From Arguments to Key Points: Towards Automatic Argument Summarization
Roy Bar-Haim, Lilach Eden, Roni Friedman, Yoav Kantor, Dan Lahav, Noam Slonim
Sentiment Analysis, Stylistic Analysis, and Argument Mining Long Paper
Session 7A: Jul 7
(08:00-09:00 GMT)
Session 8B: Jul 7
(13:00-14:00 GMT)
Abstract:
Generating a concise summary from a large collection of arguments on a given topic is an intriguing yet understudied problem. We propose to represent such summaries as a small set of talking points, termed key points, each scored according to its salience. We show, by analyzing a large dataset of crowd-contributed arguments, that a small number of key points per topic is typically sufficient for covering the vast majority of the arguments. Furthermore, we found that a domain expert can often predict these key points in advance. We study the task of argument-to-key point mapping, and introduce a novel large-scale dataset for this task. We report empirical results for an extensive set of experiments with this dataset, showing promising performance.
You can open the
pre-recorded video
in a separate window.
NOTE: The SlidesLive video may display a random order of the authors.
The correct author list is shown at the top of this webpage.
Similar Papers
A Two-Step Approach for Implicit Event Argument Detection
Zhisong Zhang, Xiang Kong, Zhengzhong Liu, Xuezhe Ma, Eduard Hovy,
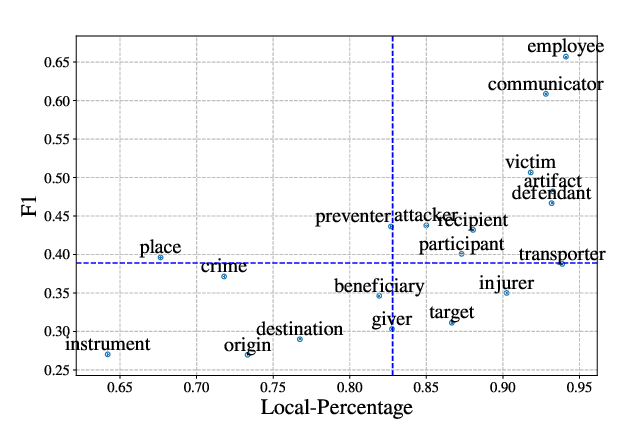
Exploring Content Selection in Summarization of Novel Chapters
Faisal Ladhak, Bryan Li, Yaser Al-Onaizan, Kathy McKeown,
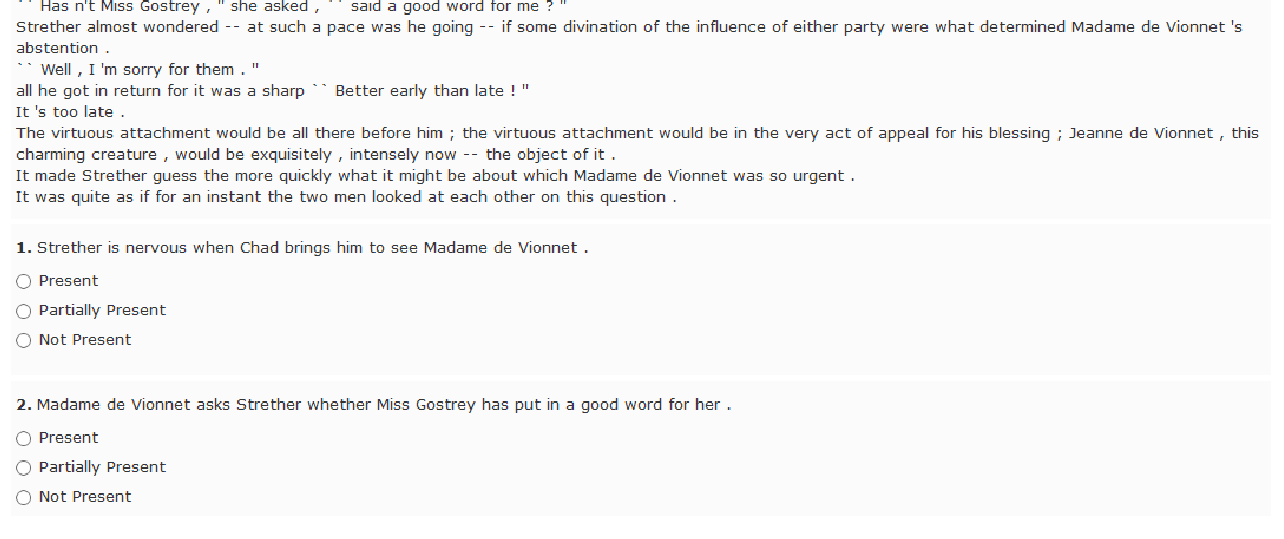
Towards Better Non-Tree Argument Mining: Proposition-Level Biaffine Parsing with Task-Specific Parameterization
Gaku Morio, Hiroaki Ozaki, Terufumi Morishita, Yuta Koreeda, Kohsuke Yanai,
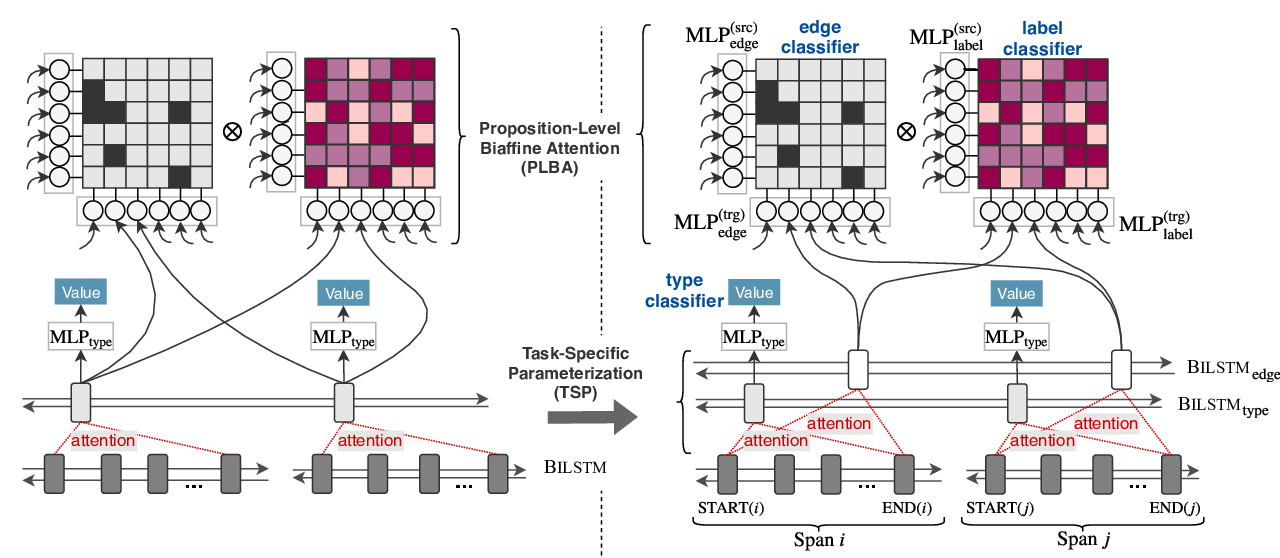
Topic Balancing with Additive Regularization of Topic Models
Eugeniia Veselova, Konstantin Vorontsov,
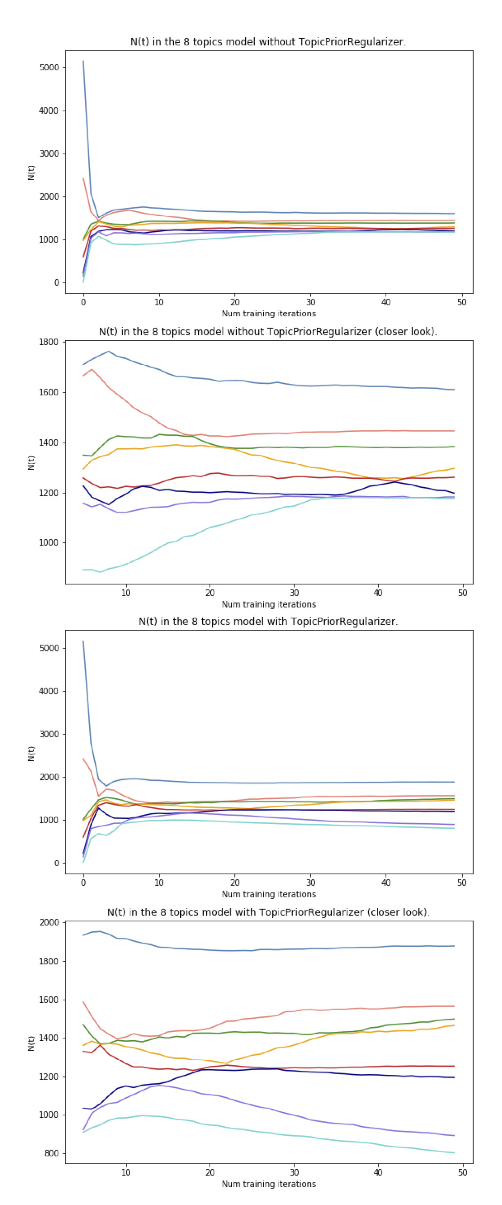