Adversarial and Domain-Aware BERT for Cross-Domain Sentiment Analysis
Chunning Du, Haifeng Sun, Jingyu Wang, Qi Qi, Jianxin Liao
Sentiment Analysis, Stylistic Analysis, and Argument Mining Long Paper
Session 7A: Jul 7
(08:00-09:00 GMT)
Session 8A: Jul 7
(12:00-13:00 GMT)
Abstract:
Cross-domain sentiment classification aims to address the lack of massive amounts of labeled data. It demands to predict sentiment polarity on a target domain utilizing a classifier learned from a source domain. In this paper, we investigate how to efficiently apply the pre-training language model BERT on the unsupervised domain adaptation. Due to the pre-training task and corpus, BERT is task-agnostic, which lacks domain awareness and can not distinguish the characteristic of source and target domain when transferring knowledge. To tackle these problems, we design a post-training procedure, which contains the target domain masked language model task and a novel domain-distinguish pre-training task. The post-training procedure will encourage BERT to be domain-aware and distill the domain-specific features in a self-supervised way. Based on this, we could then conduct the adversarial training to derive the enhanced domain-invariant features. Extensive experiments on Amazon dataset show that our model outperforms state-of-the-art methods by a large margin. The ablation study demonstrates that the remarkable improvement is not only from BERT but also from our method.
You can open the
pre-recorded video
in a separate window.
NOTE: The SlidesLive video may display a random order of the authors.
The correct author list is shown at the top of this webpage.
Similar Papers
Dynamic Fusion Network for Multi-Domain End-to-end Task-Oriented Dialog
Libo Qin, Xiao Xu, Wanxiang Che, Yue Zhang, Ting Liu,
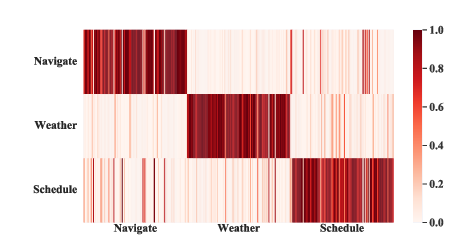
Coupling Distant Annotation and Adversarial Training for Cross-Domain Chinese Word Segmentation
Ning Ding, Dingkun Long, Guangwei Xu, Muhua Zhu, Pengjun Xie, Xiaobin Wang, Haitao Zheng,
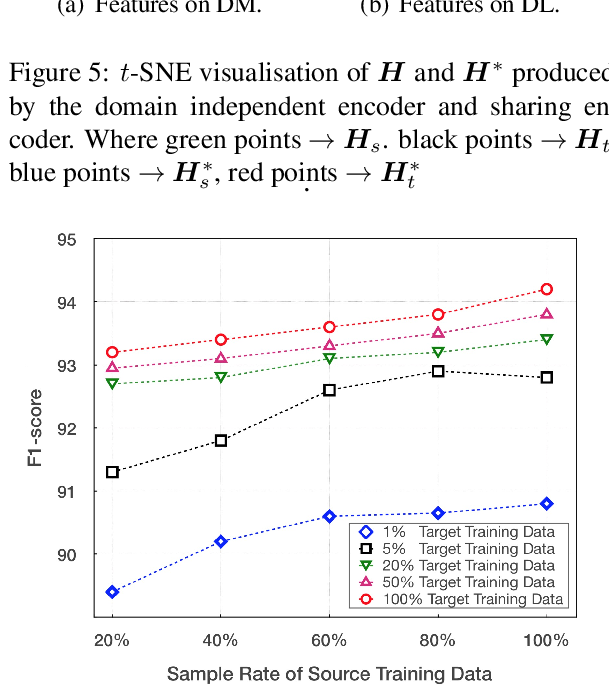
Towards Open Domain Event Trigger Identification using Adversarial Domain Adaptation
Aakanksha Naik, Carolyn Rose,
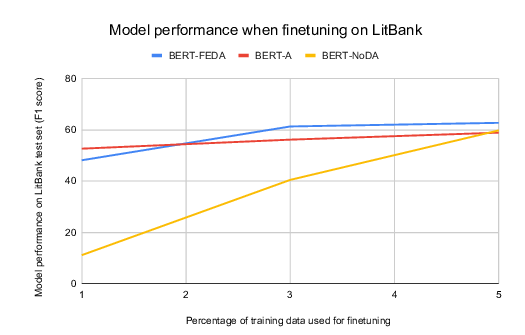
Span Selection Pre-training for Question Answering
Michael Glass, Alfio Gliozzo, Rishav Chakravarti, Anthony Ferritto, Lin Pan, G P Shrivatsa Bhargav, Dinesh Garg, Avi Sil,
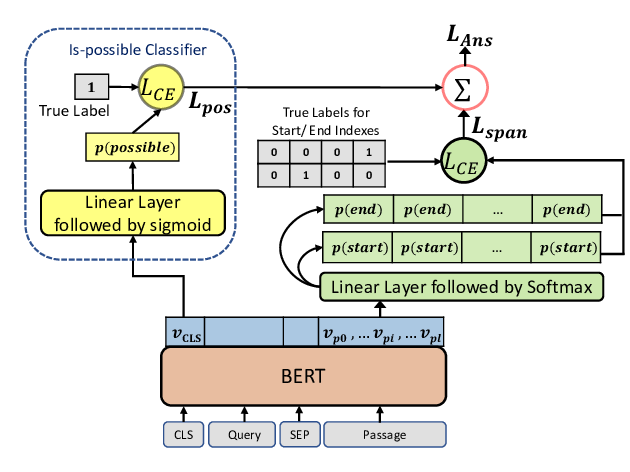