RAT-SQL: Relation-Aware Schema Encoding and Linking for Text-to-SQL Parsers
Bailin Wang, Richard Shin, Xiaodong Liu, Oleksandr Polozov, Matthew Richardson
Semantics: Sentence Level Long Paper
Session 13A: Jul 8
(12:00-13:00 GMT)
Session 14A: Jul 8
(17:00-18:00 GMT)
Abstract:
When translating natural language questions into SQL queries to answer questions from a database, contemporary semantic parsing models struggle to generalize to unseen database schemas. The generalization challenge lies in (a) encoding the database relations in an accessible way for the semantic parser, and (b) modeling alignment between database columns and their mentions in a given query. We present a unified framework, based on the relation-aware self-attention mechanism, to address schema encoding, schema linking, and feature representation within a text-to-SQL encoder. On the challenging Spider dataset this framework boosts the exact match accuracy to 57.2%, surpassing its best counterparts by 8.7% absolute improvement. Further augmented with BERT, it achieves the new state-of-the-art performance of 65.6% on the Spider leaderboard. In addition, we observe qualitative improvements in the model's understanding of schema linking and alignment. Our implementation will be open-sourced at https://github.com/Microsoft/rat-sql.
You can open the
pre-recorded video
in a separate window.
NOTE: The SlidesLive video may display a random order of the authors.
The correct author list is shown at the top of this webpage.
Similar Papers
Photon: A Robust Cross-Domain Text-to-SQL System
Jichuan Zeng, Xi Victoria Lin, Steven C.H. Hoi, Richard Socher, Caiming Xiong, Michael Lyu, Irwin King,
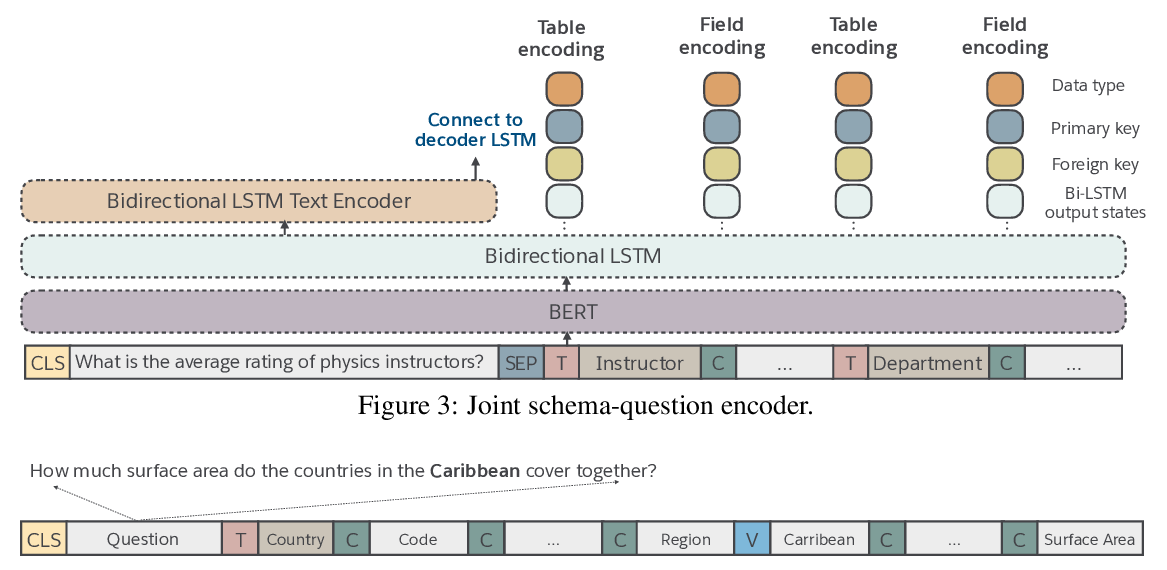
A Transformer-based Approach for Source Code Summarization
Wasi Ahmad, Saikat Chakraborty, Baishakhi Ray, Kai-Wei Chang,
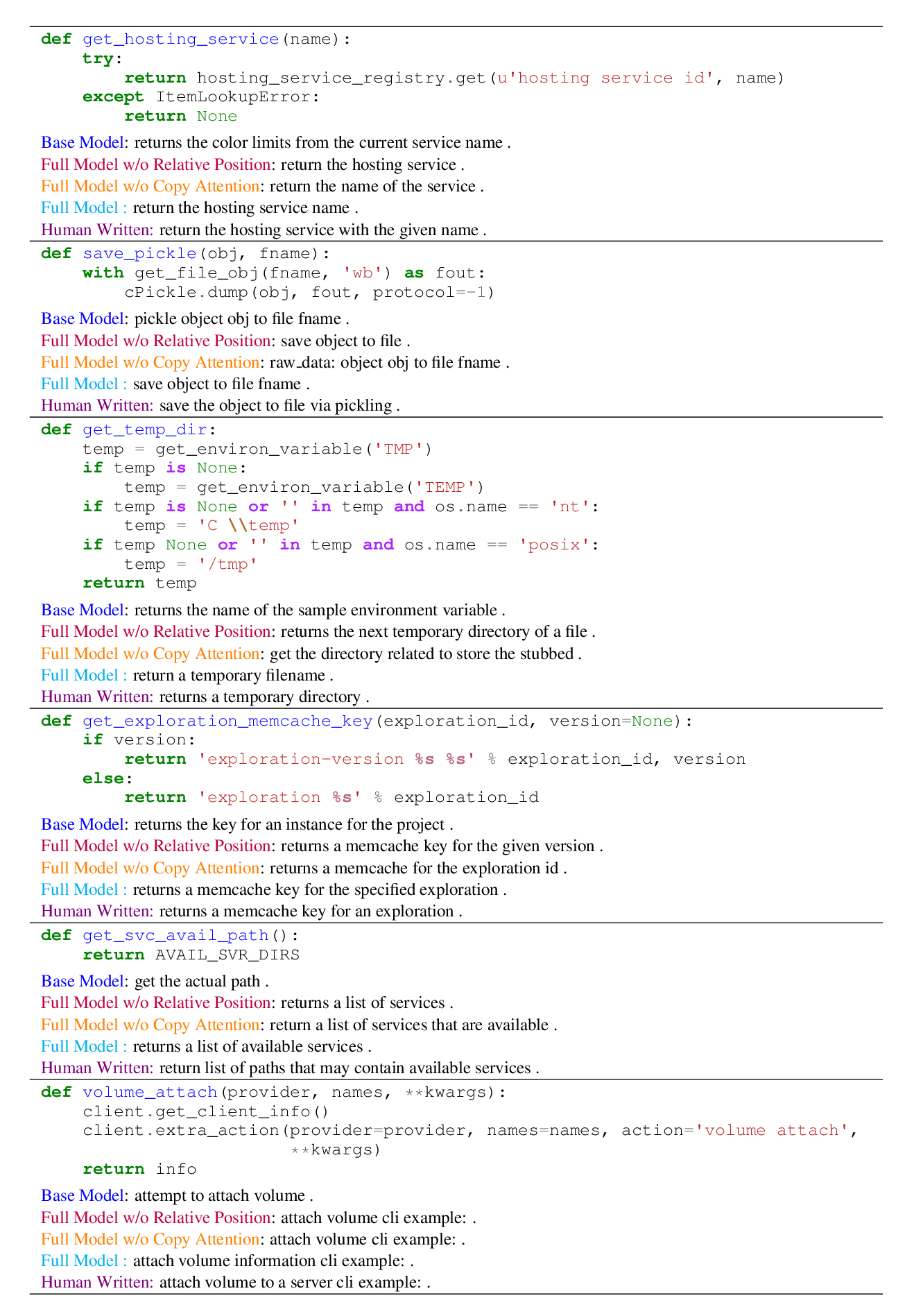
Incorporating External Knowledge through Pre-training for Natural Language to Code Generation
Frank F. Xu, Zhengbao Jiang, Pengcheng Yin, Bogdan Vasilescu, Graham Neubig,
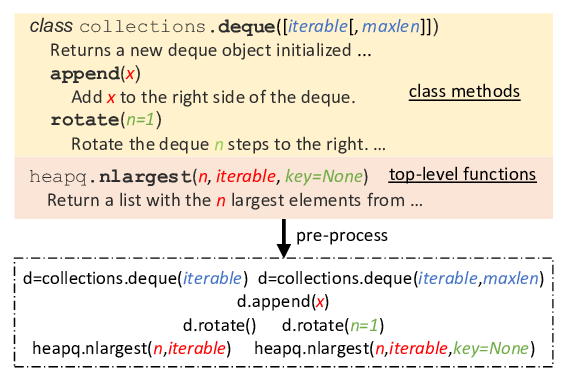