Image-Chat: Engaging Grounded Conversations
Kurt Shuster, Samuel Humeau, Antoine Bordes, Jason Weston
Dialogue and Interactive Systems Long Paper
Session 4B: Jul 6
(18:00-19:00 GMT)
Session 5B: Jul 6
(21:00-22:00 GMT)
Abstract:
To achieve the long-term goal of machines being able to engage humans in conversation, our models should captivate the interest of their speaking partners. Communication grounded in images, whereby a dialogue is conducted based on a given photo, is a setup naturally appealing to humans (Hu et al., 2014). In this work we study large-scale architectures and datasets for this goal. We test a set of neural architectures using state-of-the-art image and text representations, considering various ways to fuse the components. To test such models, we collect a dataset of grounded human-human conversations, where speakers are asked to play roles given a provided emotional mood or style, as the use of such traits is also a key factor in engagingness (Guo et al., 2019). Our dataset, Image-Chat, consists of 202k dialogues over 202k images using 215 possible style traits. Automatic metrics and human evaluations of engagingness show the efficacy of our approach; in particular, we obtain state-of-the-art performance on the existing IGC task, and our best performing model is almost on par with humans on the Image-Chat test set (preferred 47.7% of the time).
You can open the
pre-recorded video
in a separate window.
NOTE: The SlidesLive video may display a random order of the authors.
The correct author list is shown at the top of this webpage.
Similar Papers
You Impress Me: Dialogue Generation via Mutual Persona Perception
Qian Liu, Yihong Chen, Bei Chen, Jian-Guang Lou, Zixuan Chen, Bin Zhou, Dongmei Zhang,
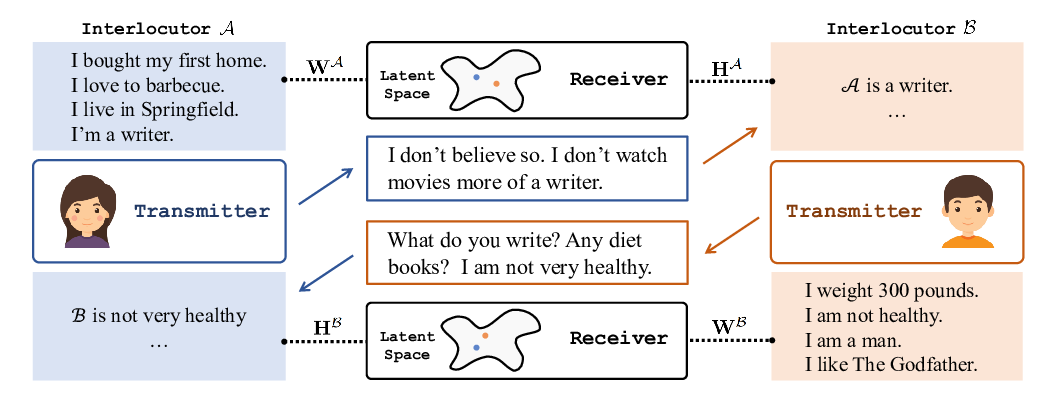
Improving Image Captioning Evaluation by Considering Inter References Variance
Yanzhi Yi, Hangyu Deng, Jinglu Hu,
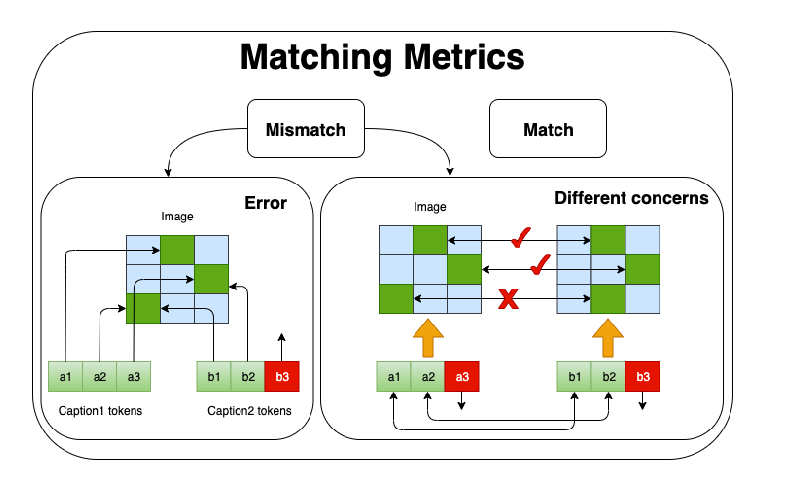
Automatic Detection of Generated Text is Easiest when Humans are Fooled
Daphne Ippolito, Daniel Duckworth, Chris Callison-Burch, Douglas Eck,
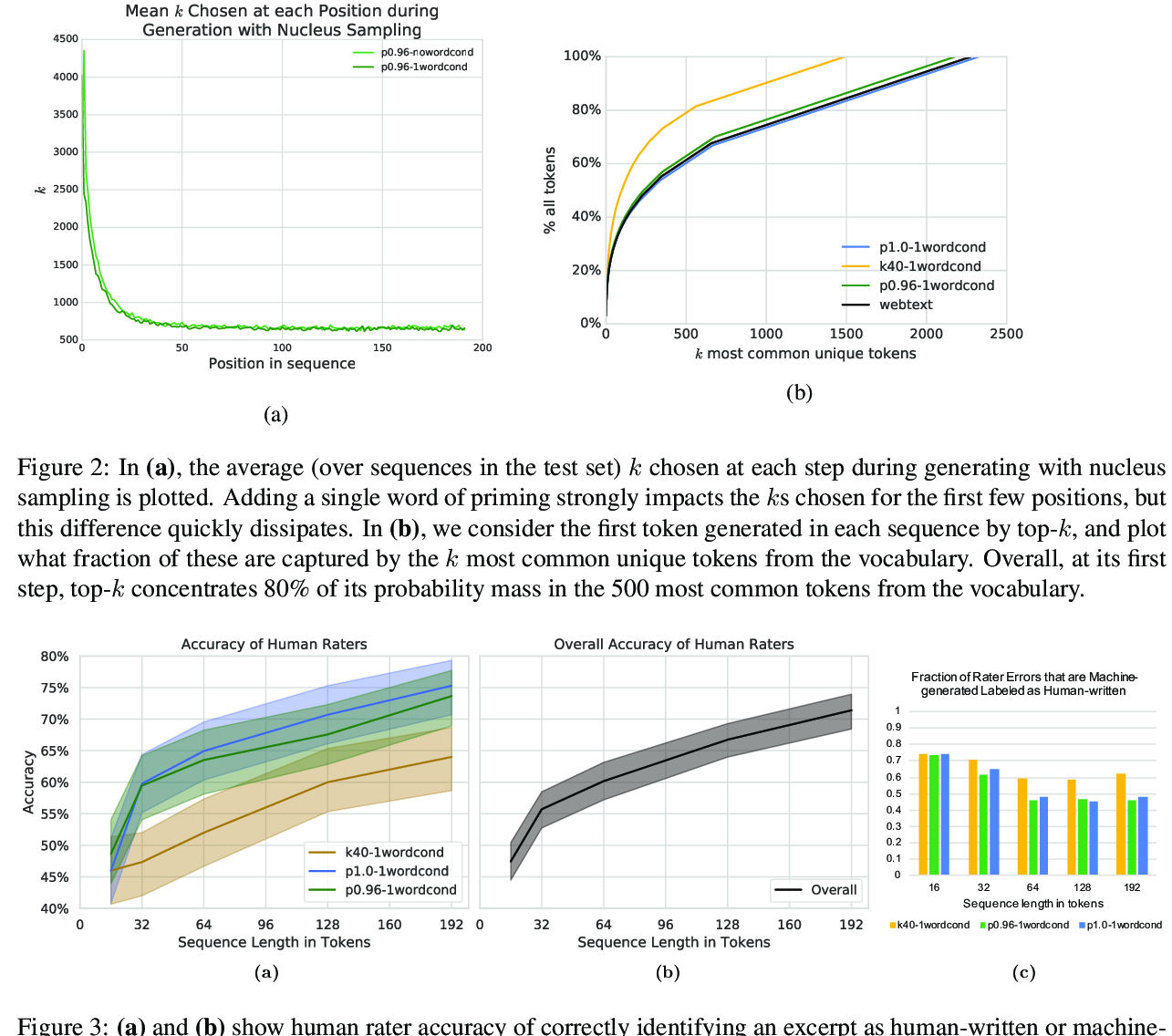