Do Transformers Need Deep Long-Range Memory?
Jack Rae, Ali Razavi
Machine Learning for NLP Short Paper
Session 13A: Jul 8
(12:00-13:00 GMT)
Session 14B: Jul 8
(18:00-19:00 GMT)
Abstract:
Deep attention models have advanced the modelling of sequential data across many domains. For language modelling in particular, the Transformer-XL --- a Transformer augmented with a long-range memory of past activations --- has been shown to be state-of-the-art across a variety of well-studied benchmarks. The Transformer-XL incorporates a long-range memory at every layer of the network, which renders its state to be thousands of times larger than RNN predecessors. However it is unclear whether this is necessary. We perform a set of interventions to show that comparable performance can be obtained with 6X fewer long range memories and better performance can be obtained by limiting the range of attention in lower layers of the network.
You can open the
pre-recorded video
in a separate window.
NOTE: The SlidesLive video may display a random order of the authors.
The correct author list is shown at the top of this webpage.
Similar Papers
Learning Source Phrase Representations for Neural Machine Translation
Hongfei Xu, Josef van Genabith, Deyi Xiong, Qiuhui Liu, Jingyi Zhang,
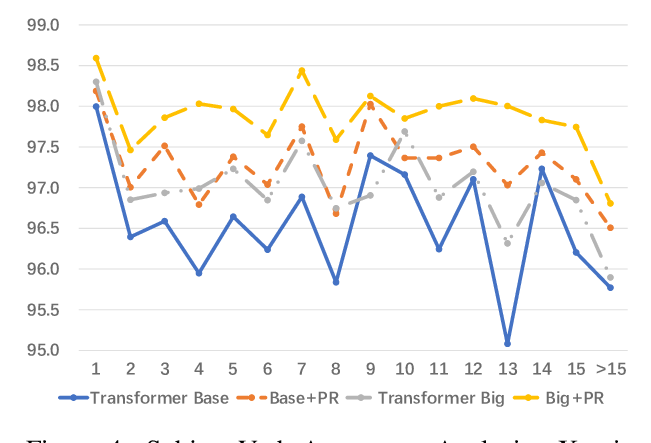
Lipschitz Constrained Parameter Initialization for Deep Transformers
Hongfei Xu, Qiuhui Liu, Josef van Genabith, Deyi Xiong, Jingyi Zhang,
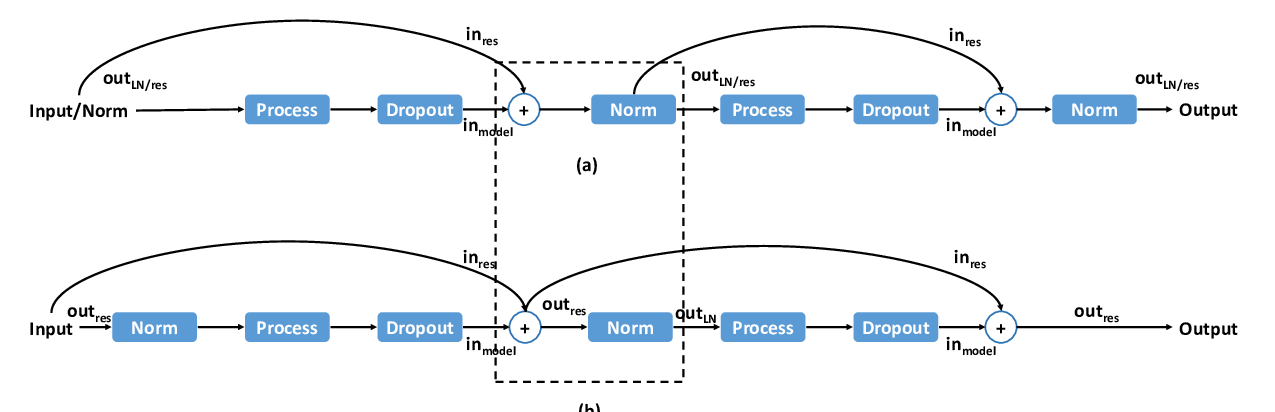