Reasoning with Latent Structure Refinement for Document-Level Relation Extraction
Guoshun Nan, Zhijiang Guo, Ivan Sekulic, Wei Lu
Information Extraction Long Paper
Session 2B: Jul 6
(09:00-10:00 GMT)
Session 3B: Jul 6
(13:00-14:00 GMT)
Abstract:
Document-level relation extraction requires integrating information within and across multiple sentences of a document and capturing complex interactions between inter-sentence entities. However, effective aggregation of relevant information in the document remains a challenging research question. Existing approaches construct static document-level graphs based on syntactic trees, co-references or heuristics from the unstructured text to model the dependencies. Unlike previous methods that may not be able to capture rich non-local interactions for inference, we propose a novel model that empowers the relational reasoning across sentences by automatically inducing the latent document-level graph. We further develop a refinement strategy, which enables the model to incrementally aggregate relevant information for multi-hop reasoning. Specifically, our model achieves an F1 score of 59.05 on a large-scale document-level dataset (DocRED), significantly improving over the previous results, and also yields new state-of-the-art results on the CDR and GDA dataset. Furthermore, extensive analyses show that the model is able to discover more accurate inter-sentence relations.
You can open the
pre-recorded video
in a separate window.
NOTE: The SlidesLive video may display a random order of the authors.
The correct author list is shown at the top of this webpage.
Similar Papers
SciREX: A Challenge Dataset for Document-Level Information Extraction
Sarthak Jain, Madeleine van Zuylen, Hannaneh Hajishirzi, Iz Beltagy,
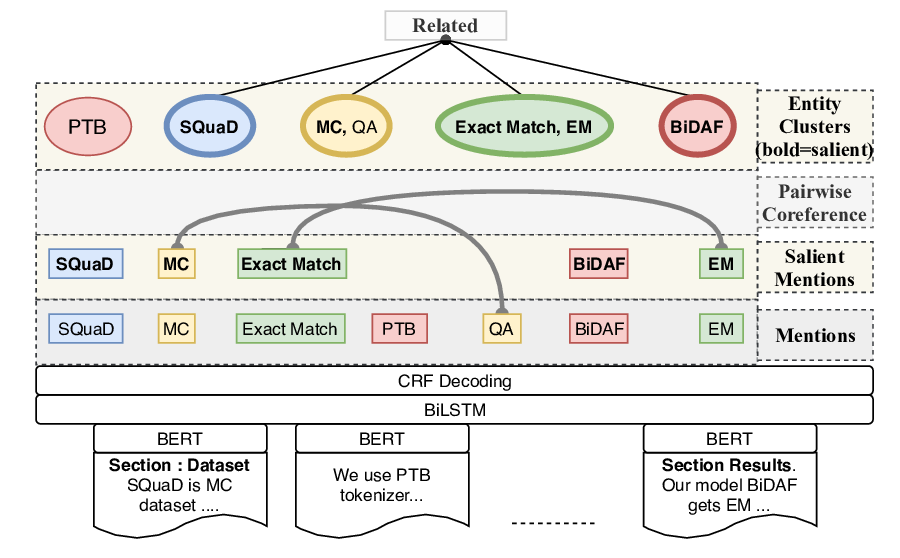
Semantic Graphs for Generating Deep Questions
Liangming Pan, Yuxi Xie, Yansong Feng, Tat-Seng Chua, Min-Yen Kan,
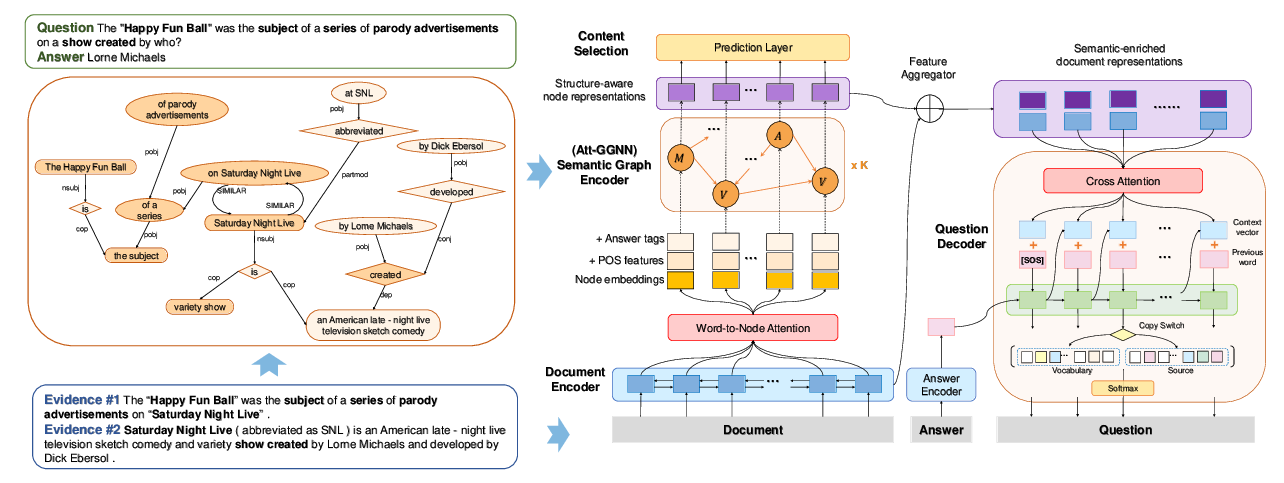
SPECTER: Document-level Representation Learning using Citation-informed Transformers
Arman Cohan, Sergey Feldman, Iz Beltagy, Doug Downey, Daniel Weld,
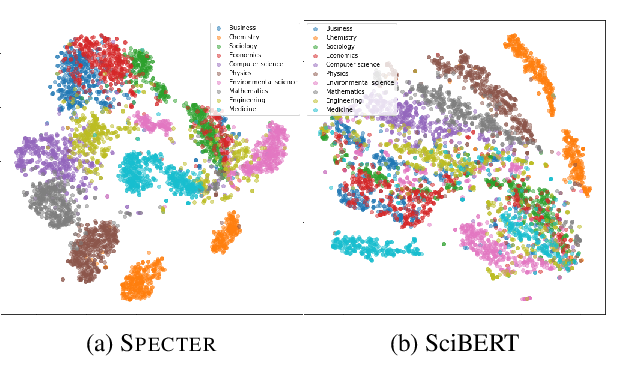
A Simple and Effective Unified Encoder for Document-Level Machine Translation
Shuming Ma, Dongdong Zhang, Ming Zhou,
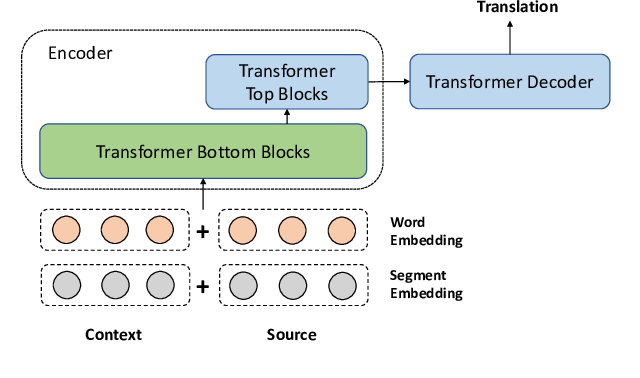