Good-Enough Compositional Data Augmentation
Jacob Andreas
Semantics: Sentence Level Long Paper
Session 13A: Jul 8
(12:00-13:00 GMT)
Session 15A: Jul 8
(20:00-21:00 GMT)
Abstract:
We propose a simple data augmentation protocol aimed at providing a compositional inductive bias in conditional and unconditional sequence models. Under this protocol, synthetic training examples are constructed by taking real training examples and replacing (possibly discontinuous) fragments with other fragments that appear in at least one similar environment. The protocol is model-agnostic and useful for a variety of tasks. Applied to neural sequence-to-sequence models, it reduces error rate by as much as 87% on diagnostic tasks from the SCAN dataset and 16% on a semantic parsing task. Applied to n-gram language models, it reduces perplexity by roughly 1% on small corpora in several languages.
You can open the
pre-recorded video
in a separate window.
NOTE: The SlidesLive video may display a random order of the authors.
The correct author list is shown at the top of this webpage.
Similar Papers
Mind the Trade-off: Debiasing NLU Models without Degrading the In-distribution Performance
Prasetya Ajie Utama, Nafise Sadat Moosavi, Iryna Gurevych,
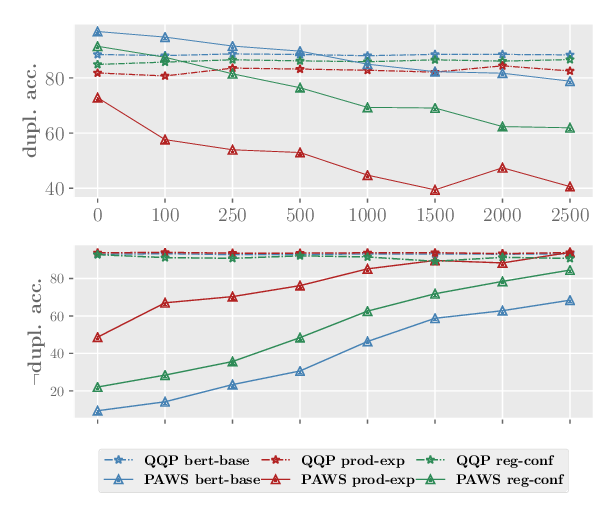
NAT: Noise-Aware Training for Robust Neural Sequence Labeling
Marcin Namysl, Sven Behnke, Joachim Köhler,
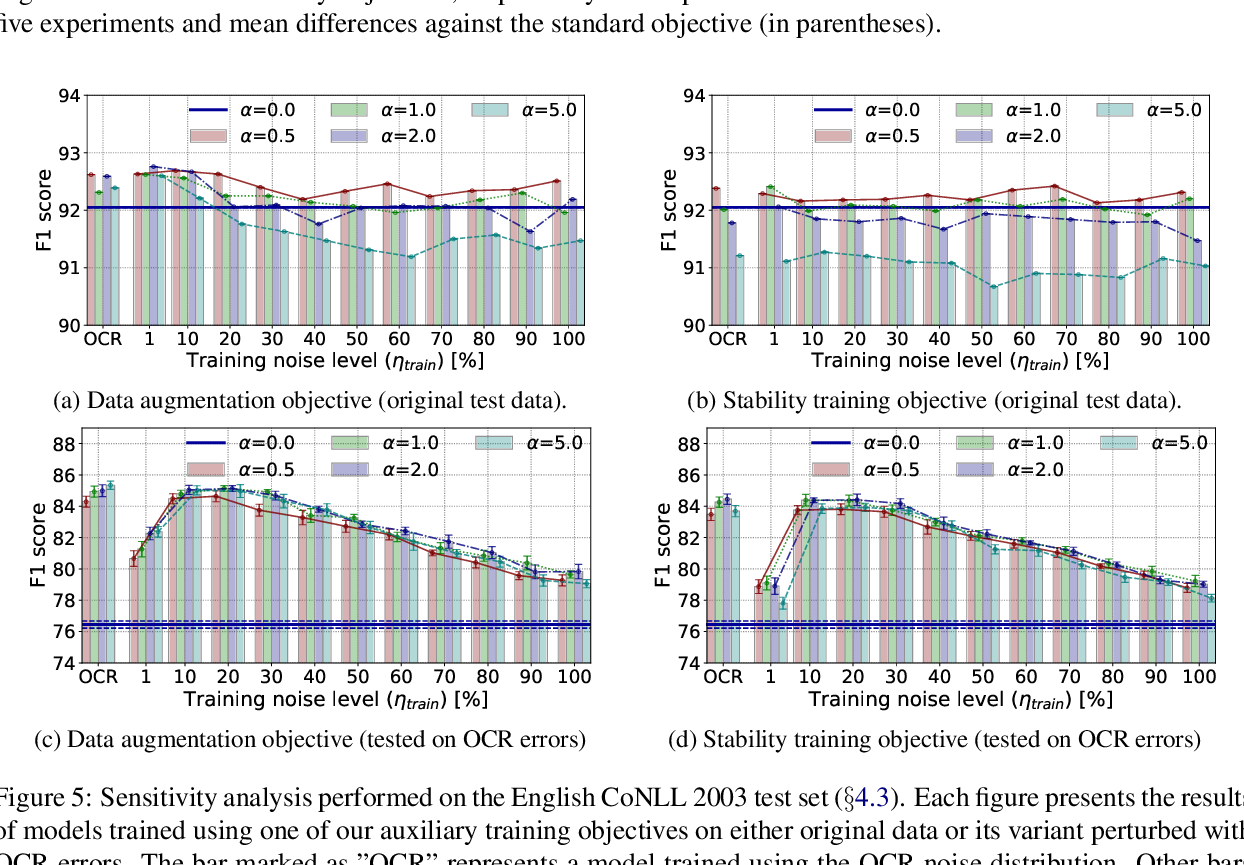
Balancing Training for Multilingual Neural Machine Translation
Xinyi Wang, Yulia Tsvetkov, Graham Neubig,
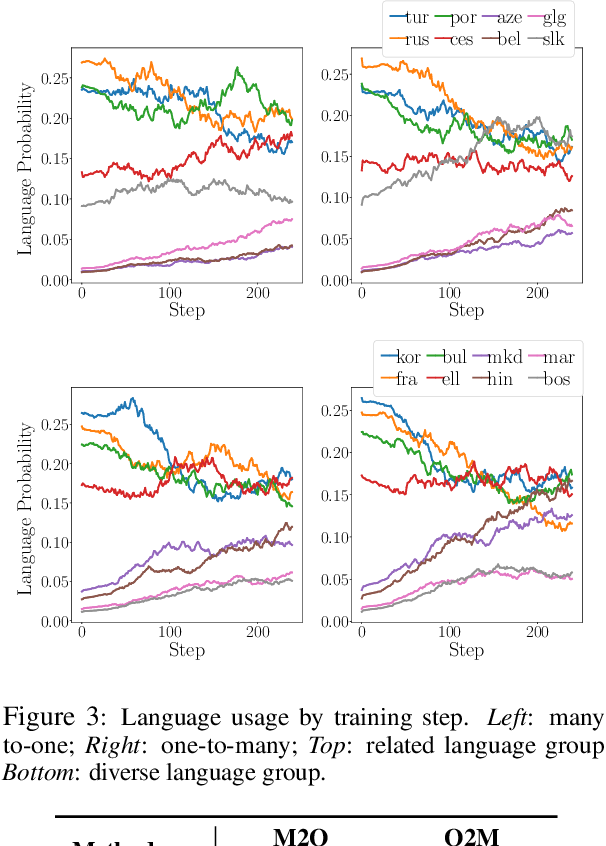