Temporal Common Sense Acquisition with Minimal Supervision
Ben Zhou, Qiang Ning, Daniel Khashabi, Dan Roth
Semantics: Textual Inference and Other Areas of Semantics Long Paper
Session 13A: Jul 8
(12:00-13:00 GMT)
Session 14B: Jul 8
(18:00-19:00 GMT)
Abstract:
Temporal common sense (e.g., duration and frequency of events) is crucial for understanding natural language. However, its acquisition is challenging, partly because such information is often not expressed explicitly in text, and human annotation on such concepts is costly. This work proposes a novel sequence modeling approach that exploits explicit and implicit mentions of temporal common sense, extracted from a large corpus, to build TacoLM, a temporal common sense language model. Our method is shown to give quality predictions of various dimensions of temporal common sense (on UDST and a newly collected dataset from RealNews). It also produces representations of events for relevant tasks such as duration comparison, parent-child relations, event coreference and temporal QA (on TimeBank, HiEVE and MCTACO) that are better than using the standard BERT. Thus, it will be an important component of temporal NLP.
You can open the
pre-recorded video
in a separate window.
NOTE: The SlidesLive video may display a random order of the authors.
The correct author list is shown at the top of this webpage.
Similar Papers
Moving Down the Long Tail of Word Sense Disambiguation with Gloss Informed Bi-encoders
Terra Blevins, Luke Zettlemoyer,
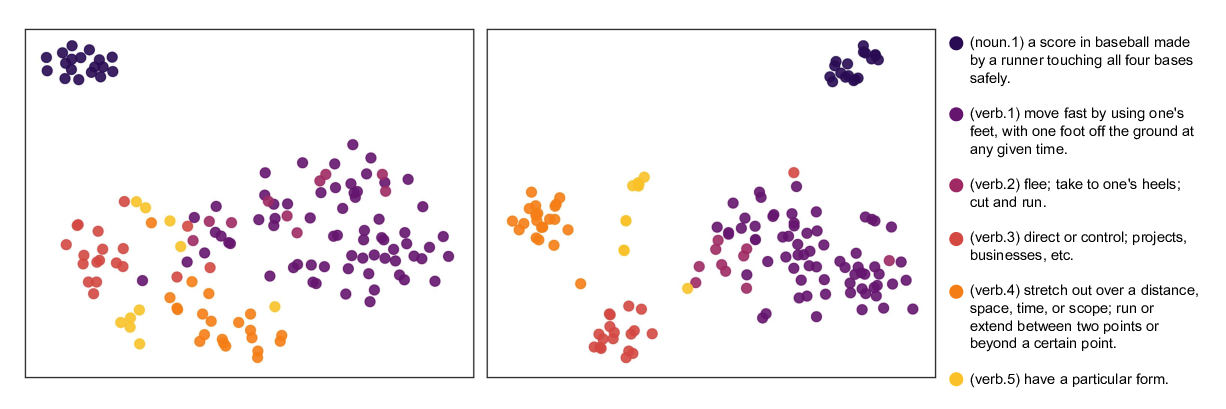
SenseBERT: Driving Some Sense into BERT
Yoav Levine, Barak Lenz, Or Dagan, Ori Ram, Dan Padnos, Or Sharir, Shai Shalev-Shwartz, Amnon Shashua, Yoav Shoham,
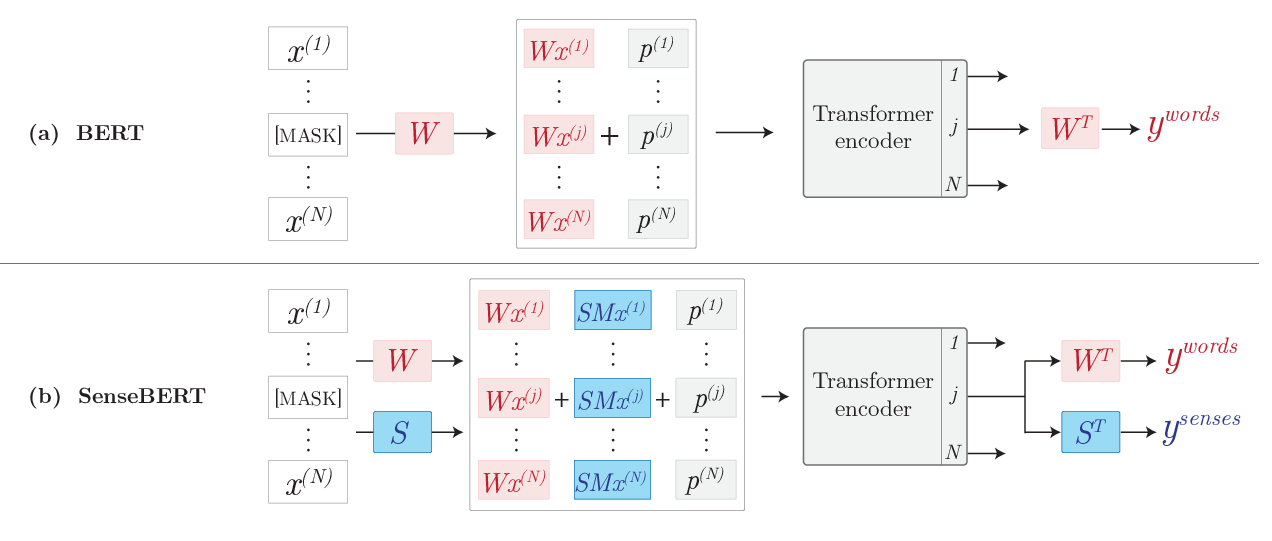
Machine Reading of Historical Events
Or Honovich, Lucas Torroba Hennigen, Omri Abend, Shay B. Cohen,
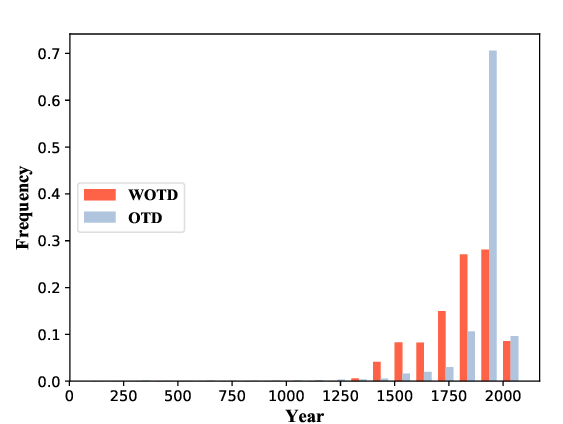