Non-Linear Instance-Based Cross-Lingual Mapping for Non-Isomorphic Embedding Spaces
Goran Glavaš, Ivan Vulić
Semantics: Lexical Short Paper
Session 13A: Jul 8
(12:00-13:00 GMT)
Session 14B: Jul 8
(18:00-19:00 GMT)
Abstract:
We present InstaMap, an instance-based method for learning projection-based cross-lingual word embeddings. Unlike prior work, it deviates from learning a single global linear projection. InstaMap is a non-parametric model that learns a non-linear projection by iteratively: (1) finding a globally optimal rotation of the source embedding space relying on the Kabsch algorithm, and then (2) moving each point along an instance-specific translation vector estimated from the translation vectors of the point's nearest neighbours in the training dictionary. We report performance gains with InstaMap over four representative state-of-the-art projection-based models on bilingual lexicon induction across a set of 28 diverse language pairs. We note prominent improvements, especially for more distant language pairs (i.e., languages with non-isomorphic monolingual spaces).
You can open the
pre-recorded video
in a separate window.
NOTE: The SlidesLive video may display a random order of the authors.
The correct author list is shown at the top of this webpage.
Similar Papers
Classification-Based Self-Learning for Weakly Supervised Bilingual Lexicon Induction
Mladen Karan, Ivan Vulić, Anna Korhonen, Goran Glavaš,
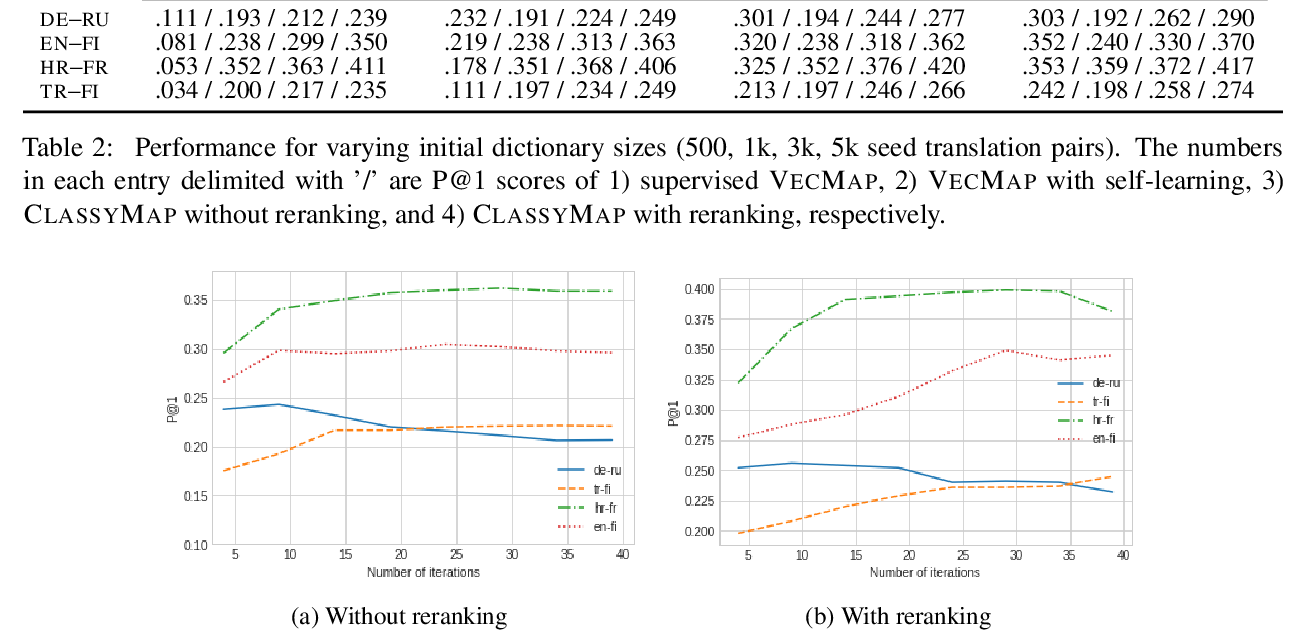
On the Cross-lingual Transferability of Monolingual Representations
Mikel Artetxe, Sebastian Ruder, Dani Yogatama,
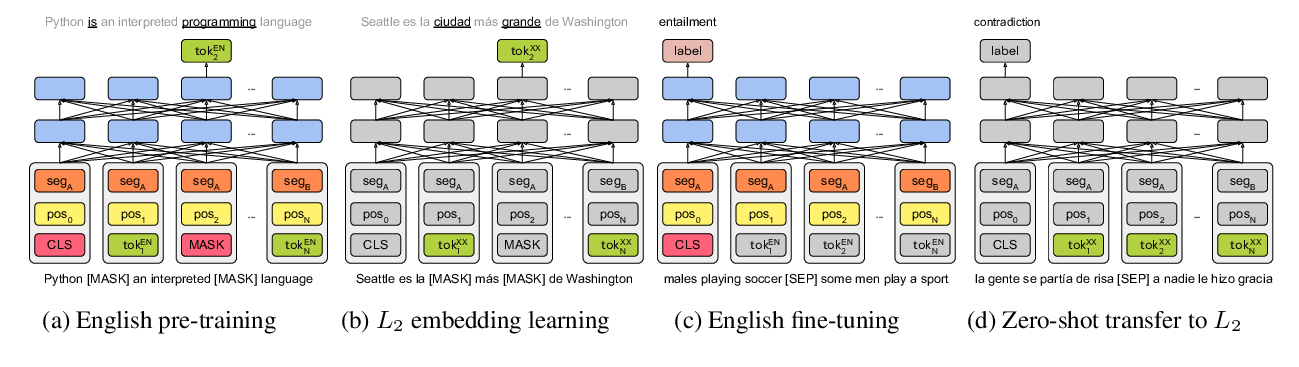
Bilingual Dictionary Based Neural Machine Translation without Using Parallel Sentences
Xiangyu Duan, Baijun Ji, Hao Jia, Min Tan, Min Zhang, Boxing Chen, Weihua Luo, Yue Zhang,
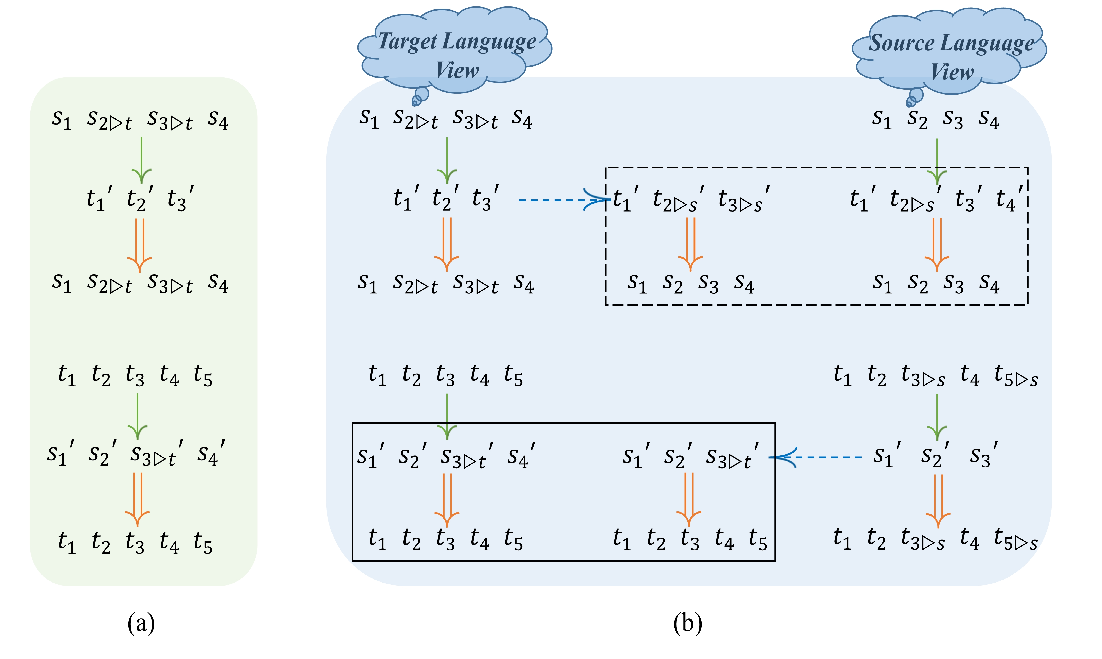