexplored in the field of natural language processing for mathematical text. The natural language premise selection task consists in using conjectures written in both natural language and mathematical formulae to recommend premises that most likely will be useful to prove a particular statement. We propose an approach to solve this task as a link prediction problem, using Deep Convolutional Graph Neural Networks. This paper also analyses how different baselines perform in this task and shows that a graph structure can provide higher F1-score, especially when considering multi-hop premise selection.
You can open the
pre-recorded video
in a separate window.
NOTE: The SlidesLive video may display a random order of the authors.
The correct author list is shown at the top of this webpage.
Similar Papers
Bridging the Structural Gap Between Encoding and Decoding for Data-To-Text Generation
Chao Zhao, Marilyn Walker, Snigdha Chaturvedi,
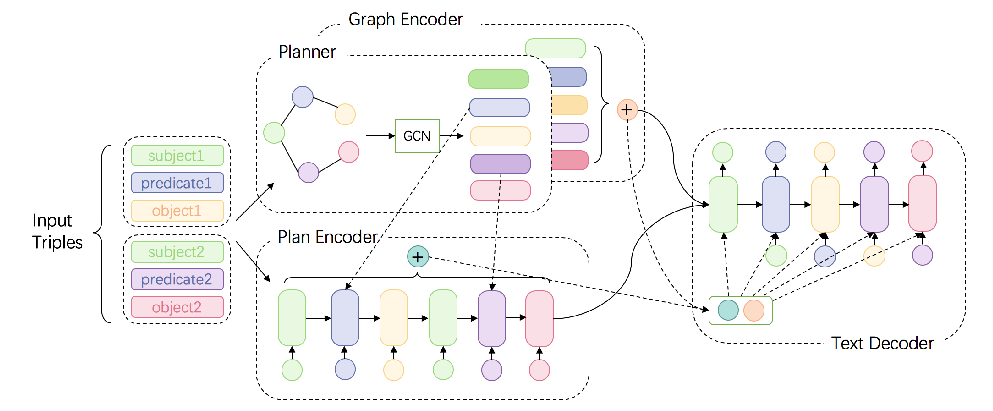
Line Graph Enhanced AMR-to-Text Generation with Mix-Order Graph Attention Networks
Yanbin Zhao, Lu Chen, Zhi Chen, Ruisheng Cao, Su Zhu, Kai Yu,
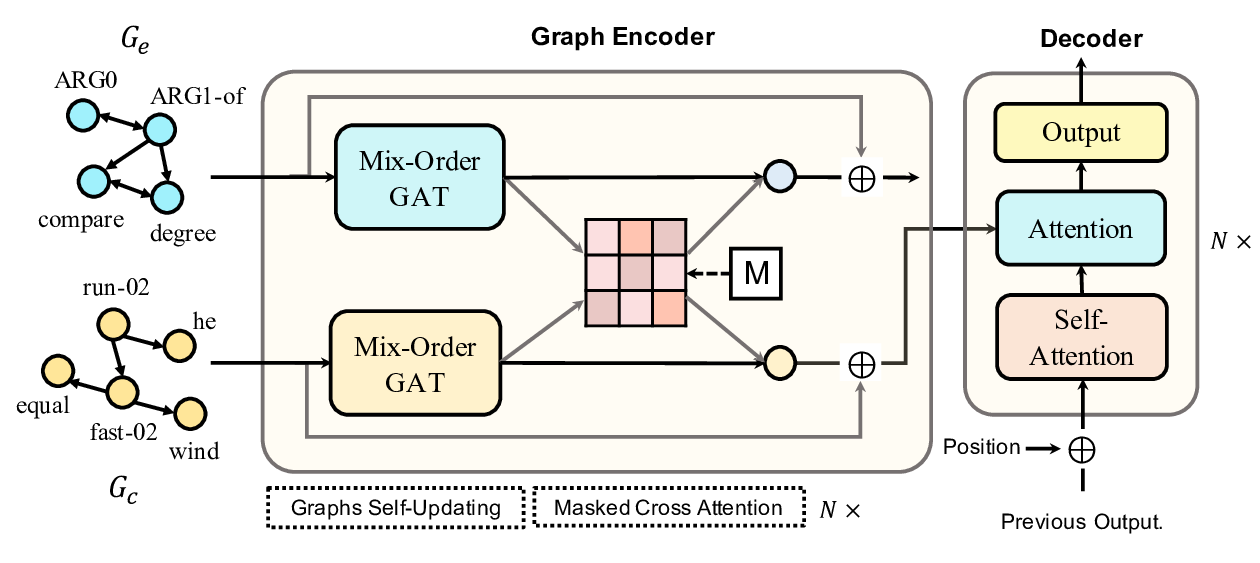
Reasoning Over Semantic-Level Graph for Fact Checking
Wanjun Zhong, Jingjing Xu, Duyu Tang, Zenan Xu, Nan Duan, Ming Zhou, Jiahai Wang, Jian Yin,
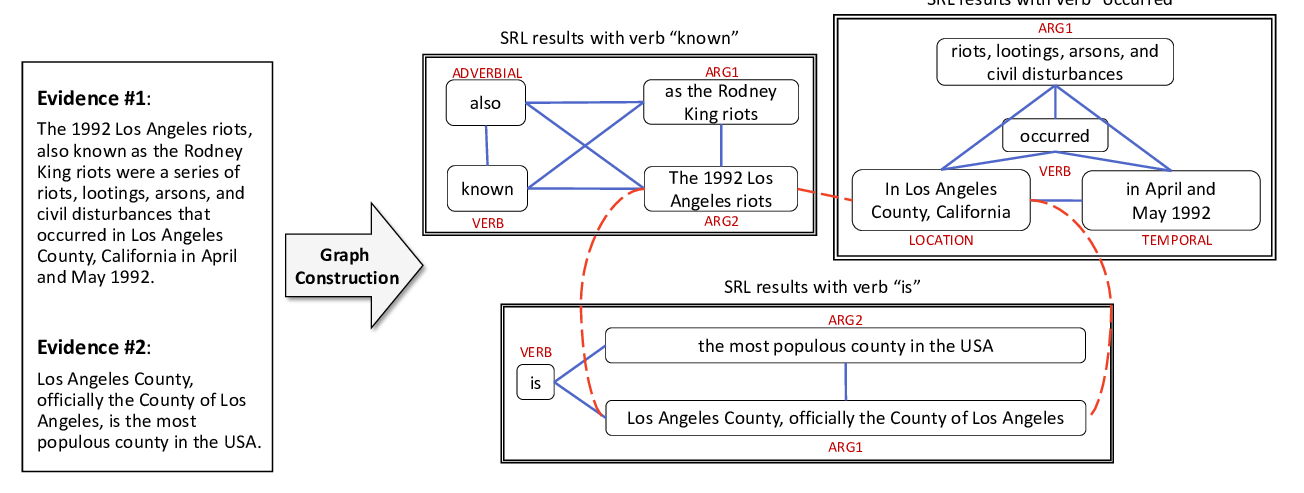
INFOTABS: Inference on Tables as Semi-structured Data
Vivek Gupta, Maitrey Mehta, Pegah Nokhiz, Vivek Srikumar,
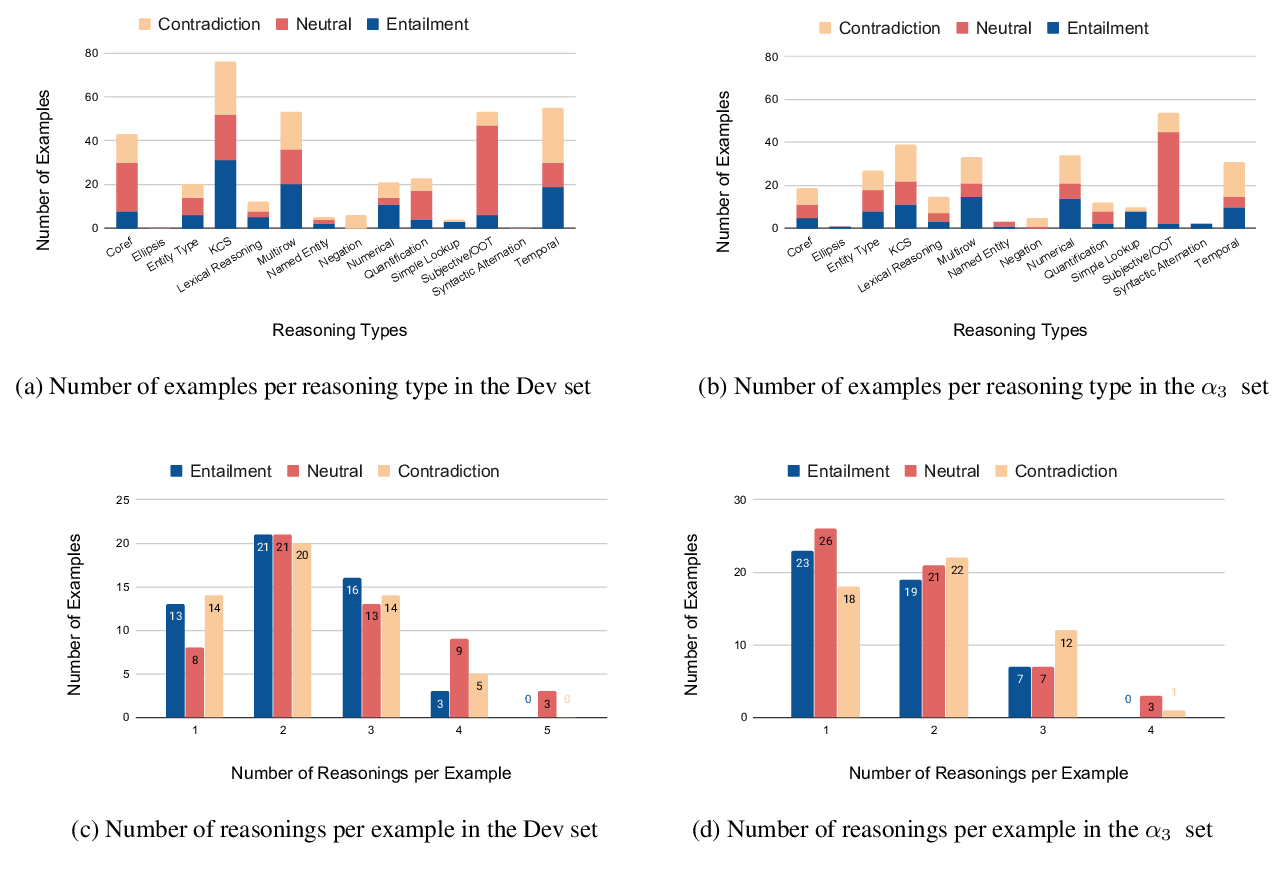