Don’t Stop Pretraining: Adapt Language Models to Domains and Tasks
Suchin Gururangan, Ana Marasović, Swabha Swayamdipta, Kyle Lo, Iz Beltagy, Doug Downey, Noah A. Smith
Semantics: Sentence Level Long Paper
Session 14A: Jul 8
(17:00-18:00 GMT)
Session 15B: Jul 8
(21:00-22:00 GMT)
Abstract:
Language models pretrained on text from a wide variety of sources form the foundation of today's NLP. In light of the success of these broad-coverage models, we investigate whether it is still helpful to tailor a pretrained model to the domain of a target task. We present a study across four domains (biomedical and computer science publications, news, and reviews) and eight classification tasks, showing that a second phase of pretraining in-domain (domain-adaptive pretraining) leads to performance gains, under both high- and low-resource settings. Moreover, adapting to the task's unlabeled data (task-adaptive pretraining) improves performance even after domain-adaptive pretraining. Finally, we show that adapting to a task corpus augmented using simple data selection strategies is an effective alternative, especially when resources for domain-adaptive pretraining might be unavailable. Overall, we consistently find that multi-phase adaptive pretraining offers large gains in task performance.
You can open the
pre-recorded video
in a separate window.
NOTE: The SlidesLive video may display a random order of the authors.
The correct author list is shown at the top of this webpage.
Similar Papers
Intermediate-Task Transfer Learning with Pretrained Language Models: When and Why Does It Work?
Yada Pruksachatkun, Jason Phang, Haokun Liu, Phu Mon Htut, Xiaoyi Zhang, Richard Yuanzhe Pang, Clara Vania, Katharina Kann, Samuel R. Bowman,
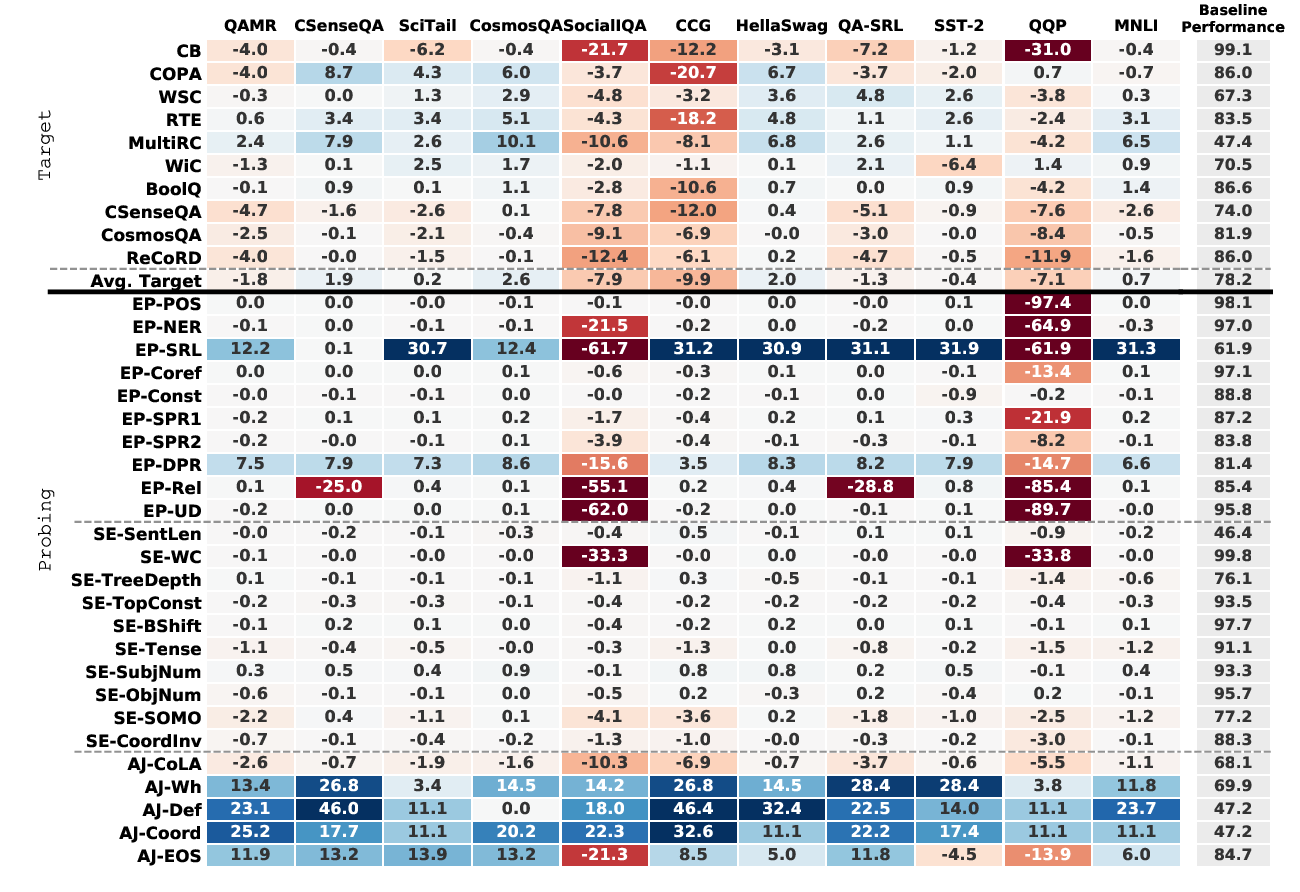
BART: Denoising Sequence-to-Sequence Pre-training for Natural Language Generation, Translation, and Comprehension
Mike Lewis, Yinhan Liu, Naman Goyal, Marjan Ghazvininejad, Abdelrahman Mohamed, Omer Levy, Veselin Stoyanov, Luke Zettlemoyer,
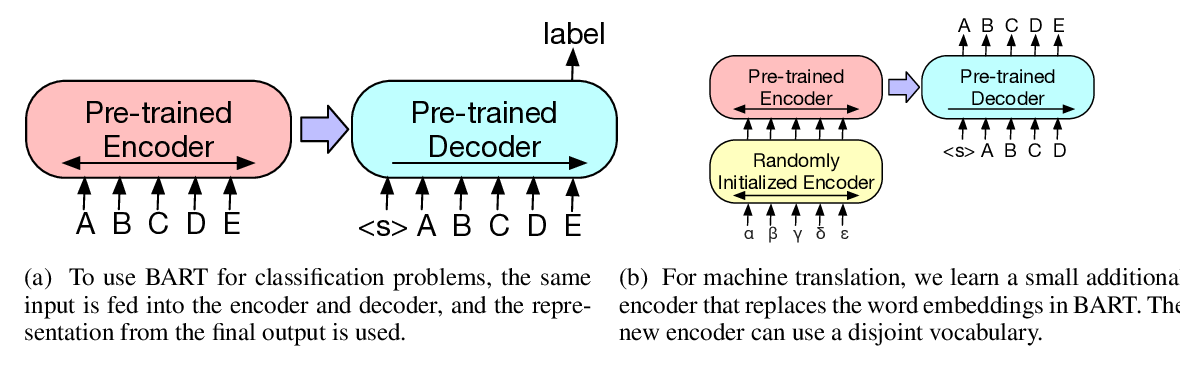
Pretrained Transformers Improve Out-of-Distribution Robustness
Dan Hendrycks, Xiaoyuan Liu, Eric Wallace, Adam Dziedzic, Rishabh Krishnan, Dawn Song,
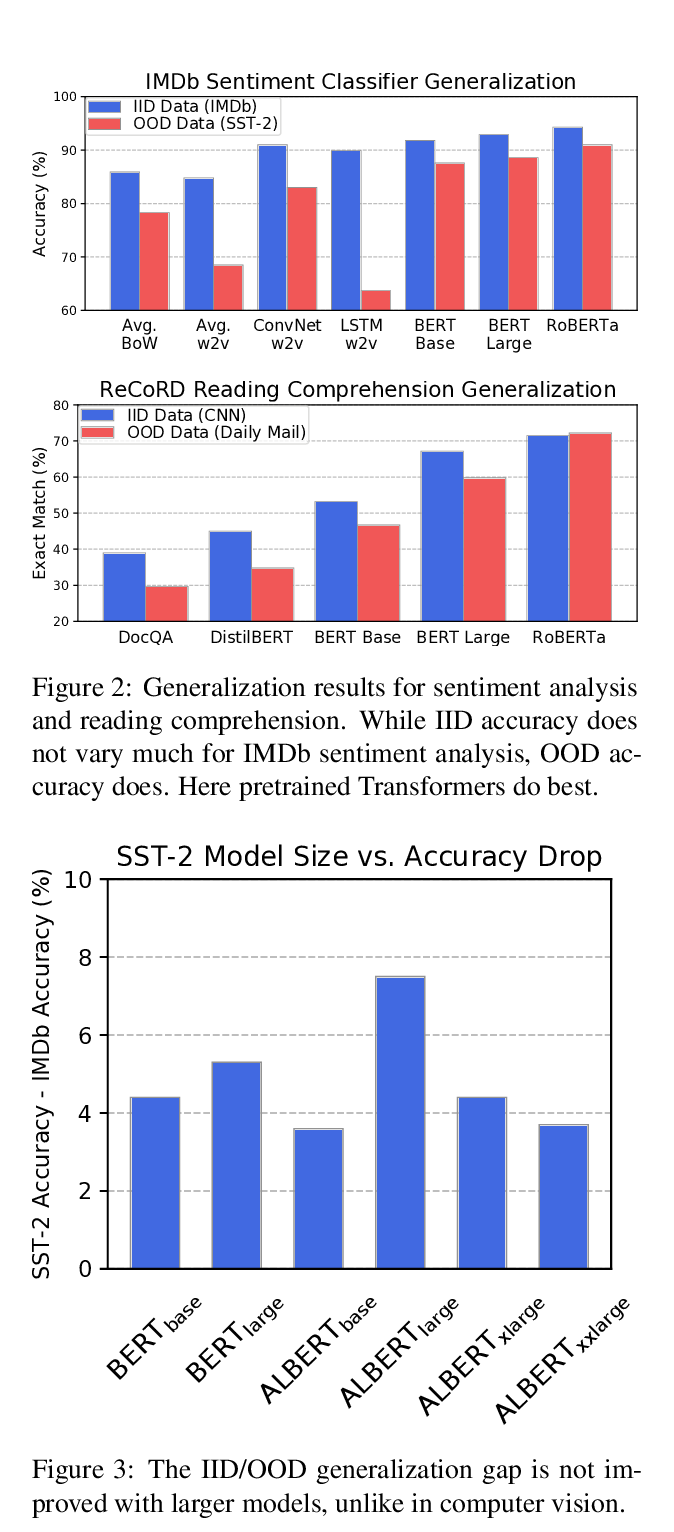
Adversarial and Domain-Aware BERT for Cross-Domain Sentiment Analysis
Chunning Du, Haifeng Sun, Jingyu Wang, Qi Qi, Jianxin Liao,
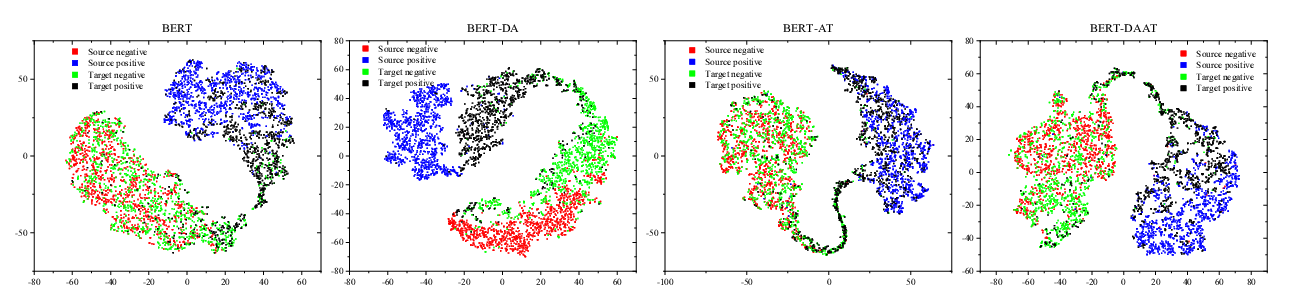