A Two-Step Approach for Implicit Event Argument Detection
Zhisong Zhang, Xiang Kong, Zhengzhong Liu, Xuezhe Ma, Eduard Hovy
Information Extraction Short Paper
Session 13A: Jul 8
(12:00-13:00 GMT)
Session 15A: Jul 8
(20:00-21:00 GMT)
Abstract:
In this work, we explore the implicit event argument detection task, which studies event arguments beyond sentence boundaries. The addition of cross-sentence argument candidates imposes great challenges for modeling. To reduce the number of candidates, we adopt a two-step approach, decomposing the problem into two sub-problems: argument head-word detection and head-to-span expansion. Evaluated on the recent RAMS dataset (Ebner et al., 2020), our model achieves overall better performance than a strong sequence labeling baseline. We further provide detailed error analysis, presenting where the model mainly makes errors and indicating directions for future improvements. It remains a challenge to detect implicit arguments, calling for more future work of document-level modeling for this task.
You can open the
pre-recorded video
in a separate window.
NOTE: The SlidesLive video may display a random order of the authors.
The correct author list is shown at the top of this webpage.
Similar Papers
Multi-Sentence Argument Linking
Seth Ebner, Patrick Xia, Ryan Culkin, Kyle Rawlins, Benjamin Van Durme,
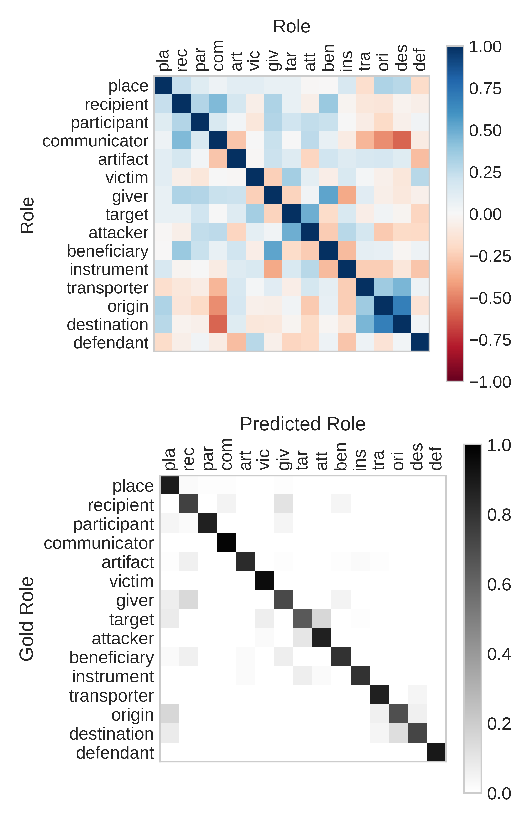
From Arguments to Key Points: Towards Automatic Argument Summarization
Roy Bar-Haim, Lilach Eden, Roni Friedman, Yoav Kantor, Dan Lahav, Noam Slonim,
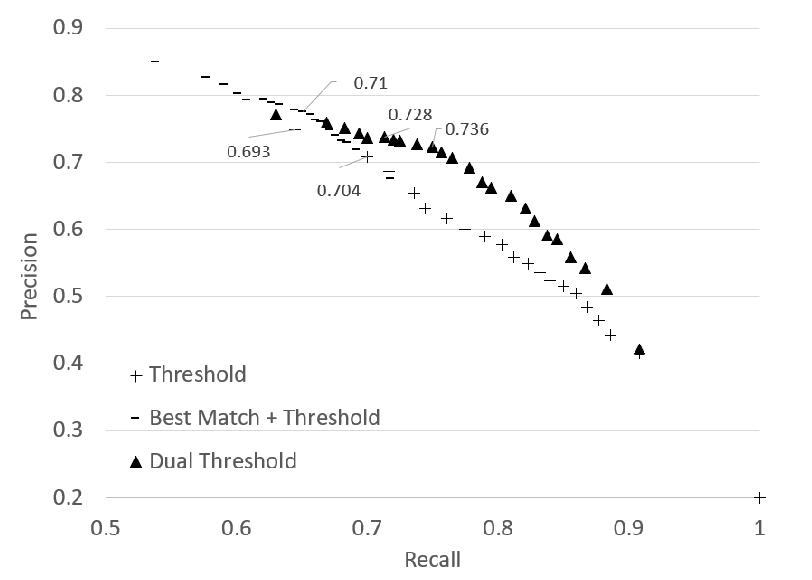
Document-Level Event Role Filler Extraction using Multi-Granularity Contextualized Encoding
Xinya Du, Claire Cardie,
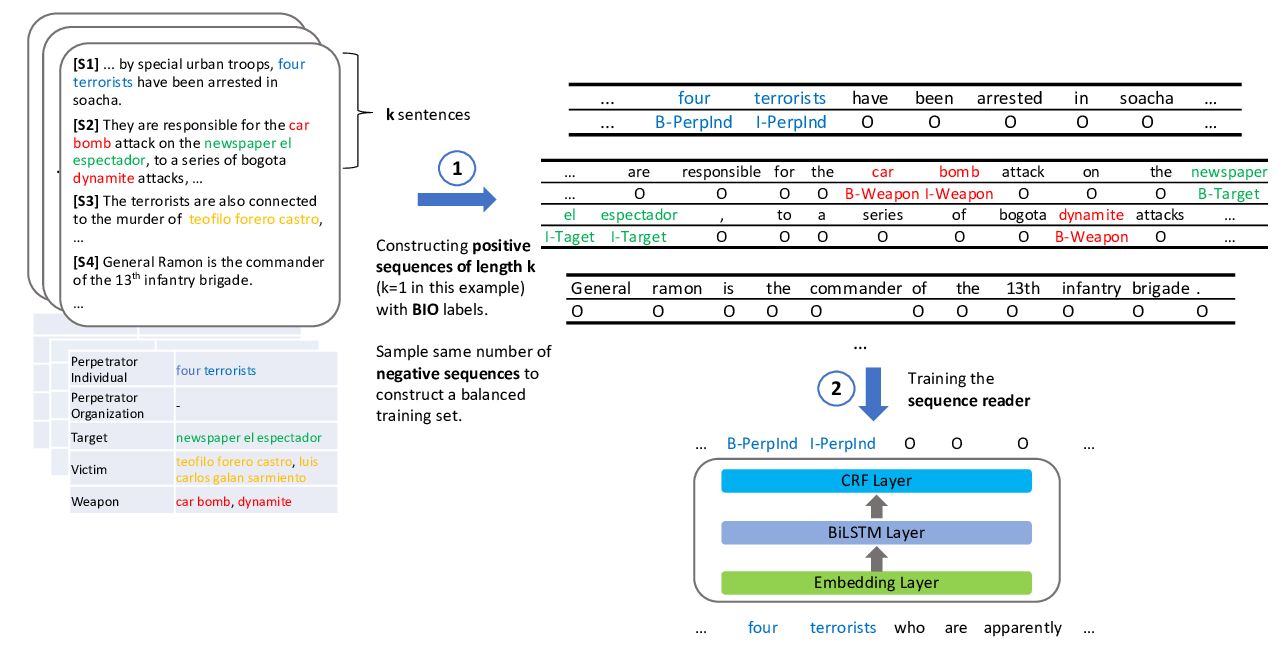