CompGuessWhat?!: A Multi-task Evaluation Framework for Grounded Language Learning
Alessandro Suglia, Ioannis Konstas, Andrea Vanzo, Emanuele Bastianelli, Desmond Elliott, Stella Frank, Oliver Lemon
Language Grounding to Vision, Robotics and Beyond Long Paper
Session 13B: Jul 8
(13:00-14:00 GMT)
Session 14A: Jul 8
(17:00-18:00 GMT)
Abstract:
Approaches to Grounded Language Learning are commonly focused on a single task-based final performance measure which may not depend on desirable properties of the learned hidden representations, such as their ability to predict object attributes or generalize to unseen situations. To remedy this, we present GroLLA, an evaluation framework for Grounded Language Learning with Attributes based on three sub-tasks: 1) Goal-oriented evaluation; 2) Object attribute prediction evaluation; and 3) Zero-shot evaluation. We also propose a new dataset CompGuessWhat?! as an instance of this framework for evaluating the quality of learned neural representations, in particular with respect to attribute grounding. To this end, we extend the original GuessWhat?! dataset by including a semantic layer on top of the perceptual one. Specifically, we enrich the VisualGenome scene graphs associated with the GuessWhat?! images with several attributes from resources such as VISA and ImSitu. We then compare several hidden state representations from current state-of-the-art approaches to Grounded Language Learning. By using diagnostic classifiers, we show that current models' learned representations are not expressive enough to encode object attributes (average F1 of 44.27). In addition, they do not learn strategies nor representations that are robust enough to perform well when novel scenes or objects are involved in gameplay (zero-shot best accuracy 50.06%).
You can open the
pre-recorded video
in a separate window.
NOTE: The SlidesLive video may display a random order of the authors.
The correct author list is shown at the top of this webpage.
Similar Papers
On the Linguistic Representational Power of Neural Machine Translation Models
Yonatan Belinkov, Nadir Durrani, Fahim Dalvi, Hassan Sajjad, James Glass,
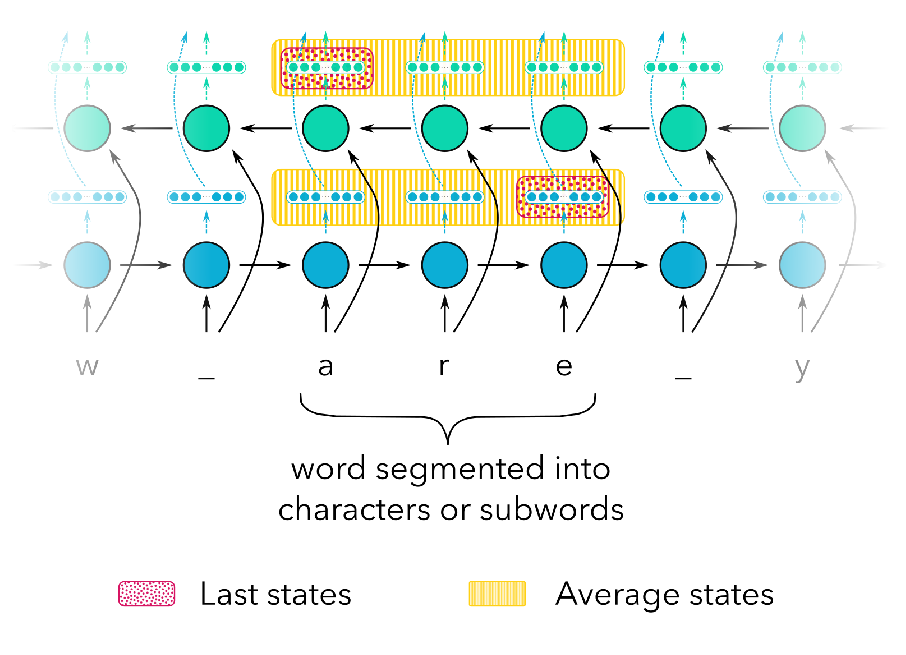
Semi-Supervised Dialogue Policy Learning via Stochastic Reward Estimation
Xinting Huang, Jianzhong Qi, Yu Sun, Rui Zhang,
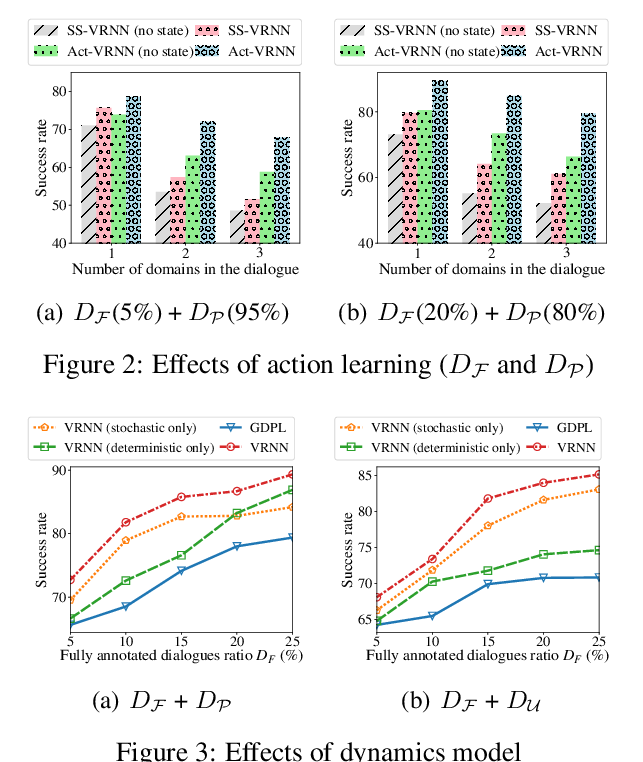
Image-Chat: Engaging Grounded Conversations
Kurt Shuster, Samuel Humeau, Antoine Bordes, Jason Weston,
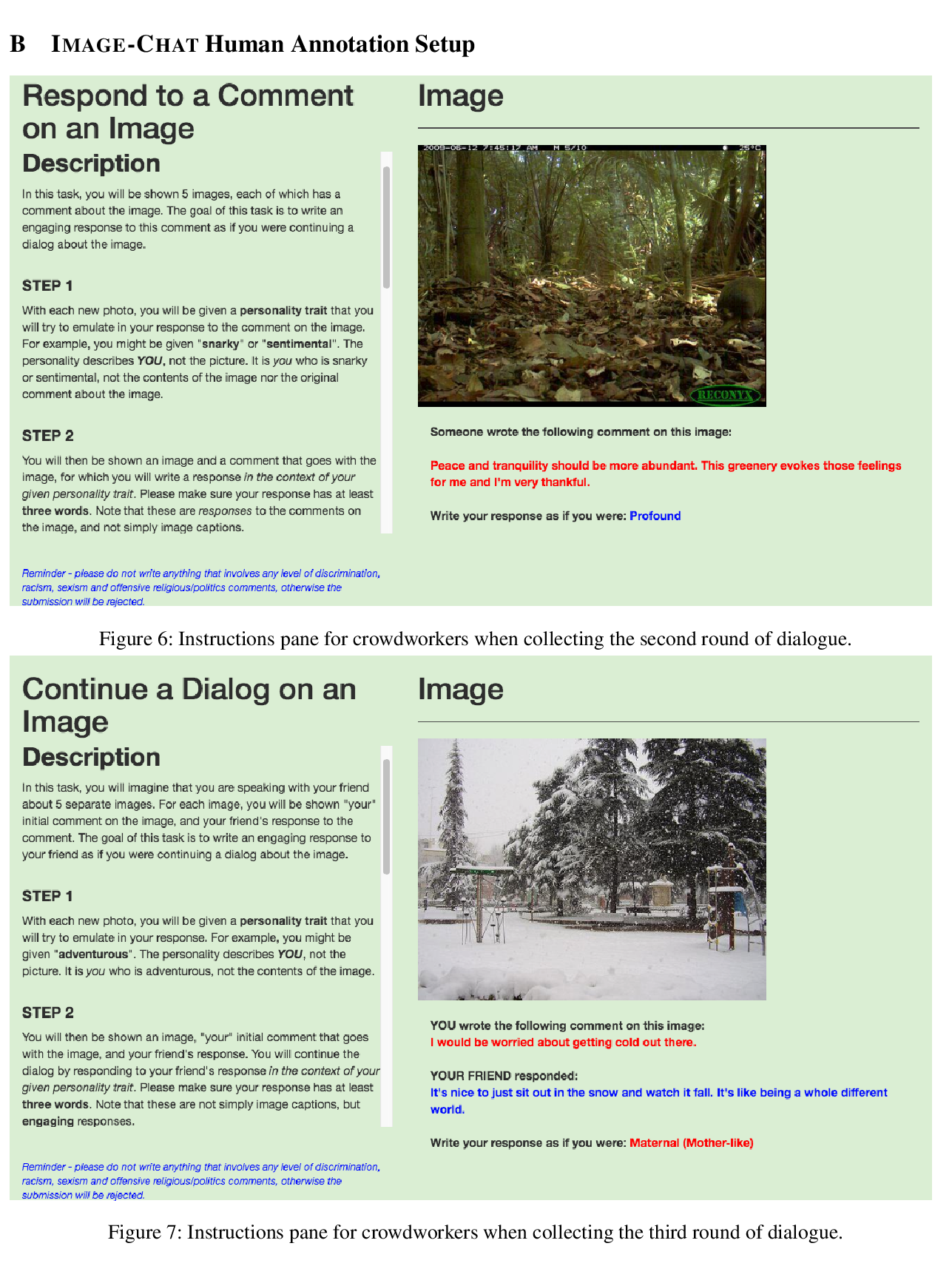