SciREX: A Challenge Dataset for Document-Level Information Extraction
Sarthak Jain, Madeleine van Zuylen, Hannaneh Hajishirzi, Iz Beltagy
Information Extraction Long Paper
Session 13A: Jul 8
(12:00-13:00 GMT)
Session 15B: Jul 8
(21:00-22:00 GMT)
Abstract:
Extracting information from full documents is an important problem in many domains, but most previous work focus on identifying relationships within a sentence or a paragraph. It is challenging to create a large-scale information extraction (IE) dataset at the document level since it requires an understanding of the whole document to annotate entities and their document-level relationships that usually span beyond sentences or even sections. In this paper, we introduce SciREX, a document level IE dataset that encompasses multiple IE tasks, including salient entity identification and document level N-ary relation identification from scientific articles. We annotate our dataset by integrating automatic and human annotations, leveraging existing scientific knowledge resources. We develop a neural model as a strong baseline that extends previous state-of-the-art IE models to document-level IE. Analyzing the model performance shows a significant gap between human performance and current baselines, inviting the community to use our dataset as a challenge to develop document-level IE models. Our data and code are publicly available at https://github.com/allenai/SciREX .
You can open the
pre-recorded video
in a separate window.
NOTE: The SlidesLive video may display a random order of the authors.
The correct author list is shown at the top of this webpage.
Similar Papers
Reasoning with Latent Structure Refinement for Document-Level Relation Extraction
Guoshun Nan, Zhijiang Guo, Ivan Sekulic, Wei Lu,
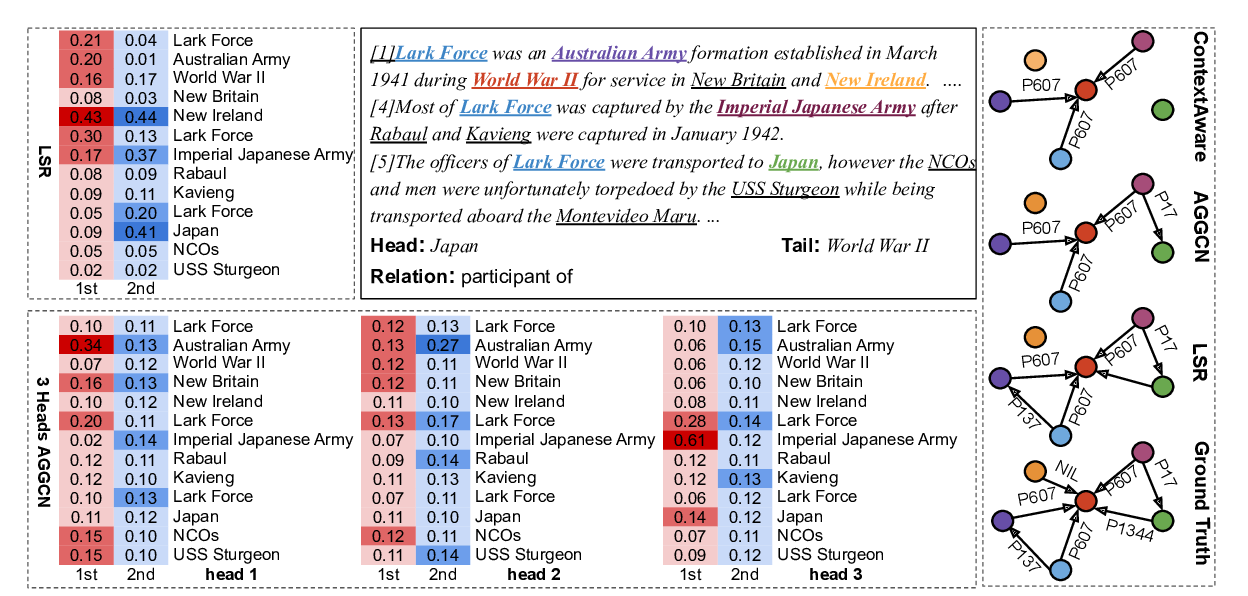
SPECTER: Document-level Representation Learning using Citation-informed Transformers
Arman Cohan, Sergey Feldman, Iz Beltagy, Doug Downey, Daniel Weld,
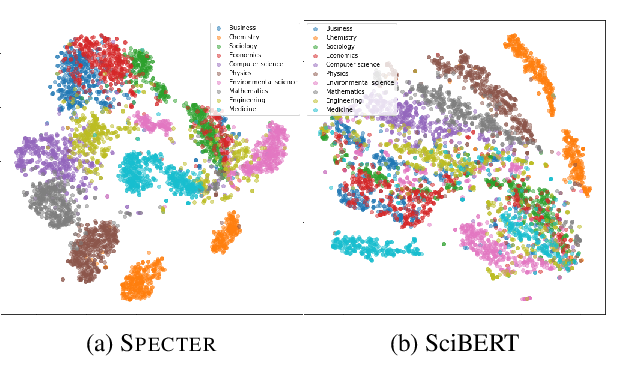
Semantic Graphs for Generating Deep Questions
Liangming Pan, Yuxi Xie, Yansong Feng, Tat-Seng Chua, Min-Yen Kan,
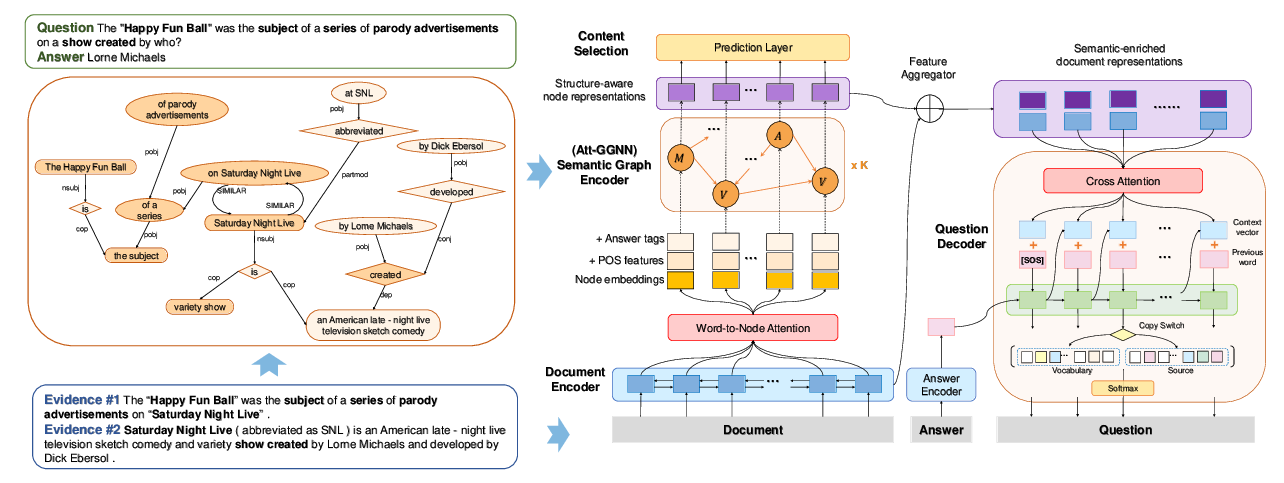
In Layman’s Terms: Semi-Open Relation Extraction from Scientific Texts
Ruben Kruiper, Julian Vincent, Jessica Chen-Burger, Marc Desmulliez, Ioannis Konstas,
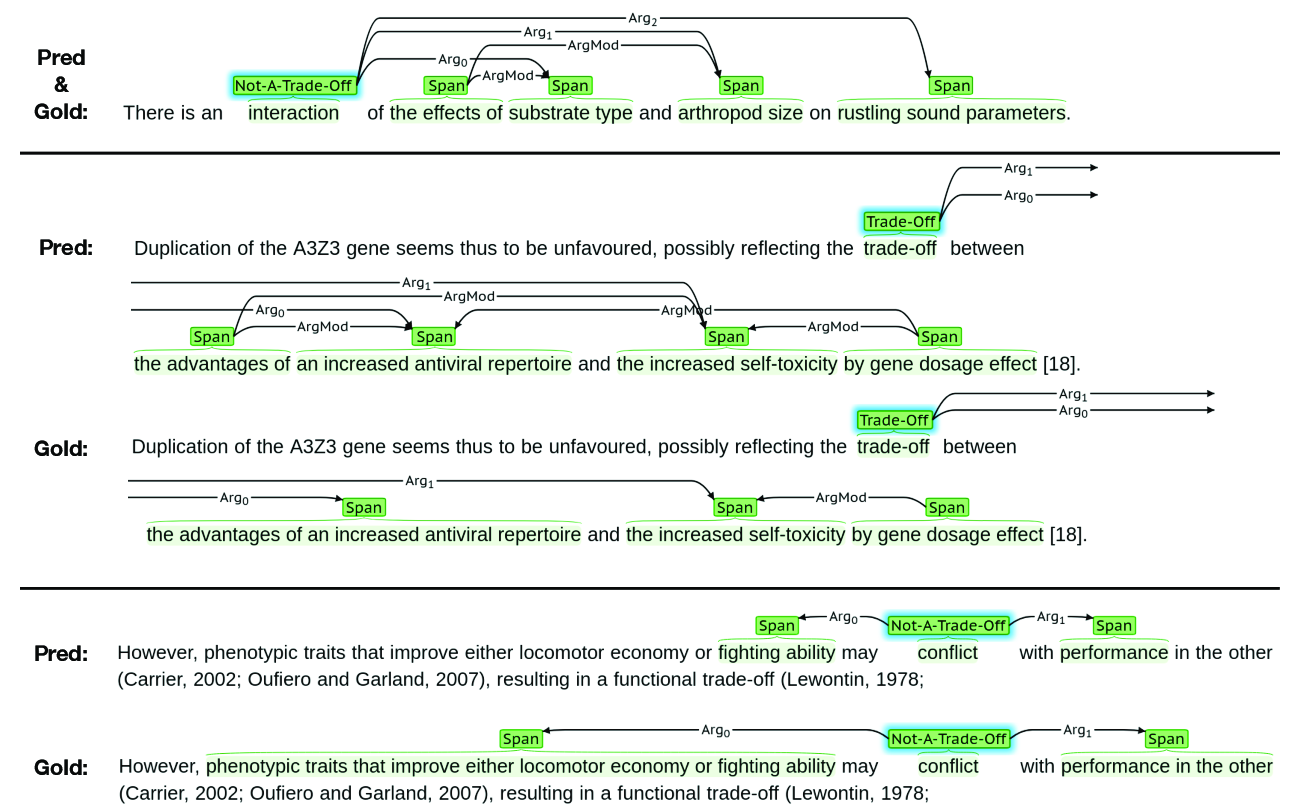